Tekhnosfere five years
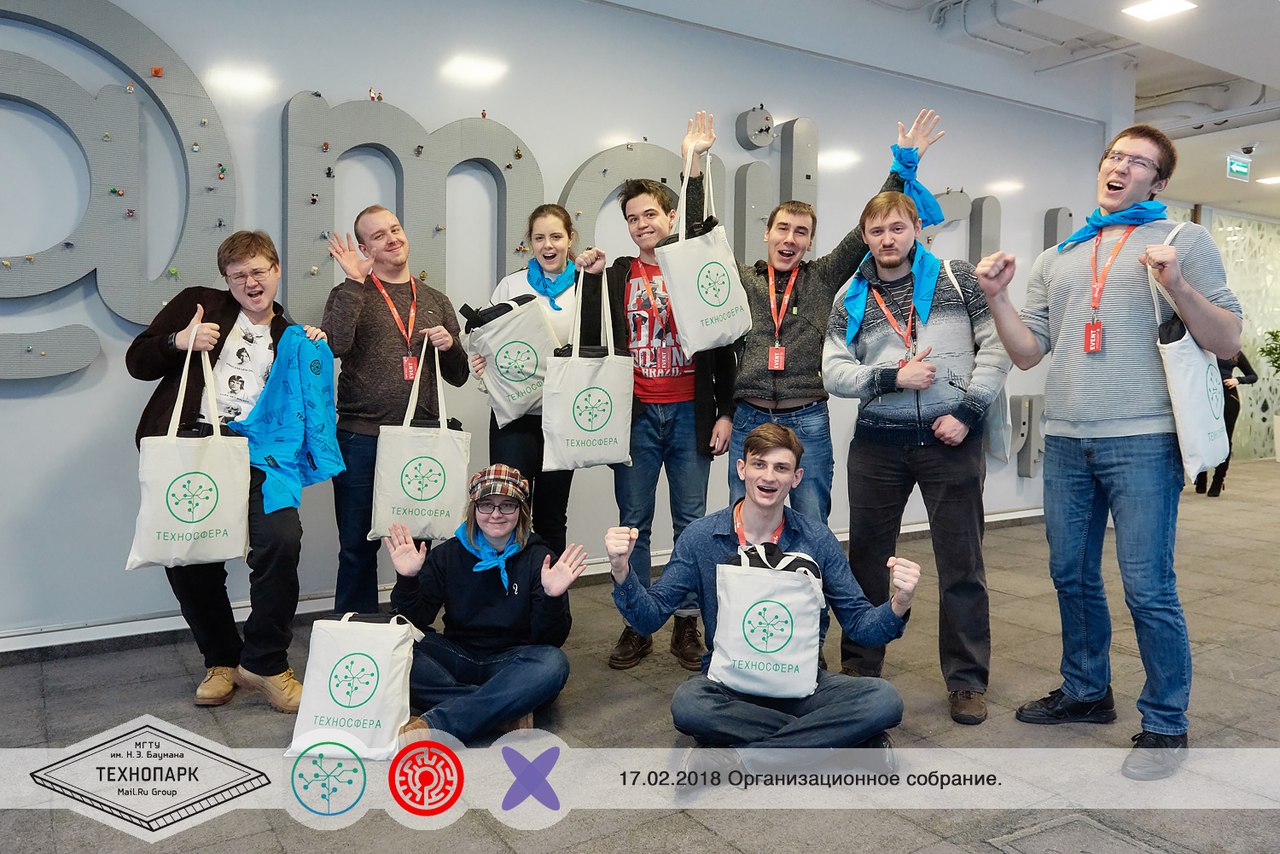
Today the Technosphere project celebrates its fifth anniversary. Here are our achievements over the years:
- Training completed 330 graduates.
- 120 students study at the course.
- Classes are 30 teachers.
- In the curriculum 250 lessons in 16 disciplines.
- Pupils perform 71 DZ.
- 8,000 users.
- More than 100 students began their careers at Mail.ru Group.
At the end of the course, students create their own graduation projects, for which they are given three months. And in honor of the fifth anniversary of the Technosphere, we have collected the brightest final works of recent years. Graduates themselves will tell about their projects.
