Researchers at MIT have taught neural networks to argue their decisions
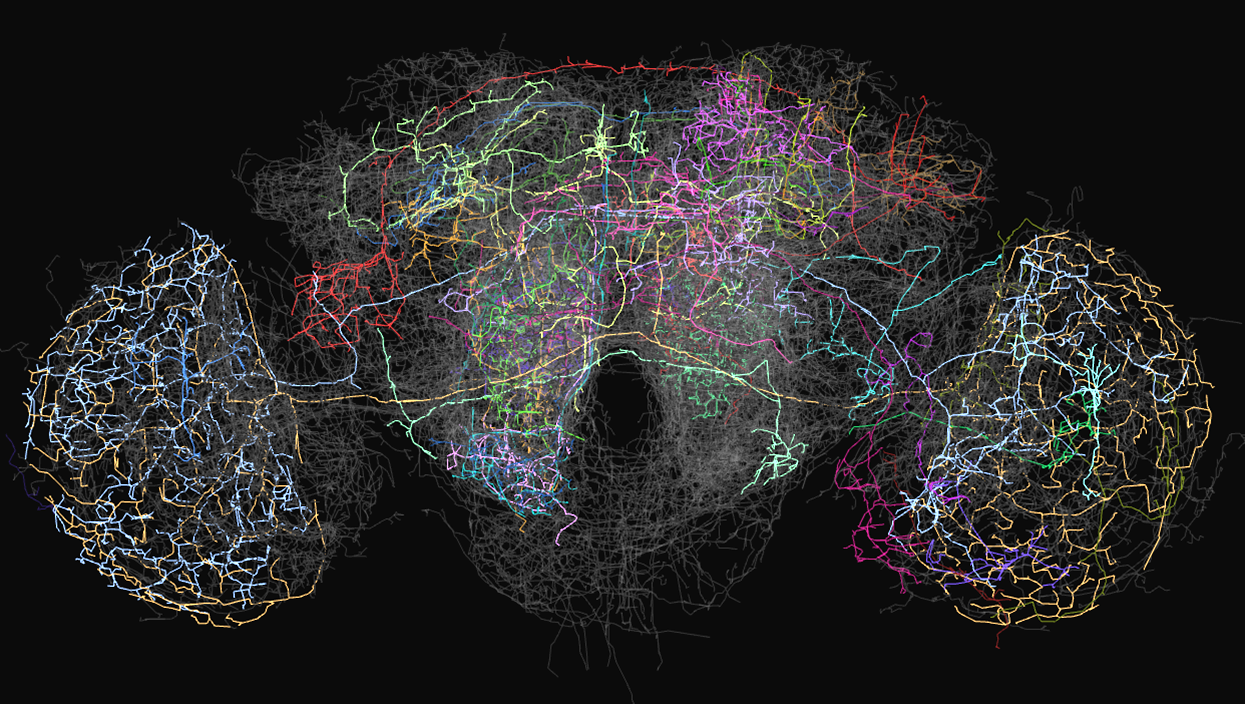
Recently, neural networks have shown themselves to be excellent in many applications. They searched for patterns in the data used for classification and forecasting. Neural networks with apparent ease recognized objects in digital images or, “having read” an excerpt of the text, summed up its subject. However, no one could tell what transformations passed the input data to obtain a solution. Even network authors possessed input data and output information. And if we consider the visual data, sometimes it is even possible to automate the experiments to determine which components of the images the neural network responds to. And with text processing systems, the process is more complicated. What is the difficulty of understanding the human language machine, you can read below.
In the laboratory of CSAIL (laboratories of computer science and artificial intelligence) of the Massachusetts Institute of Technology, neural network researchers made it so that now the “virtual brain” in addition to the solution also provides its rationale. They trained two modules of the same neural network at the same time. Data for learning were text passages. The results pleased: the computer was thinking, like people, in 95% of cases. And yet, before launching a new method of neural networks for active use, additional configuration and refinement will be required.
Why are pictures easier to process than text? Will it be possible for unmanned vehicles to drive freely, is it permissible to replace a live doctor with a programmed intelligence, within which countless neurons? Does this bring us closer to conscious machines in real life? Computer models of neural networks behave in the same way as the human brain, but they have not yet been allowed to make decisions that affect people's lives. To change this, the specialists took time and now we can find out how the neural network comes to the final values.
