Google teaches robots to perform new tasks for themselves in the "kindergarten"

In kindergarten, robots learn to open doors. The
ability to learn is one of the most important for robots. If they start to learn, accumulating the information they need for themselves over time, then they can be used to perform complex tasks that were not pre-programmed. Tasks can be very different - from caring for the elderly and patients in hospitals to cleaning the premises. True, if you have to train each robot separately, it will take a huge amount of time. And what if robots teach robots? And what if groups of robots begin to learn together?
This problem is not new, it was not once described fiction. Specialists in robotics and artificial intelligence are also trying to resolve this issue. Google moreInterested in achieving learning from robots. Probably one of the easiest ways to achieve the desired is to create a common database of knowledge of robots, where information collected by each of the machines will be collected.
All robots must be associated with this base. If one robot learns something, everyone else will immediately gain knowledge and experience. Google employees tried out this idea (also not new) in practice, and got quite good results. In particular, the actions performed by one of the robots immediately became the property of his “colleagues”.
Robots can perform the same action very differently. Sometimes it works better, sometimes it gets worse. Any information about these actions is recorded and sent to the server, where it is processed using a neural network. The cognitive system evaluates the actions of each machine, and selects only information about positive experience, discarding data on unsuccessful attempts to perform one or another task. Robots load the data processed by the neural network with a certain frequency. And with each new download, they act more efficiently. In the video below, the robot is studying the process of opening the door.
After a few hours of training, the machine transmits information about its actions to the overall network. In the course of mastering the opening of the door, robots study the details of this procedure, gradually “understanding” what role the door handle plays and what needs to be done to open the door as quickly as possible.
The learning process by trial and error is good, but not perfect. People and animals, for example, can also analyze elements of the environment, assessing their possible influence on their actions. As they grow up, both in man and in animals a certain picture of the world is formed. It is clear that in humans it is much more complicated than in most animals, but there are similar elements in both cases.
Therefore, Google engineers decided to show robots how the laws of physics affect their actions. In one of the experiments, the robot was assigned to study various objects that are common to any home or office. These are pencils, pens, books and other items. The robots quickly learned and transmitted the received information to their “colleagues”. The entire team of robots in a short time received the concept of the consequences of their actions.
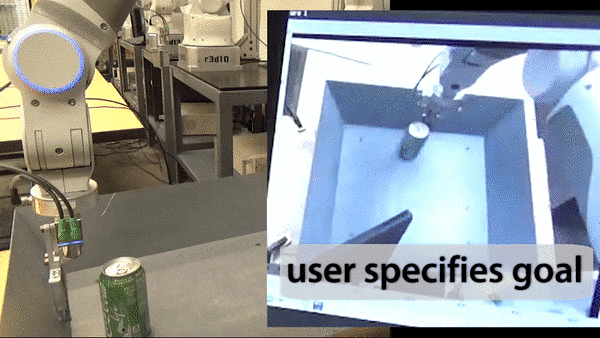
In the new experiment, engineers gave the command to the robot to move a specific object to a given point. However, the system did not receive any instructions about the nature of the object. Objects are constantly changing. It could be a bottle of water, a can of beer, a pen or a book. As it turned out, the task was completed by the robots, using data from previous experience of interaction with the real world. They were able to calculate the consequences of moving the object across the surface to the desired point.
And what about the man?
Two previous experiments were carried out with the participation of only robots, without human assistance. According to Google employees, the training of robotic systems can go much faster if a person helps the machine. After all, a person can quickly figure out what will happen as a result of performing some actions. For example, in one experience, a person helped different robots open doors of a different type. Each system received a unique door and lock.
As a result, a unified strategy was developed for all robots, which they called “politics”. All actions of the robots were processed using a deep neural network. She processed images from the cameras that recorded the actions of the robots, and transferred the already processed information to the central server in the form of a policy.
Robots consistently improved the “policy” using trial and error. Each robot tried to open the door, using the latest current policies. Robot actions were still processed by the neural network and uploaded to the server. Over time, robots began to work much more efficiently than the first time.
After the robots began to operate successfully, each of the instructors who worked with the robots changed the conditions of the problem somewhat. The changes were strong (the position of the door, the opening angle, etc.) changed, but they were sufficient to ensure that the previously developed policy was not entirely suitable for solving a new task. Robots gradually learned to cope with new conditions for themselves, and subsequently learned how to perform the most difficult tasks of opening various doors and locks. The final experiment showed the effectiveness of this type of training: robots were able to open the door and lock, which they had not yet encountered.
The authors of the project claim that the interaction of robots with each other and the central data repository helped them learn faster and more efficiently. And the use of a neural network has significantly improved the preliminary results.
Unfortunately, the list of tasks that can be performed by robots is extremely limited. Even the simplest movements and tasks like opening doors or raising various objects are difficult for them. The man still has to tell the robot what to do and how to act. But the algorithms are gradually improving, and neural networks have already ceased to be something amazing. Therefore, it is hoped that in the near future, robots will still be able to perform complex tasks. Maybe the future is already here.