"Bright Memory"
Vsevolod Vikulin, Boris Kopin, Denis Kuzmin
Initially, we planned to create a retouching service for images, which would also allow painting black and white photos. When discussing projects with mentors, an idea arose to tell the OK team about this idea, and as a result they decided to create a special application with a colorization function for BW photos of the war.
To do this, we had to design the neural network architecture, create a suitable set of photos for training the model and run the application on the OK platform.
We tried many ready-made neural networks, but none of them gave the desired quality. Then we decided to create our own. At the first stage, the neural network tried to predict the RGB image via the BW channel, but the result was so-so, because the network tried to paint everything in gray tones.
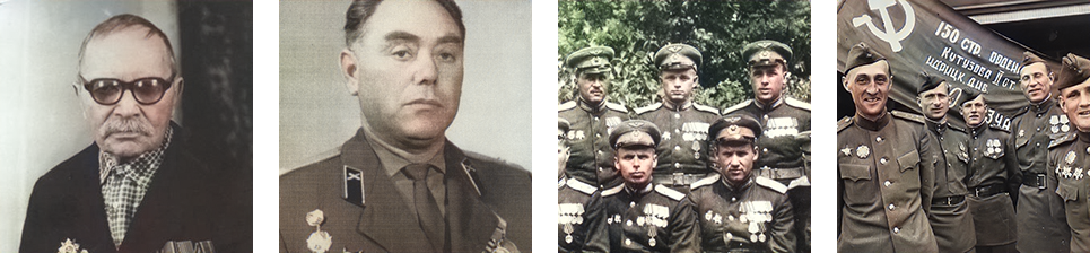
An example of the original neural network.
Then we decided to use the second pre-trained neural network.
With its help, we were able to extract signs from both the original color photo and the one that was painted with the first neural network. So we taught the second neural network to understand what colors are inherent in certain objects in real life: the sky is blue, the grass is green, and so on. To implement neural networks, we used the popular framework Pytorch.
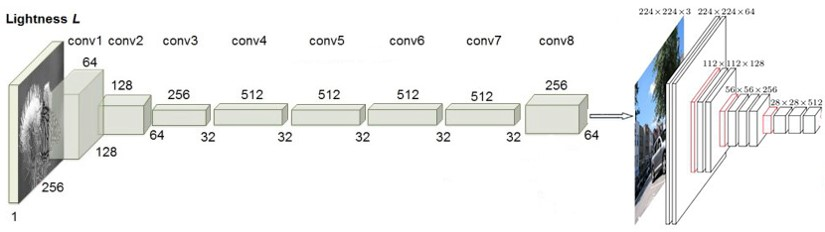
New neural network architecture.
But most importantly, it was necessary to teach the model as realistic as possible to paint the faces of people. We were faced with the fact that among the existing data sets there were no suitable ones for our task - we needed large photos of people with some natural background. To form our own set of images, we first built a list of 5,000 celebrity names. Then these names were searched for pictures in various search engines. With the help of facial recognition techniques, we screen out images that do not contain faces at all, and the remaining photographs selected the most appropriate fragments. So we collected the necessary set of 600 thousand photos.
Then came the task of realistic coloring military uniforms.
To solve it, we had to artificially generate military uniforms with various medals and orders. In addition, I had to frame some color films about the war.
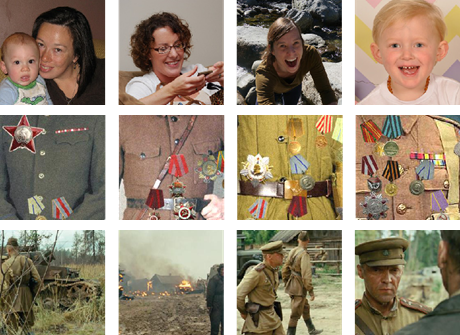
Examples of photos from the training set.
Combining all this with a popular set of general photographs, we received 2.5 million photographs for training the neural network.
