Ethical Issues of Artificial Intelligence
The author of the article is Alexey Malanov, an expert in the development of anti-virus technologies at Kaspersky Lab.
Artificial intelligence breaks into our lives. In the future, everything will probably be cool, but so far some questions arise, and more and more often these questions touch upon aspects of morality and ethics. Is it possible to mock thinking AI? When will it be invented? What prevents us from already writing the laws of robotics, putting morality in them? What surprises does machine learning present us to now? Is it possible to deceive machine learning, and how difficult is it?
There are two different things: Strong and Weak AI.
Strong AI (true, general, real) is a hypothetical machine that can think and be aware of itself, solve not only highly specialized tasks, but also learn something new.
Weak AI (narrow, superficial) - these are already existing programs for solving well-defined tasks, such as image recognition, driving, playing Go, and so on. In order not to be confused and not mislead anyone, we prefer to call Weak AI "machine learning (machine learning).
About Strong AI it is not yet known whether it will ever be invented at all. On the one hand, so far technologies have evolved with acceleration, and if it goes on like this, then there are five years left.

On the other hand, few processes in nature actually take place exponentially. More often, we still see the logistic curve.

While we are somewhere on the left of the graph, it seems to us that this is an exhibitor. For example, more recently, Earth’s population has grown with such acceleration. But at some point there is a "saturation", and growth slows down.
When experts are interviewed , it turns out that on average, to wait another 45 years.

What is curious is that North American scientists believe that AI will surpass a person in 74 years, and Asian ones - in just 30. Perhaps in Asia they know something like that ...
The same scientists predicted that the car would translate better than a man by 2024, write school essays by 2026, drive trucks by 2027, play Go, also by 2027. With Guo, the bobble has already come out, because this moment came in 2017, just 2 years after the forecast.
Well, in general, forecasts for 40+ years ahead are a thankless task. It means "sometime." For example, the cost-effective energy of thermonuclear fusion is also predicted in 40 years. The same forecast was given 50 years ago, when it was just begun to be studied.

Although a strong AI will wait for a long time, but we know for sure that there will be enough ethical problems. The first class of problems - we can offend the AI. For example:
Now no one will be indignant if you offend your voice assistant, but if you treat the dog badly, you will be convicted. And this is not because it is made of flesh and blood, but because it feels and experiences a bad relationship, as it will with Strong AI.
The second class of ethical problems - AI can offend us. Hundreds of such examples can be found in films and books. How to explain the AI, what do we want from it? People for AI are like ants for workers building a dam: for the sake of a great goal, you can crush a couple.
Science fiction is playing a trick on us. We used to think that Skynet and Terminators are not there yet, and they will be soon, but for now you can relax. AI in films is often malicious, and we hope that this will not happen in life: after all, we were warned, and we are not as stupid as film characters. At the same time, in our thoughts about the future, we forget to think well about the present.
Machine learning allows you to solve a practical problem without explicit programming, but by learning from precedents. You can read more in the article “ In simple words: how machine learning works .”
Since we teach the machine to solve a specific problem, the resulting mathematical model (the so-called algorithm) cannot suddenly want to enslave / save humanity. Do normally - it will be normal. What can go wrong?

First, the problem itself may not be ethical enough. For example, if we teach drones to kill people with machine learning.

https://www.youtube.com/watch?v=TlO2gcs1YvM
Just recently, a small scandal broke out about this. Google is developing software used for the Project Maven military drone pilot project. Presumably, in the future this could lead to the creation of fully autonomous weapons.

