Limitations of formal education, or why robots can't dance
- Transfer
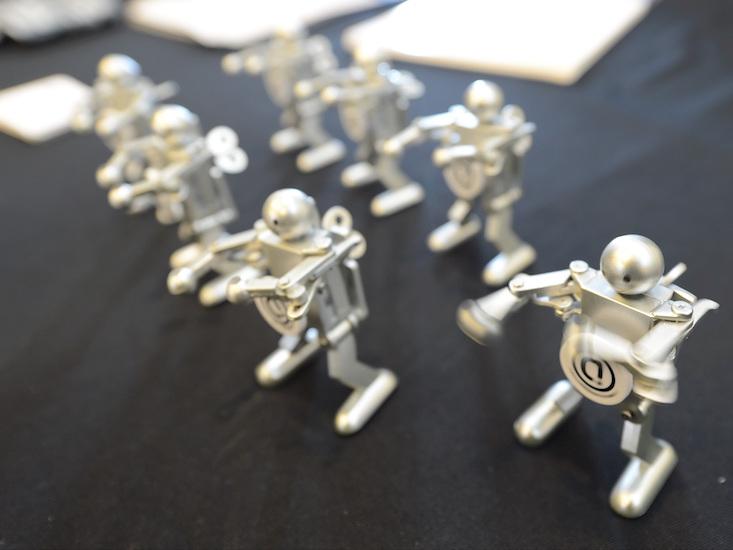
The 80s in the MIT computer science and artificial intelligence lab seemed to outsiders something of a golden age, but from the inside, David Chapman could see that winter was coming. As a member of the laboratory, Chapman became the first researcher to apply the mathematics of the theory of computational complexity to robotic planning, and also to show the absence of a real generalized method of creating an AI capable of creating a plan for handling all unforeseen circumstances. He concluded that although the AI of a person’s level can be possible in principle, none of the approaches available to us have any hope of achieving this level.
In 1990, Chapman wrote, a widely circulated subsequently, research proposal, which called for a new approach to testing and another task for AI:teach the robot to dance . Dance, as Chapman wrote, was an important model because “he does not achieve goals. You can not win or lose. This is not a task to be solved. Dance is a process of interaction. ” Dancing robots demanded a sharp change in the priorities of AI researchers, whose techniques were built around chess-type tasks, with a clear structure and unambiguous goals. The complexity of creating dancing robots required an even greater change in our assumptions about what intelligence is.
Chapman now writes about the practical applications of philosophy and cognitive science . Recently, in an interview with Nautilus magazine, he talked about the importance of imitation and apprenticeship, the limitations of formal rationality, and why robots are not preparing you breakfast.
What is interesting dancing robot?
Human learning is a social thing, tangible, and occurs in particular practical situations. You do not learn to dance by the book or the results of laboratory experiments. You learn by dancing with more experienced people.
Imitation and apprenticeship are basic ways of educating people. We forget this because classroom instructions have become important again in the last century, and more noticeable.
I decided to shift the focus of learning to development. “Learning” implies graduation — as soon as you learn something, you graduate. “Development” means an ongoing endless process. Dance does not do exams, after which you finish training.
It was a serious departure from the traditional approach to teaching AI, is not it?
Yes, in the first decades, AI researchers focused on those tasks that are especially associated with intelligence, because for people they are difficult: for example, chess. It turns out that for fairly fast computers, chess is simple. In the early works they ignored simple tasks for people: cooking breakfast, for example. Such simple tasks turned out to be difficult for computers controlling robots.
Early attempts at researching AI training also addressed formal problems, such as chess, in which bodies, social and practical contexts can be ignored. Recent studies show impressive progress in practical problems of the real world, such as pattern recognition. But there is still no success in the social and physical resources that are critical for human learning.
What can Heidegger teach us about intelligence and learning?