Sometimes in the world of real-world applications, people want to know why the machine made just such a prediction, and not any other. The main reason that doctors do not trust AI decisions is the lack of information about the decision making process. This also applies to other areas, too - any where the cost of an incorrectly made forecast is high. Therefore, everyone needs proof and guarantees. Most likely, everything is actually even wider: you can not only want to confirm the correctness of the forecast model, but also find out how you can influence what is happening with the help of analysis. As an ordinary person can understand a complex model that is trained on unknown algorithms. These algorithms can tell about the rationality of a particular solution. On Giktymes already asked a question on the topic. And now we can respond positively.
Neural networks - what?
The name “artificial neural networks” means that these structures behave approximately like human brain structures. A component unit of such a network is a processing node, which, like a neuron, can itself perform the simplest operations. Power appears when multiple nodes merge into one huge network. Most of the unknown work takes place in the neuron. This is it - a black box. The data on the input and output can be found. During training, the operations performed by individual nodes are constantly changing in order to get good results across a set of examples for training. By the end of the process, the network programmer does not know what the parameters of the nodes are now. Even if these data were available, it would be difficult to understand this low-level information so as to be able to translate it into a language understandable to humans.
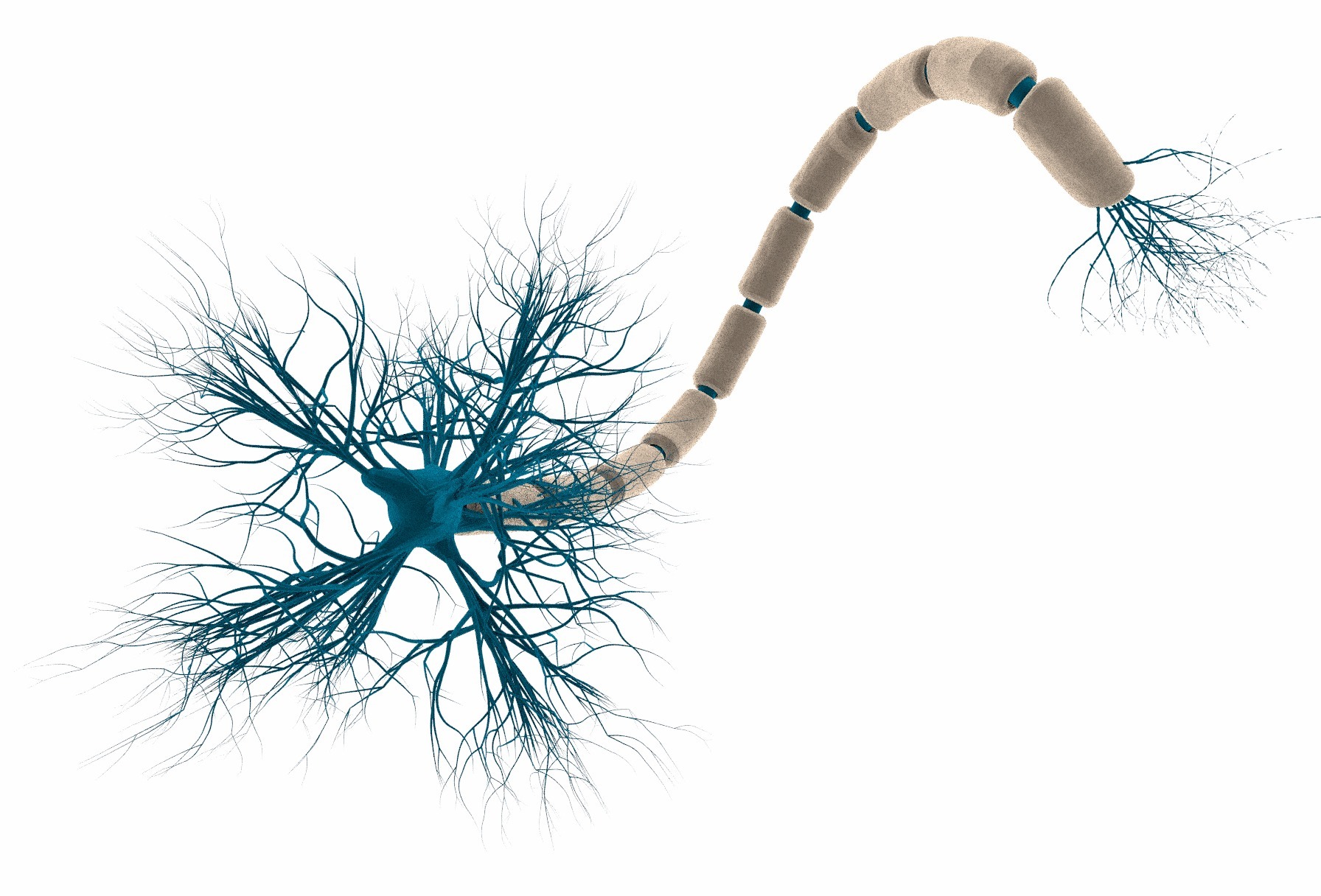
In the process of deep learning, the data goes to the input nodes of the network, which transform them and transfer them to the following nodes. The last action is repeated many times. The process stops when values arrive at the output nodes of the network. Information is correlated with the data area in which learning takes place. These can be objects in the image or subject of the article.
