Why AlphaGo is not artificial intelligence
- Transfer
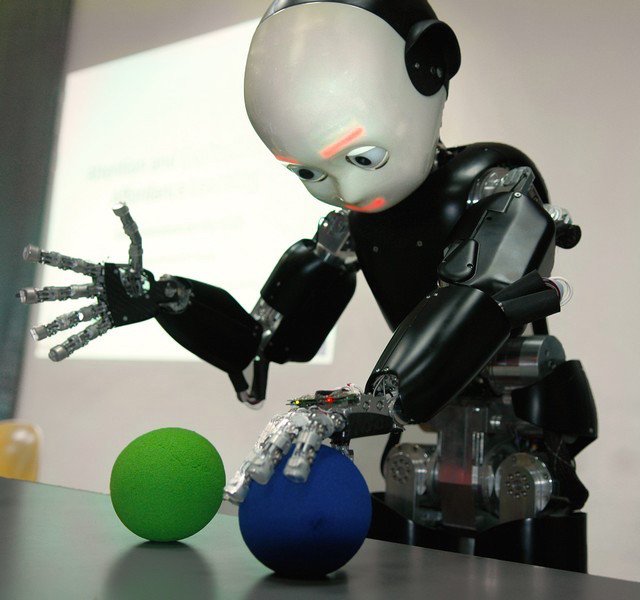
What can be called AI, and what is impossible - in a sense, depends on the definition. It is impossible to deny that AlphaGo — AI, playing go, developed by the Google DeepMind team, and beating the world champion — as well as similar systems with in-depth training over the past few years have been able to solve quite complex computational problems. But will they lead us to a real, full AI, to a generalized intellect, or an OI? Hardly - and here, why.
One of the key features of the Olympics, which we have to deal with when creating it, is that it should be able to independently deal with the outside world and develop its own, inner understanding of everything that it encounters, hear, say, or do. Otherwise, you will have on your hands modern AI programs, the meaning of which was laid by the developer of the application. AI, in fact, does not understand what is happening and its area of specialization is very narrow.
The problem of meaning is perhaps the most fundamental of the problems of AI, and it has not been solved yet. One of the first people to express it was the cognitive scholar Stevan Harnad, who wrote in 1990, The Problem of Matching Characters. Even if you do not believe that we are manipulating with symbols, the task still remains: comparing the view that exists within the system with the real world.
To be concrete, let us note that the task of reflection leads us to four subtasks:
1. How to structure the information that a person (person or AI) receives from the outside world?
2. How to connect this structured information with the world, that is, how to build a person’s understanding of the world?
3. How to synchronize this understanding with other personalities? (Otherwise, communication will be impossible, and intelligence will turn out to be inexplicable and detached).
4. Why does a person do something? How to start all this movement?
The first problem, structuring, is well solved through in-depth training and similar learning algorithms that do not require supervision - for example, it is used in AlphaGo. In this area, we have achieved significant success, in particular due to the recent increase in computing power and the use of GPUs, which are particularly well parallelize the processing of information. These systems work by taking an excessively excessive signal, expressed in multidimensional space, at the input and reduce it to signals of smaller dimensions, minimizing the loss of information. In other words, they capture an important part of the signal in terms of information processing.
The second problem, the problem of communication of information with the real world, that is, the creation of “reflection”, is directly related to robotization. To interact with the world, you need a body, and to build this connection you need to interact with the world. Therefore, I often say that there is no AI without robotization (although there are excellent robots without AI, but this is another story). This is often called the “incarnation problem”, and most AI researchers agree that intelligence and incarnation are closely related. In different bodies there are different forms of intelligence, which can easily be seen in animals.
Everything starts with such simple things as finding meaning in your own parts of the body and how to control them to achieve the desired effect in the visible world, how the sense of space, distance, color, etc. is built. In this area, detailed research has been conducted by such a scientist as J. Kevin O'Regan, known for his "sensorimotor theory of consciousness." But this is only the first step, since then it will be necessary to build more and more abstract concepts based on these mundane sensorimotor structures. We have not yet got to this, but research on this topic is already underway.