Formal rationality, used in science, engineering, mathematics, has provided many breakthroughs over the past few centuries. It is natural to take it for the essence of the intellect, and to assume that it lies at the basis of the functioning of man. For decades, analytic philosophers, cognitive psychologists, and AI researchers unconditionally accepted that people first create a rational plan with the help of logic, and then execute it. In the mid-1980s, it became obvious that for technical reasons it is usually just impossible.
Philosopher hubert dreyfus foresawthis deadlock is still ten years before its occurrence, in his book “What computers cannot do”. He proceeded from Heidegger's analysis of routine practical actions, such as the preparation of breakfast. Such physical skills do not seem to require formal rationality. Moreover, our ability to engage in formal reasoning depends on our ability to do practical, informal, and physical things — but not vice versa. Cognitive studies understood everything exactly the opposite! Heidegger suggested that most of life is like breakfast, and not like chess.
My colleague Phil Agre and I have developed new, interactive computational approaches to practical exercises that do not include formal reasoning, and have shown that they can be much more efficient than traditional logical paradigms. However, our systems need to be programmed manually, which seems impractical for tasks a little more complex than video games. The next step should be AI systems that develop skills without directly programming them.
Heidegger said little about learning, but his thought that human activities always have a social aspect was key. Phil and I were inspired by the schools of anthropology, sociology and social developmental psychology (some of which, in turn, were inspired by Heidegger). We began developing a computational theory of learning through apprenticeship. An article about dancing robots partially outlines our aspirations. Soon after, we realized that it was not yet possible to turn these ideas into working programs.
The construction of a physical robot carries with it many difficulties - for example, it is necessary that it does not fall - not having, at first glance, a direct relationship to learning and intelligence. Why not start creating a dancing robot with computer animation?
One of the difficulties of the rationalist approach to AI is that we cannot build an absolutely accurate model of the real world. He is very sloppy. A spoon of berry jam does not have a specific form. It is sticky, pliable, fluid. It is heterogeneous - partially grated berries behave differently from liquid parts. At the atomic level, it obeys the laws of physics, but to cook breakfast with them is impractical.
These are our bodies. Muscles are jelly bags interspersed with expanding filaments. The bones are irregular in shape, connected by elastic tendons, as a result of which the joints are amenable according to a complex pattern, approaching the limit of strength.
Using physical simulations, you can make an animated figure dance. She may seem very realistic. But these methods do not work with robots to perform the simplest human tasks. Dancing or cooking breakfast is still beyond the reach of modern science.
Physical simulations do not work well because the bodies of robots, like humans, are imperfect. Most modern developments are trying to tie robots to simple physical models, making them strong and solid, and as precisely manufactured as possible. But still, they show both flexibility, and limitations, and inconstancy, which is why they are difficult to control. They also have to be very heavy and powerful, which makes them dangerous and ineffective.
In the article “dancing robots,” I proposed to abandon this approach, and using machine learning, find ways to control light, weaker, and more flexible robots. Like a child, the system must gradually develop physical skills through experience. At that time, we did not have sufficient computer capacity, but some researchers have recently achieved success with this approach.
It seems that this topic is repeated in your work. We want a rigid and absolute world, but it is complex and heterogeneous.
Yes. My latest work on “ meanings ” offers to work in the interaction of uncertainty and patterns in order to improve understanding and actions. This is a “practical philosophy” for personal effectiveness, coming from the work that I did in the field of AI, and the academic fields that I mentioned earlier. She has a dimension for learning. Research into the development of adults shows that people can develop through pre-rational, rational, and meta-rational ways of understanding. The average condition is extremely hard. It has the idea that the world can be adjusted to the system. This approach can be clumsy, inefficient and unsustainable.
If the barrier that separates us from ideally accurate models is fundamental, not technological, will we need a completely different approach to AI?
The basic approach from the 1970s and 1980s has definitely failed, and for this very reason. " Deep Learning " which has achieved dramatic results, more flexible. It builds statistical and implicit models, instead of absolute and logical. However, it requires huge amounts of data, and people often learn from a single example. It will be very interesting to discover the scope and limitations of the deep learning approach.