Source
So, at least 12 employees of Google quit in protest, another 4000 signed a petition asking them to withdraw from the contract with the military. Over 1000 eminent scientists in the field of AI, ethics and information technology have written an open letter asking Google to stop working on the project and support an international treaty to ban autonomous weapons.
But even if the authors of the machine learning algorithm and do not want to kill people and bring harm, they, nevertheless, often still want to benefit. In other words, not all algorithms work for the good of society, many work for the benefit of the creators. This can often be observed in the field of medicine - it is more important not to cure, but to recommend more treatment.
In general, if machine learning advises something paid - with high probability the algorithm is “greedy”.
Well, and sometimes the society itself is not interested in the resulting algorithm being a model of morality. For example, there is a compromise between the speed of traffic and mortality on the roads. We could greatly reduce mortality if we limited the speed to 20 km / h, but then life in big cities would be difficult.
Imagine, we are asking the algorithm to impose a country's budget in order to “maximize GDP / labor productivity / life expectancy.” In the formulation of this problem there are no ethical restrictions and goals. Why allocate money for orphanages / hospices / environmental protection, because it does not increase GDP (at least, directly)? And it’s good if we only assign the budget to the algorithm, otherwise, in a broader formulation of the problem, it will be released that it is “more profitable” to kill the inoperative population right away in order to increase labor productivity.
It turns out that ethical issues should be among the goals of the system initially.
There is one problem with ethics - it is difficult to formalize it. Different countries have different ethics. It changes with time. For example, on issues such as LGBT rights and interracial / inter-caste marriages, opinions may change significantly over the decades. Ethics may depend on the political climate.

For example, in China, controlling the movement of citizens with the help of surveillance cameras and face recognition is considered the norm. In other countries, the attitude to this issue may be different and depend on the situation.
Imagine a machine learning based system that advises you which movie to watch. Based on your ratings for other films, and by comparing your tastes with the tastes of other users, the system can quite reliably recommend a film that you’ll like very much.
But at the same time, the system will eventually change your tastes and make them more narrow. Without a system, you would occasionally watch both bad films and films of unusual genres. And so that no movie - to the point. As a result, we cease to be "film experts", and become only consumers of what they give. Another interesting fact is that we do not even notice how the algorithms manipulate us.
If you say that the impact of algorithms on people is even good, here is another example. China is preparing to launch the Social Rating System - a system for evaluating individual citizens or organizations for various parameters, the values of which are obtained using mass monitoring tools and using big data analysis technology.
If a person buys diapers - this is good, the rating grows. If you spend money on video games - this is bad, the rating drops. If communicates with a person with a low rating, it also falls.
As a result, it turns out that thanks to the System, citizens consciously or subconsciously begin to behave differently. Communicate less with unreliable citizens, buy more diapers, etc.
Besides the fact that we sometimes do not know what we want from the algorithm, there is also a whole bunch of technical limitations.
The algorithm absorbs the imperfections of the surrounding world.
If data from a company with racist politicians is used as a training sample for an algorithm for hiring employees, the algorithm will also be racist.
Microsoft once taught a chat bot to chat on Twitter. It had to be turned off in less than a day, because the bot quickly mastered curses and racist remarks.

In addition, the algorithm during training can not take into account some non-formalizable parameters. For example, when calculating a recommendation to a defendant - whether or not to admit guilt on the basis of collected evidence, the algorithm finds it difficult to take into account what impression such a confession will make on a judge, because the impression and emotions are not recorded anywhere.
False correlation - this is when it seems that the more firefighters in the city, the more often fires. Or when it is obvious that the fewer pirates on Earth, the warmer the climate on the planet.