We prepared a working prototype of the neural network and started developing an application on the OK platform. This is a standard web application with backend and front end. We were responsible for the backend, and the frontend was taken over by the OK team. Really appreciating the available resources, we decided that it would be more rational to use the current architecture of the Artisto project.
To do this, we ported the neural network code into the Lua Torch framework and implemented it in the environment.
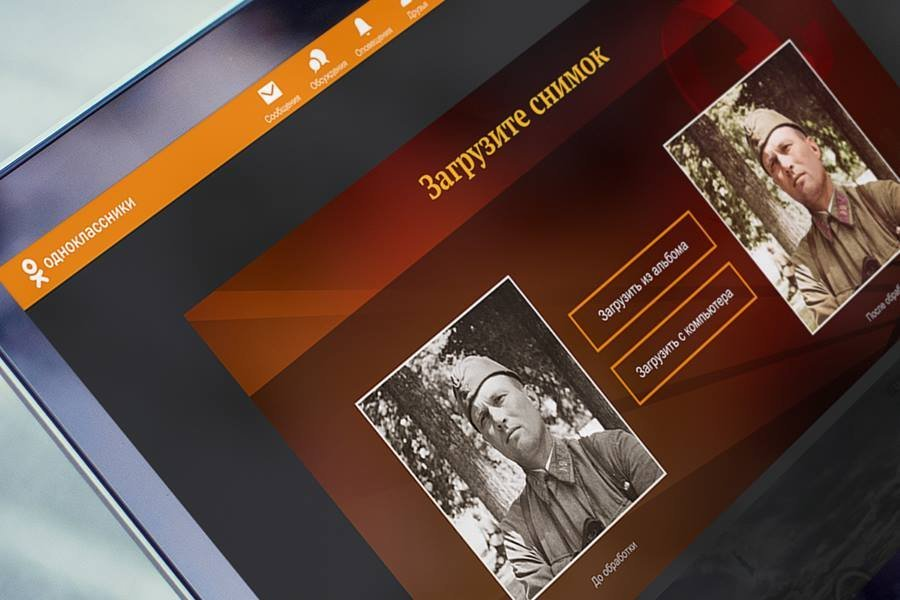
Application interface in OK.
On May 9, our application became available to Odnoklassniki’s multi-million dollar audience, several major media outlets wrote about it, and currently 230 thousand people use the service. It was very hard to implement the project in such a short time, but we coped with everything. Many thanks to our mentors Olga Schubert and Alexei Voropayev, who helped us with integration into the OC. We also thank the infrastructure development team from Mail.ru Search for help with the integration into the Artisto project, and Dmitry Solovyov separately for invaluable advice on the neural network architecture.
"Music Map"
Vladimir Bugaevsky, Dana Zlochevskaya, Ralina Shavalieva
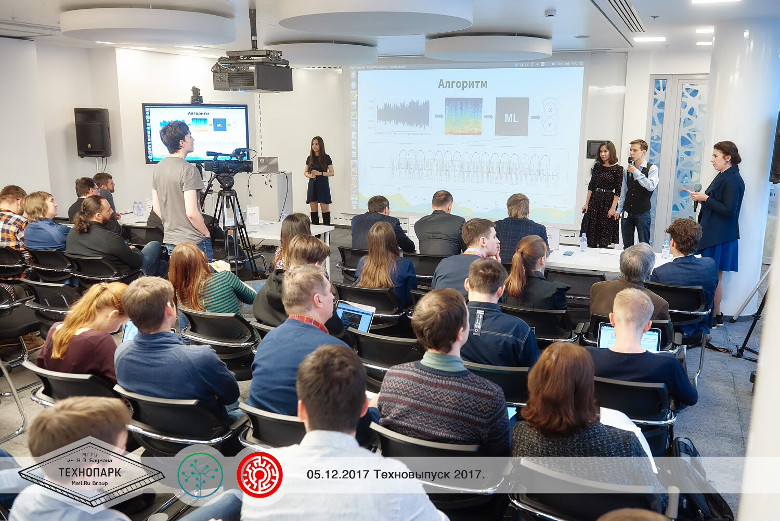
The mentor Alexey Voropaev and Dmitry Solovyov prompted us to the idea of the project. Once there was a Sony player who could classify songs according to four moods. Today, technology has made great strides forward, artificial intelligence and neural networks are actively developing, and we realized that we could do something cooler that our users would like - a music card that would visualize the mood of VK's audio records. And they decided to implement it as an extension for Chrome - it is easy to install and convenient to use.