How the process became transparent
To understand how a neural network makes decisions, the researchers decided to train it on textual data. In the institute laboratory, a team of specialists divided the created network into two parts. One was designed to extract pieces of text from training data and evaluate them in length and consistency. The shorter the passage and the greater part of it consists of lines of consecutive words, the higher the score.
Further passages came in the second part. The second part of the neural network predicted the topic of the passage or tried to classify the text. For the test, online reviews from the beer rating site were used. The network of scientists tried to evaluate beer brands on a five-star scale, based on the factors: flavor, taste, appearance, and written reviews. After learning the system, the researchers found that their neural network evaluates flavor and appearance as well as real people: 95% and 96%, respectively. According to a more subjective characteristic of taste, the neural network "agreed" with people in 80% of cases.
The modules were trained together, and the goal of the training was to maximize the assessment of the selected segments and the accuracy of the prediction or classification.
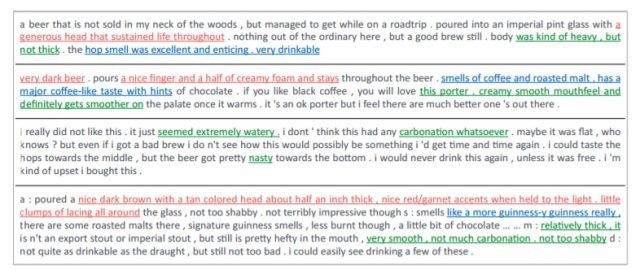
The illustration shows an example of a beer review with a ranking in two categories. If the first subnet chose these three phrases and the second subnet associated them with the correct ratings, then the system used the same thing to judge as the person. The researchers also tested the neural network based on a database of free questions and answers to technical topics. The question was whether a specific answer had already been given before.
Scientists have applied this method to thousands of results of biopsies with breast cancer pathology. Analyzed text and images.
What is the difficulty for the machine to understand human language?
“It’s hard to give an answer if you don’t understand the question.” Sarek, Spock's father in Star Trek - 4: Coming Home.
Natural language processing is one of the trends in artificial intelligence. Our point of view and a wide range of knowledge about the world and an understanding of the context influence the way we perceive even the most elementary grammar structures that link words into meaningful phrases and sentences.
Let me explain with an example, which is given in the book “Calculate the Future” by Eric Siegel. For example, phrases like of India, of milk, of your. Each such part of a sentence may play a different role depending on the words that appear before and after the phrase. The specific definition will be based on an understanding of what words mean and what are the real things that they call.
1. “Time flies like an arrow.”
2. “Fruit flies like a banana.”
If someone does not know these English linguistic puzzles, try to translate sentences yourself in several ways.
Time flies like an arrow.
Flies of time like some kind of arrow.
Measure the speed of flies as you measure the speed of an arrow.
Fruit flies like a banana.
Drosophila love banana.
The same preposition can mean different things. Especially the preposition WITH.
“I ate porrige with fruits.” I ate porridge with fruits that were part of the dish.
“I ate breakfast with spoon.” I ate breakfast with a spoon, which was a tool.
“I ate breakfast with my mom.” I had breakfast with my mom, who was a participant in the action.
The use of neural networks
Classification tasks. This is just a search for patterns, face recognition.
Forecasting tasks. How users behave in some situations. For example, banks calculate the likelihood of repayment of loans when making a decision on extradition. They also investigate the value of loans in order to resell them to other banks at the optimal moment. The scope of machine learning: security, consumer behavior in stores, crime control, marketing, of course, politics (elections), education, psychology and human resource management.
In each subject area, upon closer examination, you can find problem statements for neural networks. Here is a list of individual areas where the solution of such problems is of practical importance now. Here are some of them.