So - people suspect that pirates and the climate are not directly related, and firefighters are not so simple, and Matmodel of machine learning simply learns and generalizes.
Famous example. The program, which arranged for patients to take turns on the urgency of providing care, concluded that asthmatics with pneumonia need less help than people with pneumonia without asthma. The program looked at the statistics and concluded that asthmatics do not die - why do they need priority? But they do not actually die because such patients immediately receive the best care in medical institutions due to the very high risk.
Worse than false correlations are only feedback loops. The California crime prevention program proposed sending more police officers to the black neighborhoods based on the crime rate (number of recorded crimes). And the more police cars in the field of visibility, the more often residents report crimes (just have someone to report). As a result, crime is only increasing - it means that more police officers must be sent, etc.
In other words, if racial discrimination is a factor of arrest, then feedback loops can reinforce and perpetuate racial discrimination in police activities.
In 2016, the Big Data Working Group under the Obama Administration released a report warning of “possible coding discrimination when making automated decisions” and postulating the “principle of equal opportunities”.
But it is easy to say something, but what to do?
First, the machine learning model is hard to test and fix. For example, the Google Photo app recognized black-skinned people like gorillas. And how to be? If we read the usual programs in steps and learned how to test them, then in the case of machine learning, everything depends on the size of the control sample, and it cannot be infinite. For three years, Google could not think of anything better.except to turn off the recognition of gorillas, chimpanzees and monkeys at all, to prevent the error from recurring.
Secondly, it is difficult for us to understand and explain machine learning solutions. For example, the neural network somehow placed weights within itself so that the correct answers were obtained. And why do they turn out exactly so and what to do to change the answer?
A 2015 survey found that women are much less likely than men to see high-paying job ads being shown by Google AdSense. Amazon’s same-day delivery service was regularly unavailable in black neighborhoods. In both cases, company representatives found it difficult to explain such algorithm solutions.
It turns out that there is no one to blame, it remains to make laws and postulate the "ethical laws of robotics." Germany just recently, in May 2018, issued such a set of rules about unmanned vehicles. Among other things, it recorded there:
But what is especially important in our context:
Automatic driving systems become an ethical imperative if systems cause less accidents than human drivers.
Obviously, we will rely more and more on machine learning - simply because it will generally cope better than people.
And here we come to no less misfortune than the bias of the algorithms - they can be manipulated.
ML poisoning means that if someone takes part in the training of the model, then he can influence the decisions made by the model.
For example, in a computer virus analysis laboratory, a matmodel processes on average a million new samples (clean and malicious files) every day.
The threat landscape is constantly changing, so changes in the model in the form of anti-virus database updates are delivered to anti-virus products on the user side.
So, an attacker can constantly generate malicious files, very similar to some kind of clean, and send them to the laboratory. The border between clean and malicious files will gradually fade, the model will “degrade”. And in the end, the model can recognize the original clean file as malware - a false positive will result.
And vice versa, if you “spam” a self-learning spam filter with a ton of clean generated emails, you will eventually be able to create spam that passes through the filter.
Therefore, in Kaspersky Lab, a multi-layered approach to protection , we do not rely solely on machine learning.
Another example is fictional. Specially generated faces can be added to the facial recognition system to end up confusing you with someone else. Do not think that this is impossible, take a look at the picture from the next section.

Poisoning is an effect on the learning process. But it is not necessary to participate in training in order to get the benefit - you can deceive a ready-made model if you know how it works.