Naturally, we began by studying the approaches that were already used to determine the mood of music. Looking at about a dozen scientific articles, we realized that almost no one tried to use neural networks to analyze the emotions of audio recordings.
Another challenge for us was the task of visualizing emotions. It turned out that in psychology there are many models of the representation of human moods, each of which has its own advantages and disadvantages. We stopped at the so-called circmplex spatial model: its idea is that any emotion can be represented as a point in two-dimensional space. Thanks to this scale, we were able to visualize the mood of his audio recordings in a manner that is understandable to the user.
We have identified three fronts of work on the application:
- The server part will receive expansion requests, build spectrograms, make forecasts and return them to the user.
- The user part with which the person will interact.
- Neural network training: training set preparation, choice of network architecture and the learning process itself.
The scope of work was extremely large, so everyone could try himself in everything. Our team acted very smoothly: we constantly came up with different ways to solve various tasks and helped each other to understand the features of the implementation of individual parts. The main difficulty we encountered was an extremely short time frame - three months. During this time, we had to figure out from scratch in the development of the frontend (learn to write in JavaScript), in the intricacies of the neural network learning framework (PyTorch) and master the technologies of modular development (Docker). Now our application is working in test mode for several users.
“Video Colorization for Professionals”
Yuri Admiralsky, Denis Bibik, Anton Bogovskiy, Georgy Kasparyants
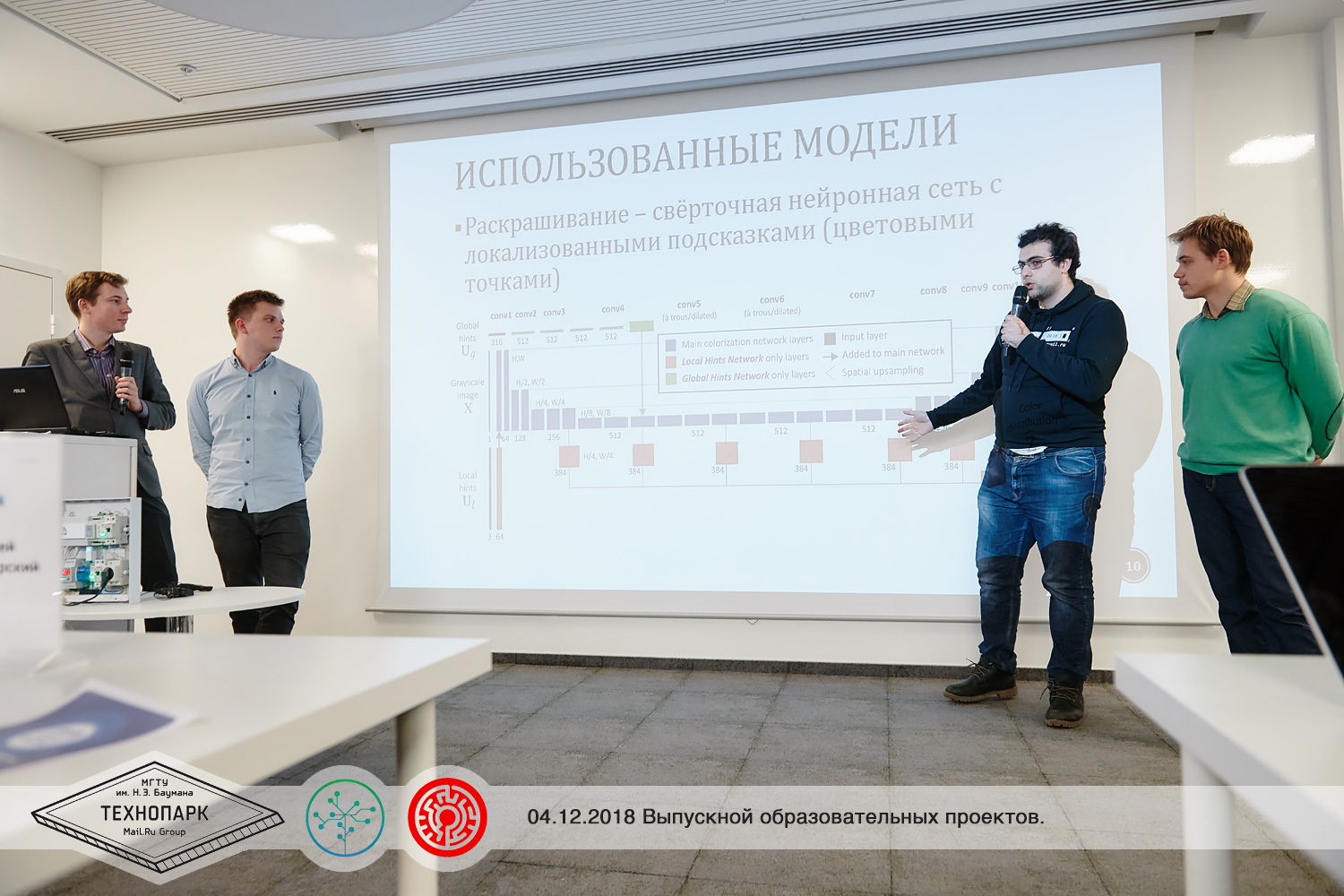
The idea of the project arose as a result of the analysis of current trends in the development of neural networks for solving problems of computer graphics and processing multimedia content. In this area, several different approaches to coloring individual images have already been proposed, such a problem arises, for example, when processing old archival photos. On the other hand, the success of painted versions of black and white Soviet films showed the relevance of video coloring tasks. Coloring