And we are all "vertically" - DBMS Vertica
Hello! My name is Sergey, I work as a chief engineer in Sbertech. I have been in the IT field for about 10 years, of which 6 have been working on databases, ETL processes, DWH, and everything related to data. In this article, I will talk about Vertica - an analytical and truly column-based DBMS, which effectively compresses, stores, quickly transfers data and perfectly suits as a big data solution.

Since the 2000s, big data began to develop and it took engines that could digest all this. In response to this, a number of columnar DBMS for this purpose appeared - including Vertica.
Vertica does not just store its data in columns, it does it efficiently, with a high degree of compression, but also effectively schedules requests and quickly sends data. Information that takes up about 1 TB of disk space in a classic lower-level DBMS, on Vertica will take about 200-300 GB, thus we get good savings on disks.
Vertica was originally designed as a column DBMS. Other databases basically only try to imitate various columnar mechanisms, but they are not always good at it, because the engine is still created to handle strings. As a rule, imitators simply transpose the table and then process it with a familiar string mechanism.
Vertica is fault tolerant, there is no control node in it - all nodes are equal. If problems arise with one of the servers in the cluster, we will still receive the data. Very often, getting data on time is critical for business customers, especially during the period when reporting is closed and information must be provided to financial authorities.
Vertica is primarily an analytical data warehouse. It should not be written in small transactions, it is not necessary to fasten it to some site, etc. Vertica should be considered as a kind of batch-layer, where you should immerse the data in large batches. If necessary, Vertica is very quickly ready to give this data - requests for millions of rows are executed in seconds.
Where can this be useful? Take for example the telecommunications company. Vertica can be used in it for geoanalytics, network development, quality management, targeted marketing, studying information from contact centers, managing customer outflow and antifraud / anti-spam solutions.

In other branches of business, everything is about the same - timely and reliable analytics is important for making a profit. In trade, for example, everyone is trying to somehow personalize customers, distribute discount cards for this, collect data on where and when a person bought, etc. Analyzing the data arrays from all these channels, we can compare it, build models and make decisions leading to profit growth.
Today, any employer requires the analyst to understand what SQL is. If you know ANSI SQL, then you can be called a confident Vertica user. If you can build models in Python and R, then you are just a "massage therapist" of the data. If you have mastered Linux and have a basic knowledge of Vertica administration, then you can work as an administrator. In general, the entrance threshold in Vertica is low, but, of course, all the nuances can be learned only by filling your hand during the operation.
Consider Vertica at the cluster level. This DBMS provides massively parallel data processing (MRR) in a distributed computing architecture - “shared-nothing” - where, in principle, any node is ready to pick up the functions of any other node. Basic properties:
The cluster without problems is linearly scaled. We just put the servers in the shelf and connect them via a graphical interface. In addition to serial servers, deployment to virtual machines is possible. What can be achieved with the help of the extension?
But something needs to be considered. Periodically, nodes must be removed from the cluster for maintenance. Another fairly common case in a large organization is that servers leave the guarantee and move from productive to some kind of test environment. In their place are new, which are under warranty of the manufacturer. Following all these operations, rebalancing must be performed. This is the process when data is redistributed between nodes - the workload is redistributed accordingly. This is a resource-demanding process, and on clusters with large amounts of data, it can severely reduce performance. To avoid this, you need to select the service window - the time when the load is minimal, and in this case, users will not notice.
To understand how data is stored in Vertica, you need to deal with one of the basic concepts - projection.
Logical information storage units are schemas, tables, and views. Physical units are projections. There are several types of projections:
When creating any table, a superprojection is automatically created , which contains all the columns of our table. If you need to speed up any of the regular processes, we can create a special query-oriented projection , which will contain, say, 3 columns out of 10.
For the acceleration, the third type is intended - aggregated projections . I will not go into their subclasses - this is not very interesting. I just want to warn you that it’s not worthwhile to constantly solve your problems with fulfilling requests through the creation of new projections. Eventually, the cluster will begin to slow down.
When creating projections, it is necessary to evaluate whether our requirements are sufficient for superprojections. If we still want to experiment, we add strictly one new projection. If problems arise, it will be easier to find the root cause. For large tables, create a segmented projection. It is divided into segments, which are distributed over several nodes, which increases fault tolerance and minimizes the load on one node. If the plates are small, then it is better to do non-segmented projections. They are completely copied to each node, and productivity is thus increased. I will make a reservation: in terms of Vertica, a “small” table is approximately 1 million rows.
Vertica fault tolerance is implemented using the K-Safety mechanism. It is quite simple in terms of description, but difficult in terms of work at the engine level. It can be controlled using the K-Safety parameter - it can be 0, 1 or 2. This parameter specifies the number of copies of the segmented projection data.
Copies of the projections are called buddy projections. I tried to translate this phrase through Yandex-translator and it turned out something like a “projection-sidekick”. Google offered options and more interesting. Typically, these projections are called partner or neighboring, according to their functional purpose. These are projections that are simply stored on neighboring nodes and thus reserved. Non-segmented projections have no buddy projections - they are copied completely.
How it works? Consider a cluster of five cars. Let K-safety be equal to 1. The