Wearing specially painted glasses, the researchers presented themselves as other people - celebrities.
This example has not yet been encountered by individuals in the “wild” - precisely because no one has yet entrusted the car to make important decisions based on facial recognition. Without control from the person will be exactly as in the picture.
Even where, seemingly, there is nothing difficult, the car is easy to deceive in a way unknown to the uninitiated.
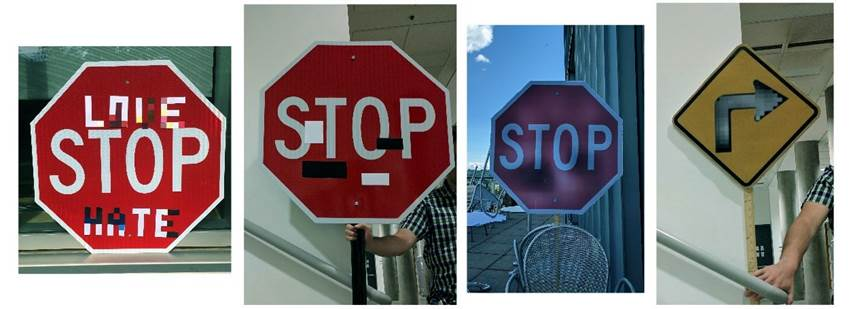
The first three characters are recognized as “Speed limit 45”, and the last one as a STOP character.
Moreover, in order for the Matmodel of machine learning to recognize surrender, it is not necessary to make significant changes, the minimum invisible edits to a person are sufficient .
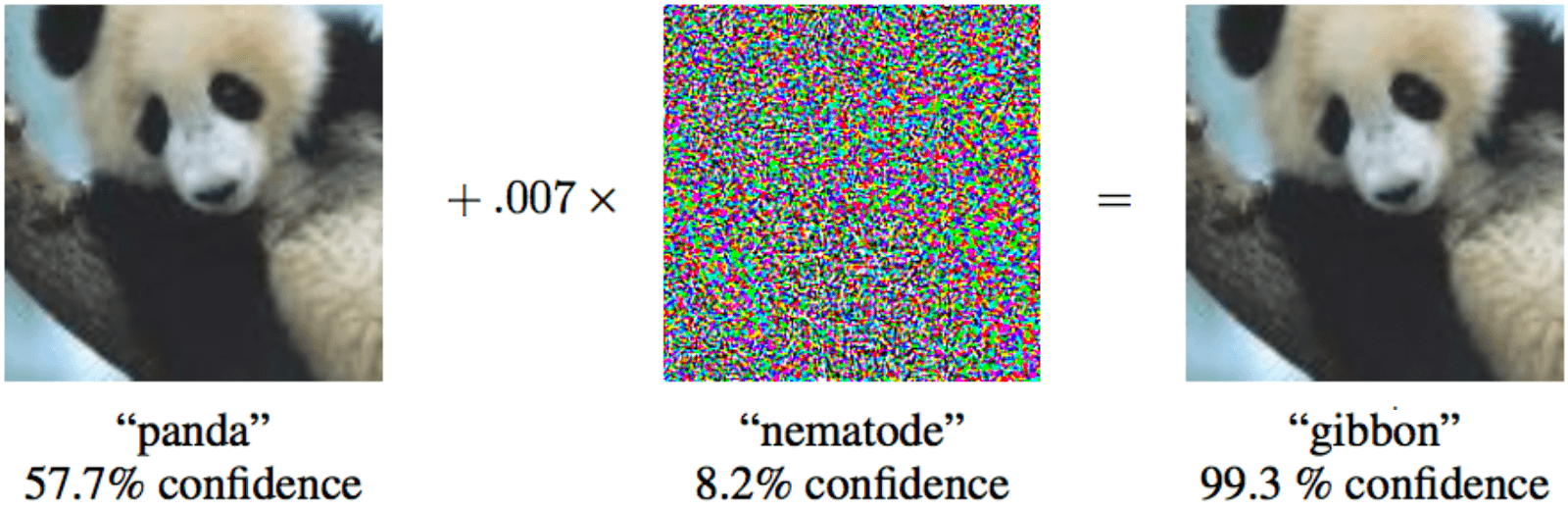
If you add the minimum special noise to the panda on the left, then machine learning will be sure that it is a gibbon.
As long as a person is smarter than most algorithms, he can deceive them. Imagine that in the near future machine learning will analyze X-rays of suitcases at the airport and look for weapons. A clever terrorist can put a special shape next to the pistol and thereby "neutralize" the pistol.
Similarly, it will be possible to “hack” the Chinese Social Rating System and become the most respected person in China.
Let's summarize what we managed to discuss.


And all this is the near future.
Artificial intelligence breaks into our lives. In the future, everything will probably be cool, but so far some questions arise, and more and more often these questions touch upon aspects of morality and ethics. Is it possible to mock thinking AI? When will it be invented? What prevents us from already writing the laws of robotics, putting morality in them? What surprises does machine learning present us to now? Is it possible to deceive machine learning, and how difficult is it?
Strong and Weak AI - different things
There are two different things: Strong and Weak AI.
Strong AI (true, general, real) is a hypothetical machine that can think and be aware of itself, solve not only highly specialized tasks, but also learn something new.
Weak AI (narrow, superficial) - these are already existing programs for solving well-defined tasks, such as image recognition, driving, playing Go, and so on. In order not to be confused and not mislead anyone, we prefer to call Weak AI "machine learning (machine learning).
A strong AI will not be soon
About Strong AI it is not yet known whether it will ever be invented at all. On the one hand, so far technologies have evolved with acceleration, and if it goes on like this, then there are five years left.

On the other hand, few processes in nature actually take place exponentially. More often, we still see the logistic curve.

While we are somewhere on the left of the graph, it seems to us that this is an exhibitor. For example, more recently, Earth’s population has grown with such acceleration. But at some point there is a "saturation", and growth slows down.
When experts are interviewed , it turns out that on average, to wait another 45 years.