The third problem is the question of the origin of culture. In some animals, the simplest forms of culture can be traced, and even capabilities transmitted through generations, but in very limited scope, and only humans have reached the threshold of the exponential growth of acquired knowledge that we call culture. Culture is a catalyst of intelligence, and AI, which does not have the possibility of cultural interaction, will be of academic interest only.
But culture cannot be manually entered into the machine as a code. It should be the result of a learning process. The best way to look for ways to understand this process is developmental psychology.. The work on this topic was done by Jean Piaget and Michael Tomasello, who studied the process of acquiring cultural knowledge by children. This approach spawned a new discipline of robotics, “robotic development,” taking the child as a model (as in the case of the iCub robot in the illustration).
Also, this question is closely related to the study of the process of learning the language, and this is one of the topics that I personally study. The work of people like Luc Steels [Luc Steels] and others showed that the process of acquiring a language can be compared with evolution: a person creates new concepts, interacting with the world, using them to communicate with other personalities, and selects structures that help to communicate more successfully than others (mainly to achieve joint goals). After hundreds of trial and error, as in the case of biological evolution, the system generates the best concept and its syntactic / grammatical translation.
This process was tested experimentally, and it is surprisingly similar to how natural languages evolve and grow. He is also responsible for instant learning, when the individual perceives the concept immediately - such things are not able to explain such statistics-based models as deep learning. Now several research laboratories using this approach are trying to go further along the path of perception of grammar, gestures and more complex cultural phenomena. Specifically, this is AI Lab, founded by me in the French company engaged in robotics Aldebaran. Now it has become part of the SoftBank Group - it was they who created the Nao , Romeo and Pepper robots (below).
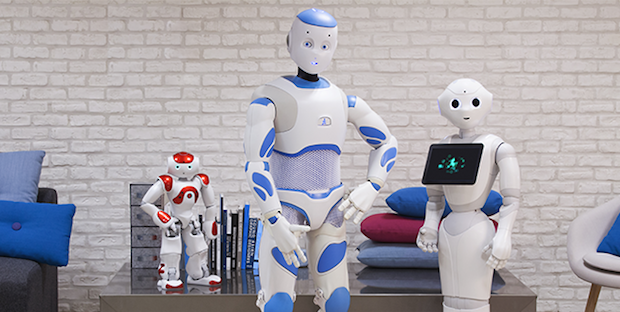
And finally, the fourth problem is faced with "internal motivation". Why does a person do anything at all, and not just be at rest. Survival requirements are not enough to explain human behavior. Even if you feed and ensure the safety of a person, he does not sit waiting for the return of hunger. People study the environment, try to do something, and are driven by some form of curiosity inherent in them. Researcher Pierre-Yves Udeier [Pierre-Yves Oudeyer] showed that the simplest mathematical expression of curiosity in the form of a person’s striving to maximize learning speed is enough to produce surprisingly complex and unexpected behavior (see, for example, an experiment with a “playground”, conducted in Sony CSL).
Apparently, something similar is necessary for the system to induce in it the desire to go through the three previous steps: to structure information about the world, combine it with your body and create meaningful concepts, and then choose the most effective from the point of view of communication in order to create a joint a culture in which cooperation is possible. This, from my point of view, is the OI program.
I repeat that the rapid development of in-depth training and the recent successes of such AI in go-type games are very good news, since such AI will find many applications in medical research, industry, environmental conservation, and other areas. But this is only one part of the problem, which I tried to explain here. I do not believe that deep learning is a panacea that will lead us to this AI, in the sense of a machine that can learn to live in peace, interact with us in a natural way, understand the complexity of our emotions and cultural distortions and ultimately help us make the world better.