nodes are numbered, and under them are written partner projections that are stored on them. Suppose we have one node turned off. What will happen?

Node 1 contains a friendly projection of node 2. Therefore, load 1 will grow at node 1, but the cluster will not stop working. And now this situation:
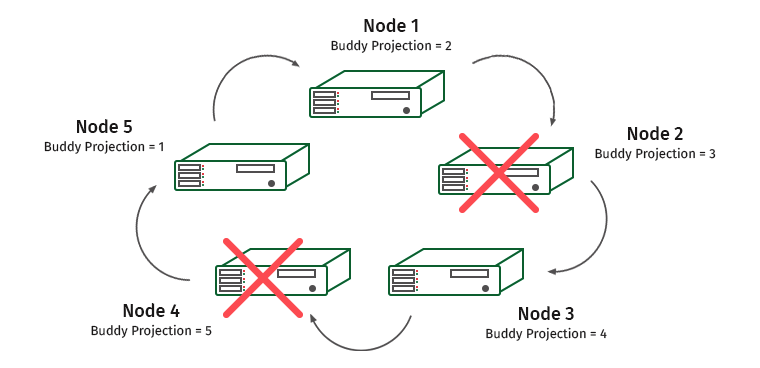
Node 3 contains a projection of node 4, and node 1 and 3 will be overloaded.
Complicate the task. K-Safety = 2, disable two adjacent nodes.
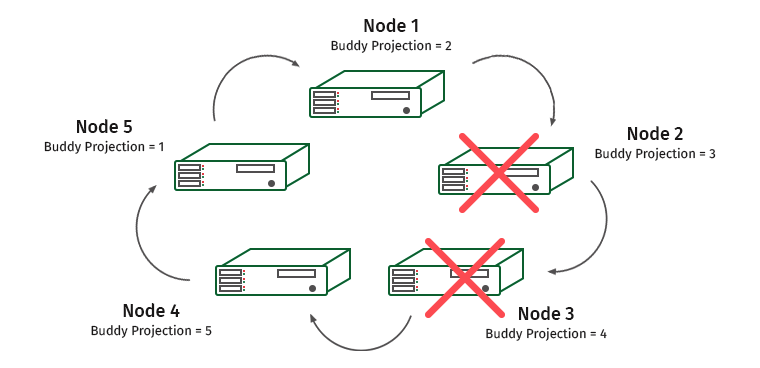
Nodes 1 and 4 will be overloaded here (node 2 contains a projection of node 1, and node 3 contains a projection of node 4).
In such situations, the system engine recognizes that one of the nodes does not respond, and the load is transferred to the neighboring node. It will be used until the node is restored again. As soon as this happens, the load and data are redistributed back. As soon as we lose more than half of the cluster or node containing all copies of some data, the cluster rises.
Vertica has data storage areas that are optimized for writing, areas that are optimized for reading, and a Tuple Mover mechanism that allows data to flow from the first to the second.
When using the operation COPY, INSERT, UPDATE, we automatically get into the WOS (Write Optimized Store) - an area where the data is not optimized for reading and sorted only when requested, stored without compression or indexing. If the data volumes are too large for the WOS area, then using the additional DIRECT instruction, it is worth writing them directly to ROS. Otherwise, the WOS will be full, and we will have a failure.
After the time specified in the settings, the data from WOS flow into the ROS (Read Optimized Store) - an optimized, read-oriented structure of disk storage. ROS stores the bulk of the data, here it is sorted and compressed. Data in ROS is divided into storage containers. A container is a set of lines created by translation operators (COPY DIRECT) and stored in a specific group of files.
Regardless of where the data is written - in WOS or in ROS - they are available immediately. But from WOS, reading is slower, because the data is not grouped there.