In the financial area, neural networks predict exchange rates, the cost of raw materials (time series), help conduct automated trading on exchanges, predict the likelihood of bankruptcy, and determine the security of transactions with plastic cards. In the medical field, they make diagnoses, process images, monitor the condition of patients, analyze the effectiveness of the prescribed treatment. Neural networks recognize radar signals, adapt piloting of damaged aircraft, compress video information, optimize cellular networks, talk to us in the form of electronic assistants (Cortana, Siri), filter and block spam, help set up targeted advertising. In production processes, they are able to prevent emergencies and control product quality. In robotics - pave the routes for the movement of robots,
Significantly simplify the life of security and security systems - here neural networks are engaged in the identification of individuals by fingerprint, voice, signatures and individuals. For geologists, networks analyze seismic data and search for minerals using associative techniques.
How can medicine rely on unsubstantiated neural network solutions? But, perhaps the decisions made by man, by reason mixed with emotions, can always be considered absolutely correct for a particular case? There are, of course, the commission of doctors, but they will not always be able to collect. So in our not always evidence-based medicine, decisions are still made by people with specific training. The human factor, medical error against the likely false triggering of artificial intelligence. Maybe the fact is that in the case of the machine there is no concrete guilty? .. In nature, man has to look for answers, to justify and justify decisions in front of his own brain. It is always easier for a person when it is known who is to blame.
The same on the roads. It is unforgivable for a car to knock a person down, just as it is unforgivable for another person who has broken the rules to cause harm. Does the perpetrator always receive an objective punishment? Questions of morality will remain eternal. Probably, there is no one general answer. When self-driving cars from BMW or Google become commonplace on city streets, people will take the engine risk. And although in some cases computer driver will cause the death of a person, the total number of accidents and victims will decrease dramatically due to robots.
Most for ethicalneural networks are struggling companies that produce self-driving cars. Question: what should the autopilot do when in front of it two children play ball right at the intersection. Who should be in danger: children who are obviously breaking the rules (!) Or passengers of a car.
This example is reminiscent of the classic ethical task , in which you are the operator of a road arrow, on the same path a group of people, and on the other someone. The decision will always be unfair to the victim, his relatives and save the rest. Although people who survive at such a price may also not be happy.
Evolutionary brain resemblance
There is the notion of neurodarvinism. It includes a self-loading mechanism that works on the feedback between the environment and the brain. Even the simplest computer models of neural networks, if they are programmed to remove configurations that are unfavorable to their existence and reproduce beneficial ones, achieve amazing levels of complexity in a short time. This is what I mean; no structures in the real world are created for the purpose of self-destruction. Any creature is programmed to live. And neural networks too. Even such, in our opinion, a simple creature, like the fly Drosophila, has a complex system of connections in the brain . This is her brain you saw in the image at the beginning.
How the human brain works with images
As Rita Carter writes, in the book How the Brain Works, information-memories about the faces of people we know are stored in the brain as special neural networks (Face Recognition Units). When we see a new image, it is compared with our experience by scanning ERL. If there is something connecting, then that ERL becomes active and connects with the last seen image. The brain behaves in the same way, whether a new image is seen on the street or is generated independently by the consciousness of a person. The more often the consciousness accesses the stored images, the more actively the corresponding neural networks. Unnecessary networks disintegrate over time. This is what we call “completely forgotten.”
Consciousness
Why do I draw analogies with the human brain? Maybe it's not just to trust computer neural networks? Perhaps this is a matter of understanding and acceptance. Yes, another person, as a creature completely accepted by us as “our own” in contrast to a mechanical computer, will explain and justify any decision so that we will understand. And if he lies? And if he is mentally ill? There are nuances everywhere. Machines do not have consciousness, which means there are no ethical problems either - they are always more objective and impartial. But researchers cannot manage to leave them only for certain areas, such as creating the best chess combination, designing complex systems, and, therefore, we, people need to adapt too. Although it is difficult. Like to feel any line between mechanical and emotional. But such is the future
Thus, one more step towards understanding in a man-machine pair has been made. I want to quote the words of Roger Penrose, a professor of mathematics from the University of Oxford.
Understanding requires awareness. The illusion of understanding comes from the comprehensive processing of large amounts of data. Computation and understanding are complementary things.
“I believe that in order to explain understanding, we turn to new physical concepts with a quantum world, the mathematical structure of which is for the most part unknown.”
Penrose says that understanding generates a special component of brain tissue.
“There are microtubules in the human body, especially many in the nervous chambers.”
The scientist proposes to investigate whether it is possible that microtubules create stable quantum states that bind cellular activity throughout the brain, generating consciousness. Computer simulation of this state is impossible.