What is curious is that North American scientists believe that AI will surpass a person in 74 years, and Asian ones - in just 30. Perhaps in Asia they know something like that ...
The same scientists predicted that the car would translate better than a man by 2024, write school essays by 2026, drive trucks by 2027, play Go, also by 2027. With Guo, the bobble has already come out, because this moment came in 2017, just 2 years after the forecast.
Well, in general, forecasts for 40+ years ahead are a thankless task. It means "sometime." For example, the cost-effective energy of thermonuclear fusion is also predicted in 40 years. The same forecast was given 50 years ago, when it was just begun to be studied.

Strong AI raises a host of ethical issues.
Although a strong AI will wait for a long time, but we know for sure that there will be enough ethical problems. The first class of problems - we can offend the AI. For example:
- Is it ethical to torture an AI if he is able to feel pain?
- Is it normal to leave an AI without communication for a long time if he is able to feel loneliness?
- Can you use it as a pet? And what about a slave? And who will control it and how, because this is a program that works "lives" in your "smartphone"?
Now no one will be indignant if you offend your voice assistant, but if you treat the dog badly, you will be convicted. And this is not because it is made of flesh and blood, but because it feels and experiences a bad relationship, as it will with Strong AI.
The second class of ethical problems - AI can offend us. Hundreds of such examples can be found in films and books. How to explain the AI, what do we want from it? People for AI are like ants for workers building a dam: for the sake of a great goal, you can crush a couple.
Science fiction is playing a trick on us. We used to think that Skynet and Terminators are not there yet, and they will be soon, but for now you can relax. AI in films is often malicious, and we hope that this will not happen in life: after all, we were warned, and we are not as stupid as film characters. At the same time, in our thoughts about the future, we forget to think well about the present.
Machine learning is here
Machine learning allows you to solve a practical problem without explicit programming, but by learning from precedents. You can read more in the article “ In simple words: how machine learning works .”
Since we teach the machine to solve a specific problem, the resulting mathematical model (the so-called algorithm) cannot suddenly want to enslave / save humanity. Do normally - it will be normal. What can go wrong?

Bad intentions
First, the problem itself may not be ethical enough. For example, if we teach drones to kill people with machine learning.

https://www.youtube.com/watch?v=TlO2gcs1YvM
Just recently, a small scandal broke out about this. Google is developing software used for the Project Maven military drone pilot project. Presumably, in the future this could lead to the creation of fully autonomous weapons.

Source

"Greedy" bias
But even if the authors of the machine learning algorithm and do not want to kill people and bring harm, they, nevertheless, often still want to benefit. In other words, not all algorithms work for the good of society, many work for the benefit of the creators. This can often be observed in the field of medicine - it is more important not to cure, but to recommend more treatment.

Well, and sometimes the society itself is not interested in the resulting algorithm being a model of morality. For example, there is a compromise between the speed of traffic and mortality on the roads. We could greatly reduce mortality if we limited the speed to 20 km / h, but then life in big cities would be difficult.
Ethics is only one of the parameters of the system.

It turns out that ethical issues should be among the goals of the system initially.
Ethics are hard to describe formally.
There is one problem with ethics - it is difficult to formalize it. Different countries have different ethics. It changes with time. For example, on issues such as LGBT rights and interracial / inter-caste marriages, opinions may change significantly over the decades. Ethics may depend on the political climate.


Machine learning affects people
Imagine a machine learning based system that advises you which movie to watch. Based on your ratings for other films, and by comparing your tastes with the tastes of other users, the system can quite reliably recommend a film that you’ll like very much.

But at the same time, the system will eventually change your tastes and make them more narrow. Without a system, you would occasionally watch both bad films and films of unusual genres. And so that no movie - to the point. As a result, we cease to be "film experts", and become only consumers of what they give. Another interesting fact is that we do not even notice how the algorithms manipulate us.
If you say that the impact of algorithms on people is even good, here is another example. China is preparing to launch the Social Rating System - a system for evaluating individual citizens or organizations for various parameters, the values of which are obtained using mass monitoring tools and using big data analysis technology.