Tuple Mover is a cleaner tool that performs two operations:
If we read lines, for example, to execute a command, we will have to read the entire table. This is a huge amount of information. In this case, the column approach is more profitable. It allows you to count only the three columns we need, saving memory and time.

To avoid any problems, the user needs to be slightly limited. There is always a chance that the user will write a heavy request that will eat all the resources. By default, Vertica occupies a significant part of the General area, and in addition there are separate areas for Tuple Mover, WOS and system processes (recovery, etc.).
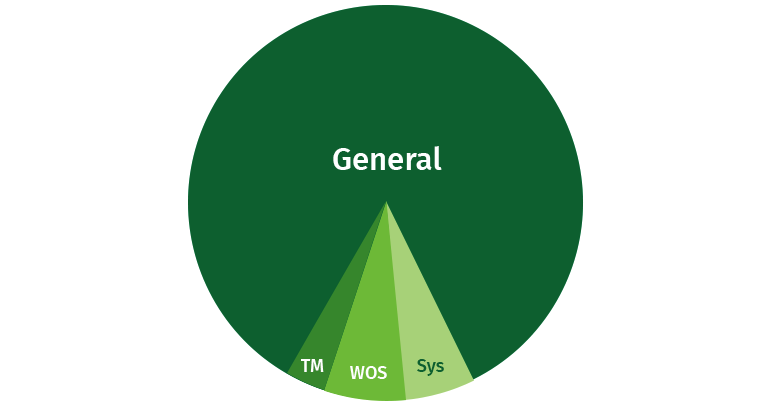
Let's try to share these resources. We create areas for writers, for readers, and for slow requests with low priority.

If you look at the system tables that store our resources - resource pools - then we will see a lot of parameters with which you can adjust everything more finely. At the start, you shouldn’t go into it; it’s better to just confine yourself to cutting off memory for certain tasks. When you gain experience and you are 100% sure that you are doing everything right, you can experiment.
By fine tuning can be attributed to the priority of implementation, competitive sessions, the amount of allocated memory. And even with processors, we can fix something. To work with these settings, you need complete confidence in the correctness of our actions, so it’s better to enlist the support of a business and have the right to make a mistake.
Below is an example of a request with which you can see the settings of the General pool:
Vertica, like all current tools, is seriously integrated with other systems. Can work well with HDFS (Hadoop). In earlier versions, Vertica could only download data from HDFS of certain formats, but now it can do everything, works with all formats, for example, ORC and Parquet. It can even attach files as external tables (external tables) and store its data in ROS containers directly on HDFS. In the eighth version of Vertica, a significant optimization of the speed of working with HDFS, the metadata catalog and parsing of these formats was carried out. You can build a Vertica cluster right on the Hadoop cluster.
Starting with version 7.2, Vertica can work with Apache Kafka - if someone needs a message broker.
Vertica 8 has full Spark support. It is possible to copy data from Spark to Vertica and back.
Vertica is a good option for working with big data, which does not require a lot of input knowledge. This DBMS has wide analytical capabilities. Of the minuses - this solution is not open source, but you can try to deploy for free with a limit of 1 TB and three nodes - this is quite enough to understand whether you need Vertica or not.

general information
Since the 2000s, big data began to develop and it took engines that could digest all this. In response to this, a number of columnar DBMS for this purpose appeared - including Vertica.
Vertica does not just store its data in columns, it does it efficiently, with a high degree of compression, but also effectively schedules requests and quickly sends data. Information that takes up about 1 TB of disk space in a classic lower-level DBMS, on Vertica will take about 200-300 GB, thus we get good savings on disks.
Vertica was originally designed as a column DBMS. Other databases basically only try to imitate various columnar mechanisms, but they are not always good at it, because the engine is still created to handle strings. As a rule, imitators simply transpose the table and then process it with a familiar string mechanism.
Vertica is fault tolerant, there is no control node in it - all nodes are equal. If problems arise with one of the servers in the cluster, we will still receive the data. Very often, getting data on time is critical for business customers, especially during the period when reporting is closed and information must be provided to financial authorities.
Areas of use
Vertica is primarily an analytical data warehouse. It should not be written in small transactions, it is not necessary to fasten it to some site, etc. Vertica should be considered as a kind of batch-layer, where you should immerse the data in large batches. If necessary, Vertica is very quickly ready to give this data - requests for millions of rows are executed in seconds.
Where can this be useful? Take for example the telecommunications company. Vertica can be used in it for geoanalytics, network development, quality management, targeted marketing, studying information from contact centers, managing customer outflow and antifraud / anti-spam solutions.

