Uber's AI went through Montezuma's Revenge better than a man
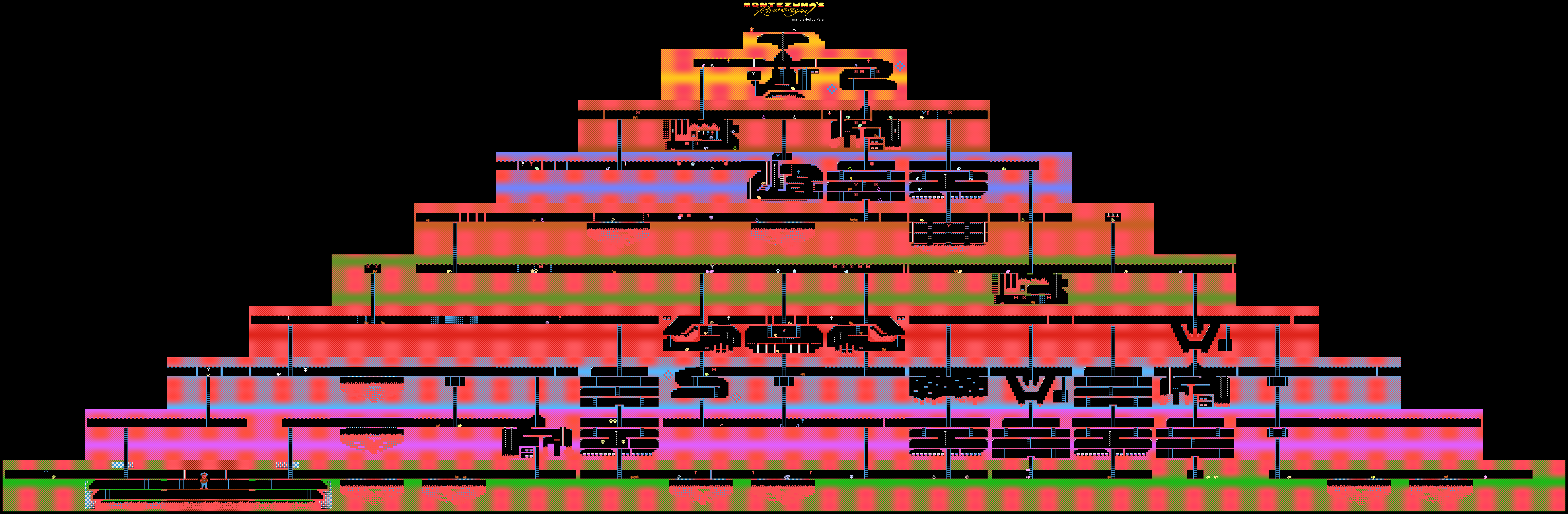
About the game Montezuma's Revenge on Habré was written not so much. This is a complex classic game that was previously very popular, but now it is played either by those with whom it causes nostalgic feelings, or by researchers who develop AI.
This summer, it was reported that DeepMind was able to teach its AI how to play games for Atari, including Montezuma's Revenge. Using the example of the same game, the developers of OpenAI taught their development. Now a similar project has taken the company Uber.
The developers have announced the passage of the game by their neural network, with a maximum score of 2 million. However, on average, the system earned no more than 400,000 for each attempt. As for the passage, the computer got to level 159.
In addition, Go-Explore learned to go through Pitfall, and with excellent results, which is superior to the average gamer, not to mention other AI agents. The number of points scored by Go-Explore in this game is 21,000.
The difference between Go-Explore and "colleagues" is that neural networks do not need to demonstrate passing different levels for learning. The system learns everything by itself in the course of the game, showing results that are much higher than those demonstrated by neural networks that require visual training. According to the developers of Go-Explore, the technology is significantly different from all others, and its capabilities allow the use of a neural network in a number of areas, including robotics.
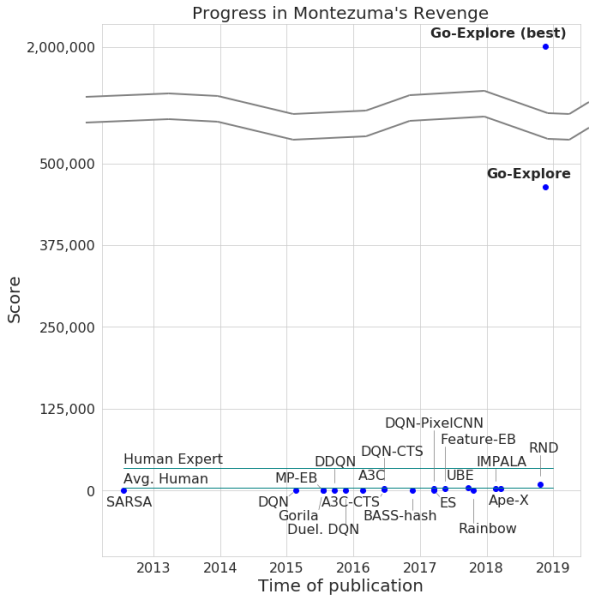
Most algorithms find it difficult to cope with Montezuma's Revenge because the game does not have too obvious feedback. For example, a neural network that is “sharpened” to receive a reward in the process of passing a level will rather fight the enemy than jump onto a ladder that leads to the exit and allows you to go forward faster. Other AI systems prefer to get a reward here and now, rather than go ahead in "hope" for more.