As a result, it turns out that thanks to the System, citizens consciously or subconsciously begin to behave differently. Communicate less with unreliable citizens, buy more diapers, etc.
Algorithmic system error
Besides the fact that we sometimes do not know what we want from the algorithm, there is also a whole bunch of technical limitations.
The algorithm absorbs the imperfections of the surrounding world.

Microsoft once taught a chat bot to chat on Twitter. It had to be turned off in less than a day, because the bot quickly mastered curses and racist remarks.

In addition, the algorithm during training can not take into account some non-formalizable parameters. For example, when calculating a recommendation to a defendant - whether or not to admit guilt on the basis of collected evidence, the algorithm finds it difficult to take into account what impression such a confession will make on a judge, because the impression and emotions are not recorded anywhere.
False correlations and "feedback loops"
False correlation - this is when it seems that the more firefighters in the city, the more often fires. Or when it is obvious that the fewer pirates on Earth, the warmer the climate on the planet.

So - people suspect that pirates and the climate are not directly related, and firefighters are not so simple, and Matmodel of machine learning simply learns and generalizes.

Worse than false correlations are only feedback loops. The California crime prevention program proposed sending more police officers to the black neighborhoods based on the crime rate (number of recorded crimes). And the more police cars in the field of visibility, the more often residents report crimes (just have someone to report). As a result, crime is only increasing - it means that more police officers must be sent, etc.
In other words, if racial discrimination is a factor of arrest, then feedback loops can reinforce and perpetuate racial discrimination in police activities.
Who is to blame
In 2016, the Big Data Working Group under the Obama Administration released a report warning of “possible coding discrimination when making automated decisions” and postulating the “principle of equal opportunities”.
But it is easy to say something, but what to do?

Secondly, it is difficult for us to understand and explain machine learning solutions. For example, the neural network somehow placed weights within itself so that the correct answers were obtained. And why do they turn out exactly so and what to do to change the answer?

It remains to make laws and rely on machine learning.
It turns out that there is no one to blame, it remains to make laws and postulate the "ethical laws of robotics." Germany just recently, in May 2018, issued such a set of rules about unmanned vehicles. Among other things, it recorded there:
- Human safety is the highest priority compared to damage to animals or property.
- In the event of an imminent accident, there should be no discrimination, and it is unacceptable to distinguish between people by any factors.
But what is especially important in our context:
Automatic driving systems become an ethical imperative if systems cause less accidents than human drivers.
Obviously, we will rely more and more on machine learning - simply because it will generally cope better than people.
Machine learning can be poisoned
And here we come to no less misfortune than the bias of the algorithms - they can be manipulated.
ML poisoning means that if someone takes part in the training of the model, then he can influence the decisions made by the model.
For example, in a computer virus analysis laboratory, a matmodel processes on average a million new samples (clean and malicious files) every day.

So, an attacker can constantly generate malicious files, very similar to some kind of clean, and send them to the laboratory. The border between clean and malicious files will gradually fade, the model will “degrade”. And in the end, the model can recognize the original clean file as malware - a false positive will result.
And vice versa, if you “spam” a self-learning spam filter with a ton of clean generated emails, you will eventually be able to create spam that passes through the filter.
Therefore, in Kaspersky Lab, a multi-layered approach to protection , we do not rely solely on machine learning.
Another example is fictional. Specially generated faces can be added to the facial recognition system to end up confusing you with someone else. Do not think that this is impossible, take a look at the picture from the next section.

Hacking machine learning
Poisoning is an effect on the learning process. But it is not necessary to participate in training in order to get the benefit - you can deceive a ready-made model if you know how it works.