In other branches of business, everything is about the same - timely and reliable analytics is important for making a profit. In trade, for example, everyone is trying to somehow personalize customers, distribute discount cards for this, collect data on where and when a person bought, etc. Analyzing the data arrays from all these channels, we can compare it, build models and make decisions leading to profit growth.
Input threshold
Today, any employer requires the analyst to understand what SQL is. If you know ANSI SQL, then you can be called a confident Vertica user. If you can build models in Python and R, then you are just a "massage therapist" of the data. If you have mastered Linux and have a basic knowledge of Vertica administration, then you can work as an administrator. In general, the entrance threshold in Vertica is low, but, of course, all the nuances can be learned only by filling your hand during the operation.
Hardware architecture
Consider Vertica at the cluster level. This DBMS provides massively parallel data processing (MRR) in a distributed computing architecture - “shared-nothing” - where, in principle, any node is ready to pick up the functions of any other node. Basic properties:
- there is no single point of failure,
- each node is independent and independent
- there is no single point of connection for the whole system,
- infrastructure nodes are duplicated,
- data on cluster nodes is automatically copied.
The cluster without problems is linearly scaled. We just put the servers in the shelf and connect them via a graphical interface. In addition to serial servers, deployment to virtual machines is possible. What can be achieved with the help of the extension?
- Increase volume for new data
- Increase maximum workload
- Increased resiliency. The larger the nodes in the cluster, the less likely the cluster will fail due to a failure, and therefore, the closer we are to ensuring 24/7 availability.
But something needs to be considered. Periodically, nodes must be removed from the cluster for maintenance. Another fairly common case in a large organization is that servers leave the guarantee and move from productive to some kind of test environment. In their place are new, which are under warranty of the manufacturer. Following all these operations, rebalancing must be performed. This is the process when data is redistributed between nodes - the workload is redistributed accordingly. This is a resource-demanding process, and on clusters with large amounts of data, it can severely reduce performance. To avoid this, you need to select the service window - the time when the load is minimal, and in this case, users will not notice.
Projections
To understand how data is stored in Vertica, you need to deal with one of the basic concepts - projection.
Logical information storage units are schemas, tables, and views. Physical units are projections. There are several types of projections:
- Superprojection,
- query-oriented projections (Query-Specific Projections),
- aggregated projections (Aggregate Projections).
When creating any table, a superprojection is automatically created , which contains all the columns of our table. If you need to speed up any of the regular processes, we can create a special query-oriented projection , which will contain, say, 3 columns out of 10.
For the acceleration, the third type is intended - aggregated projections . I will not go into their subclasses - this is not very interesting. I just want to warn you that it’s not worthwhile to constantly solve your problems with fulfilling requests through the creation of new projections. Eventually, the cluster will begin to slow down.
When creating projections, it is necessary to evaluate whether our requirements are sufficient for superprojections. If we still want to experiment, we add strictly one new projection. If problems arise, it will be easier to find the root cause. For large tables, create a segmented projection. It is divided into segments, which are distributed over several nodes, which increases fault tolerance and minimizes the load on one node. If the plates are small, then it is better to do non-segmented projections. They are completely copied to each node, and productivity is thus increased. I will make a reservation: in terms of Vertica, a “small” table is approximately 1 million rows.
fault tolerance
Vertica fault tolerance is implemented using the K-Safety mechanism. It is quite simple in terms of description, but difficult in terms of work at the engine level. It can be controlled using the K-Safety parameter - it can be 0, 1 or 2. This parameter specifies the number of copies of the segmented projection data.
Copies of the projections are called buddy projections. I tried to translate this phrase through Yandex-translator and it turned out something like a “projection-sidekick”. Google offered options and more interesting. Typically, these projections are called partner or neighboring, according to their functional purpose. These are projections that are simply stored on neighboring nodes and thus reserved. Non-segmented projections have no buddy projections - they are copied completely.
How it works? Consider a cluster of five cars. Let K-safety be equal to 1. The

nodes are numbered, and under them are written partner projections that are stored on them. Suppose we have one node turned off. What will happen?