One of the decisions of Uber engineers is to add bonuses for studying the game world, this can be called internal AI motivation. But even AI elements with added intrinsic motivation don't do too well with Montezuma's Revenge and Pitfall. The problem is that the AI “forgets” about promising locations after their passage. As a result, the AI agent gets stuck at a level where everything seems to be investigated.
An example is an AI agent who needs to study two mazes - the east and the west. He begins to pass one of them, but then suddenly decides that it would be possible to go through the second one. The first remains studied at 50%, the second - at 100%. And in the first labyrinth the agent does not return - simply because he “forgot” that he did not pass to the end. And since the passage between the eastern and western labyrinths has already been studied, the AI has no motivation to return.
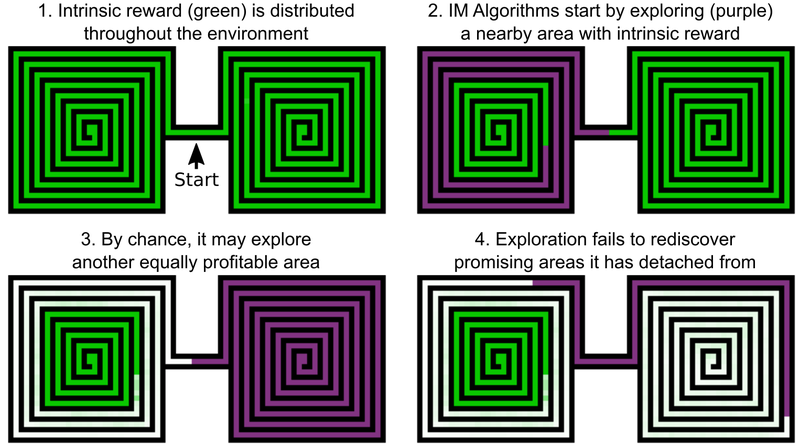
The solution to this problem, according to the developers of Uber, includes two stages: research and amplification. As for the first part, here the AI creates an archive of various game states - the cells (cells) - and the different trajectories that lead to them. AI chooses the opportunity to get the maximum points when it finds the optimal trajectory.
Cells are simplified game frames - 11 by 8 images in grayscale with 8 pixel intensity, with frames that differ sufficiently - in order not to hinder the further passage of the game.
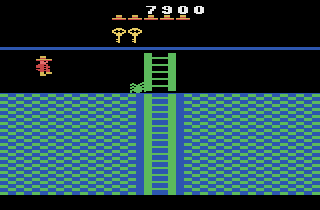
As a result, the AI remembers promising locations and returns to them after examining other parts of the game world. The “desire” to explore the game world and promising locations at Go-Explore is stronger than the desire to get an award here and now. Go-Explore also uses information about the cells in which the AI agent is trained. For Montezuma's Revenge, these are pixel data like their X and Y coordinates, the current room, and the number of keys found.
The amplification stage works as a protection against "noise". If AI solutions are unstable to "noise", then AI enhances them with the help of a multi-level neural network that works like the neurons of the human brain.

In the tests, Go-Explore shows itself very well - on average, the AI studies 37 rooms and solves 65% of the first-level puzzles. This is much better than previous attempts to conquer the game - then the AI, on average, studied 22 first-level rooms.

When adding gain to an existing AI algorithm, it began on average to successfully pass 29 levels (not rooms) with an average score of 469,209.
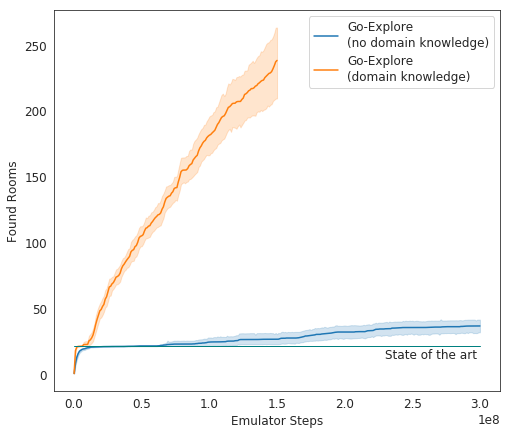
The final incarnation of AI from Uber began to go through the game much better than other AI agents, and better than people. Now developers are improving their system so that it shows even more impressive results.