This example has not yet been encountered by individuals in the “wild” - precisely because no one has yet entrusted the car to make important decisions based on facial recognition. Without control from the person will be exactly as in the picture.
Even where, seemingly, there is nothing difficult, the car is easy to deceive in a way unknown to the uninitiated.
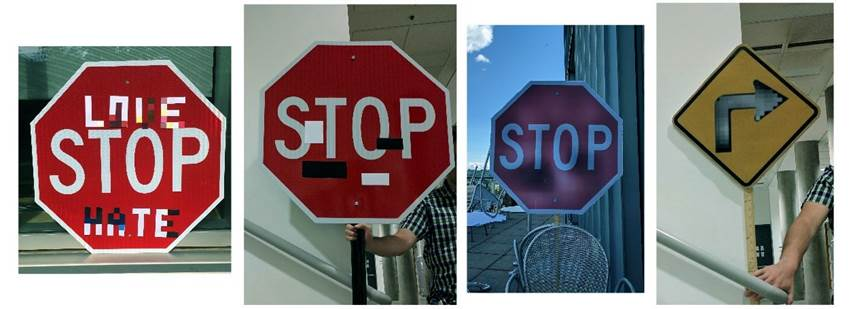
The first three characters are recognized as “Speed limit 45”, and the last one as a STOP character.

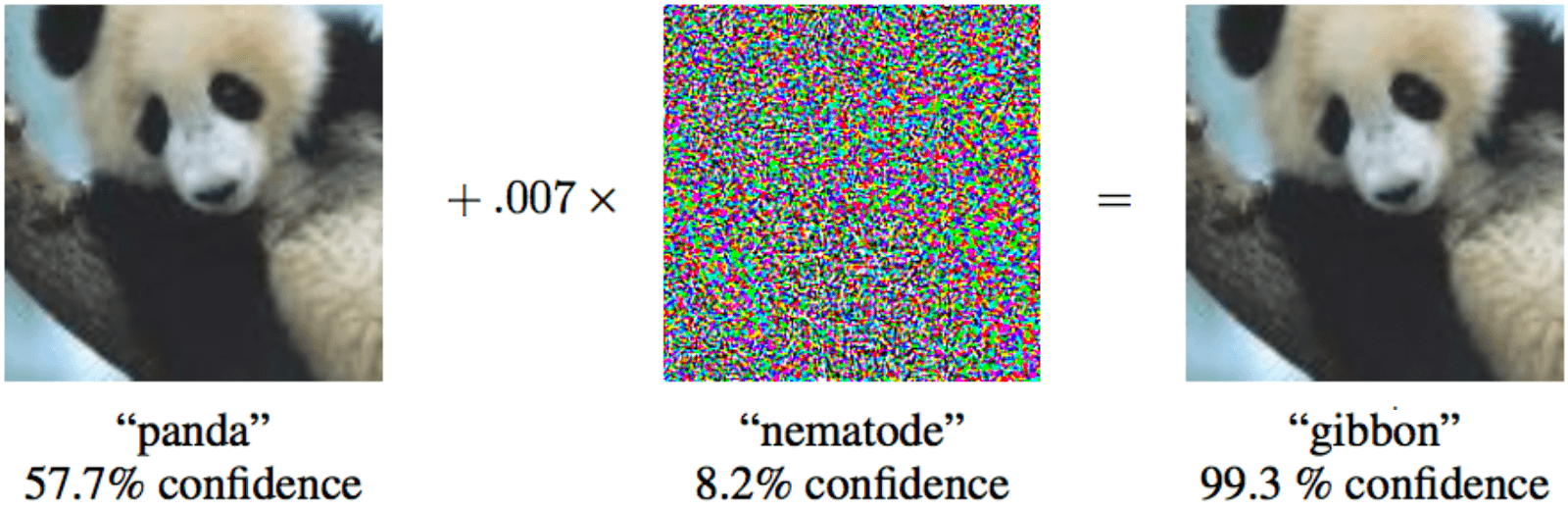
If you add the minimum special noise to the panda on the left, then machine learning will be sure that it is a gibbon.

Similarly, it will be possible to “hack” the Chinese Social Rating System and become the most respected person in China.
Conclusion
Let's summarize what we managed to discuss.


- There is no strong AI yet.
- We are relaxed.
- Machine learning will reduce the number of victims in critical areas.
- We will rely on machine learning more and more.
- We will have good intentions.
- We will even lay ethics into systems design.
- But ethics is hard formalized and different in different countries.
- Machine learning is full of bias for various reasons.
- We can not always explain the solutions of machine learning algorithms.
- Machine learning can be poisoned.
- And even "hack".
- An attacker can gain an advantage over other people.
- Machine learning has an impact on our lives.
And all this is the near future.