Node 1 contains a friendly projection of node 2. Therefore, load 1 will grow at node 1, but the cluster will not stop working. And now this situation:
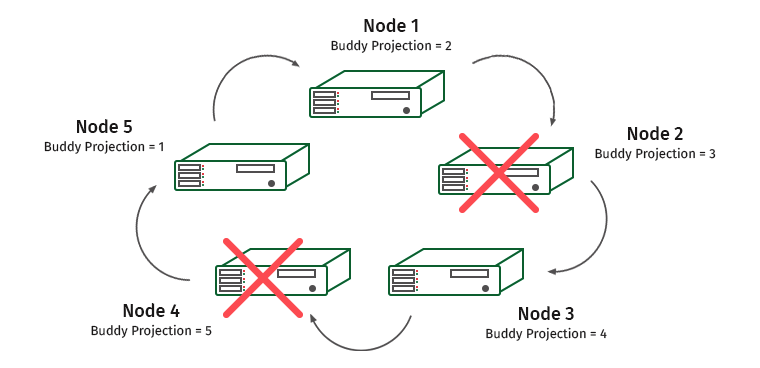
Node 3 contains a projection of node 4, and node 1 and 3 will be overloaded.
Complicate the task. K-Safety = 2, disable two adjacent nodes.
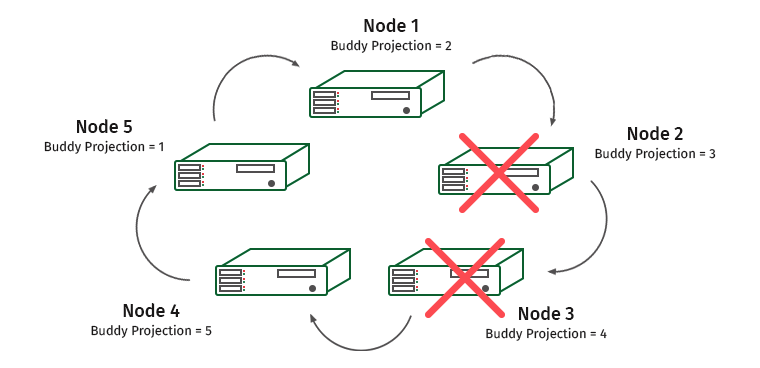
Nodes 1 and 4 will be overloaded here (node 2 contains a projection of node 1, and node 3 contains a projection of node 4).
In such situations, the system engine recognizes that one of the nodes does not respond, and the load is transferred to the neighboring node. It will be used until the node is restored again. As soon as this happens, the load and data are redistributed back. As soon as we lose more than half of the cluster or node containing all copies of some data, the cluster rises.
Logical data storage
Vertica has data storage areas that are optimized for writing, areas that are optimized for reading, and a Tuple Mover mechanism that allows data to flow from the first to the second.
When using the operation COPY, INSERT, UPDATE, we automatically get into the WOS (Write Optimized Store) - an area where the data is not optimized for reading and sorted only when requested, stored without compression or indexing. If the data volumes are too large for the WOS area, then using the additional DIRECT instruction, it is worth writing them directly to ROS. Otherwise, the WOS will be full, and we will have a failure.
After the time specified in the settings, the data from WOS flow into the ROS (Read Optimized Store) - an optimized, read-oriented structure of disk storage. ROS stores the bulk of the data, here it is sorted and compressed. Data in ROS is divided into storage containers. A container is a set of lines created by translation operators (COPY DIRECT) and stored in a specific group of files.
Regardless of where the data is written - in WOS or in ROS - they are available immediately. But from WOS, reading is slower, because the data is not grouped there.