video by hand, frame by frame, is an extremely time-consuming task, the solution of which requires the involvement of professional studios. And of the users who want to get the color versions of their old videos, few people possess the necessary skills and have enough time for hand coloring, not to mention the money to perform such a task with the help of professional teams of video studios. Therefore, we decided to try to apply known approaches to coloring and create an editor program to significantly reduce the complexity of coloring video recordings using neural networks.
The main task that had to be solved when developing such a program was to get the correct colors when coloring objects in the frame. We are faced with the fact that classical data sets (for example, ImageNet) used in training neural networks for solving image processing problems do not allow us to achieve a good automatic (without any additional information) coloring. For example, some objects in the frame were not recognized and remained black and white on the painted image. Another problem of state-of-the-art models was the wrong choice of colors for painting objects — both because of the task’s underdetermination (clothing coloring) and as a result of the incorrect definition of rare objects, as well as objects affected by compression artifacts.
To solve this problem, we applied the method of local color prompts , which, by specifying the color of individual points of objects, allowed us to achieve the correct assignment of colors for the entire object and correct color transitions. In this case, the neural network during coloring controls the observance of object boundaries and brightness transitions. This approach made it possible to reduce the laboriousness of coloring individual frames (it was necessary to explicitly specify the colors of only individual points on the frame, without using brushes), and helped solve the problem of underdeterminations and color changes when switching from frame to frame. In addition, we have implemented models that allow us to track the movement of objects in the frame and move the color hints. With the help of our editor, we painted a fragment of the old black and white film The Kid.
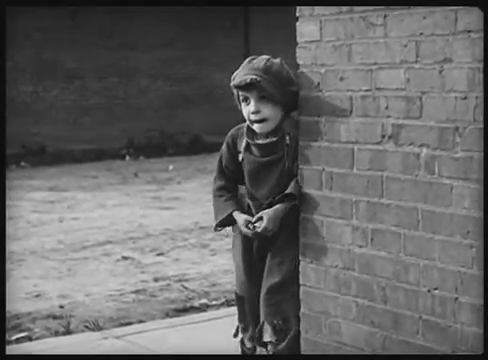
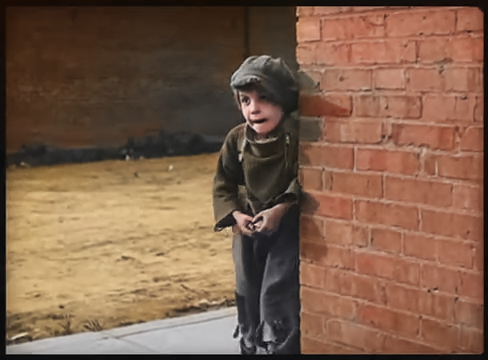
An example of a painted frame from the film Chaplin The Kid (1921).