Tuple Mover is a cleaner tool that performs two operations:
- Moveout - compresses and sorts data in WOS, moves them to ROS and creates new containers for them in ROS.
- Mergeout - sweeps up after us when we use DIRECT. We are not always able to load so much information to get large ROS-containers. Therefore, it periodically merges small ROS containers into larger ones, clears data marked for deletion, while working in the background (according to the time specified in the configuration).
What are the benefits of column storage?
If we read lines, for example, to execute a command, we will have to read the entire table. This is a huge amount of information. In this case, the column approach is more profitable. It allows you to count only the three columns we need, saving memory and time.
SELECT 1,11,15 from table1

Resource allocation
To avoid any problems, the user needs to be slightly limited. There is always a chance that the user will write a heavy request that will eat all the resources. By default, Vertica occupies a significant part of the General area, and in addition there are separate areas for Tuple Mover, WOS and system processes (recovery, etc.).
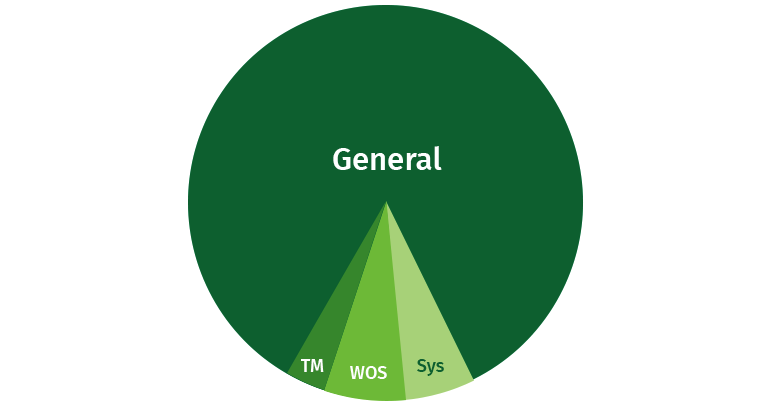
Let's try to share these resources. We create areas for writers, for readers, and for slow requests with low priority.

If you look at the system tables that store our resources - resource pools - then we will see a lot of parameters with which you can adjust everything more finely. At the start, you shouldn’t go into it; it’s better to just confine yourself to cutting off memory for certain tasks. When you gain experience and you are 100% sure that you are doing everything right, you can experiment.
By fine tuning can be attributed to the priority of implementation, competitive sessions, the amount of allocated memory. And even with processors, we can fix something. To work with these settings, you need complete confidence in the correctness of our actions, so it’s better to enlist the support of a business and have the right to make a mistake.
Below is an example of a request with which you can see the settings of the General pool:
dbadmin => select * FROM resource_pools WHERE NAME = 'general';
-[ RECORD 1 ]------------+---------------
pool_id | 45035996273721212
name | general
is_internal | t
memorysize |
maxmemorysize | 30G
executionparallelism | AUTO
priority | 0
runtimepriority | MEDIUM
runtimeprioritythreshold | 2
queuetimeout | 0:05
plannedconcurrency | 10
maxconcurrency | 20
runtimecap |
singleinitiator | f
cpuaffinityset |
cpuaffinitymode | ANY
cascadeto |
ANSI SQL and other features
- Vertica allows you to write in SQL-99 - all functionality is supported.
- Verica has great analytical power - even machine learning tools are included in the delivery.
- Vertica can index texts
- Vertica processes semi-structured data
Integration
Vertica, like all current tools, is seriously integrated with other systems. Can work well with HDFS (Hadoop). In earlier versions, Vertica could only download data from HDFS of certain formats, but now it can do everything, works with all formats, for example, ORC and Parquet. It can even attach files as external tables (external tables) and store its data in ROS containers directly on HDFS. In the eighth version of Vertica, a significant optimization of the speed of working with HDFS, the metadata catalog and parsing of these formats was carried out. You can build a Vertica cluster right on the Hadoop cluster.
Starting with version 7.2, Vertica can work with Apache Kafka - if someone needs a message broker.
Vertica 8 has full Spark support. It is possible to copy data from Spark to Vertica and back.
Conclusion
Vertica is a good option for working with big data, which does not require a lot of input knowledge. This DBMS has wide analytical capabilities. Of the minuses - this solution is not open source, but you can try to deploy for free with a limit of 1 TB and three nodes - this is quite enough to understand whether you need Vertica or not.