We implemented the editor as a client standalone application, in which the video is loaded, and then using color hints, frames are laid out. You can calculate colorization models on a local machine, or on third-party computing power (for example, by deploying the server part in the cloud) in order to process video more quickly.
To create the editor, we have done a lot of work, including testing and refining coloring models and tracking objects of the frame, developing the client-server architecture of the application and developing the usability of the client application. We studied the subtleties of working with the PyTorch framework that implements the work of neural networks, mastered the Qt 5 framework for developing a client application, learned how to use Django-REST and Docker for developing and deploying a computing backend.
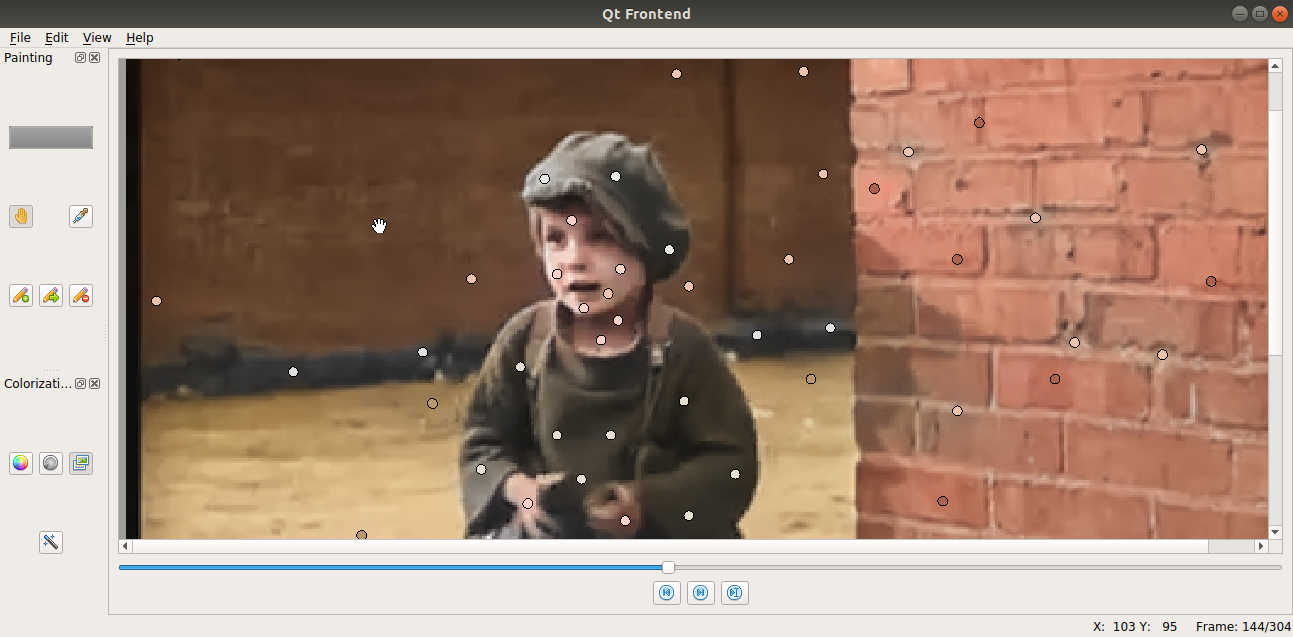
An example of the client application.
Thanks to the teachers of Tekhnosfera for their dedicated work, for the actual knowledge that you give to students. We wish the project to grow and develop!
* * *
You can apply for training until 10:00 on February 16 at sphere.mail.ru. Please note that only students and graduate students of Moscow State University can be trained in the Technosphere. Mv Lomonosov.