IT Service Management (ITSM) Made More Efficient With Machine Learning Tools
- Transfer
- Recovery mode
In 2018, we firmly consolidated our positions - IT services management services (ITSM) and IT services still continue to operate, despite ongoing talk about how long they will last in the digital revolution. Indeed, the demand for technical support services is growing - the Technical Support Report and the HDI Salary Report (Help Desk Institute) for 2017 indicate that 55% of technical support services noted an increase in the volume of applications over the past year.
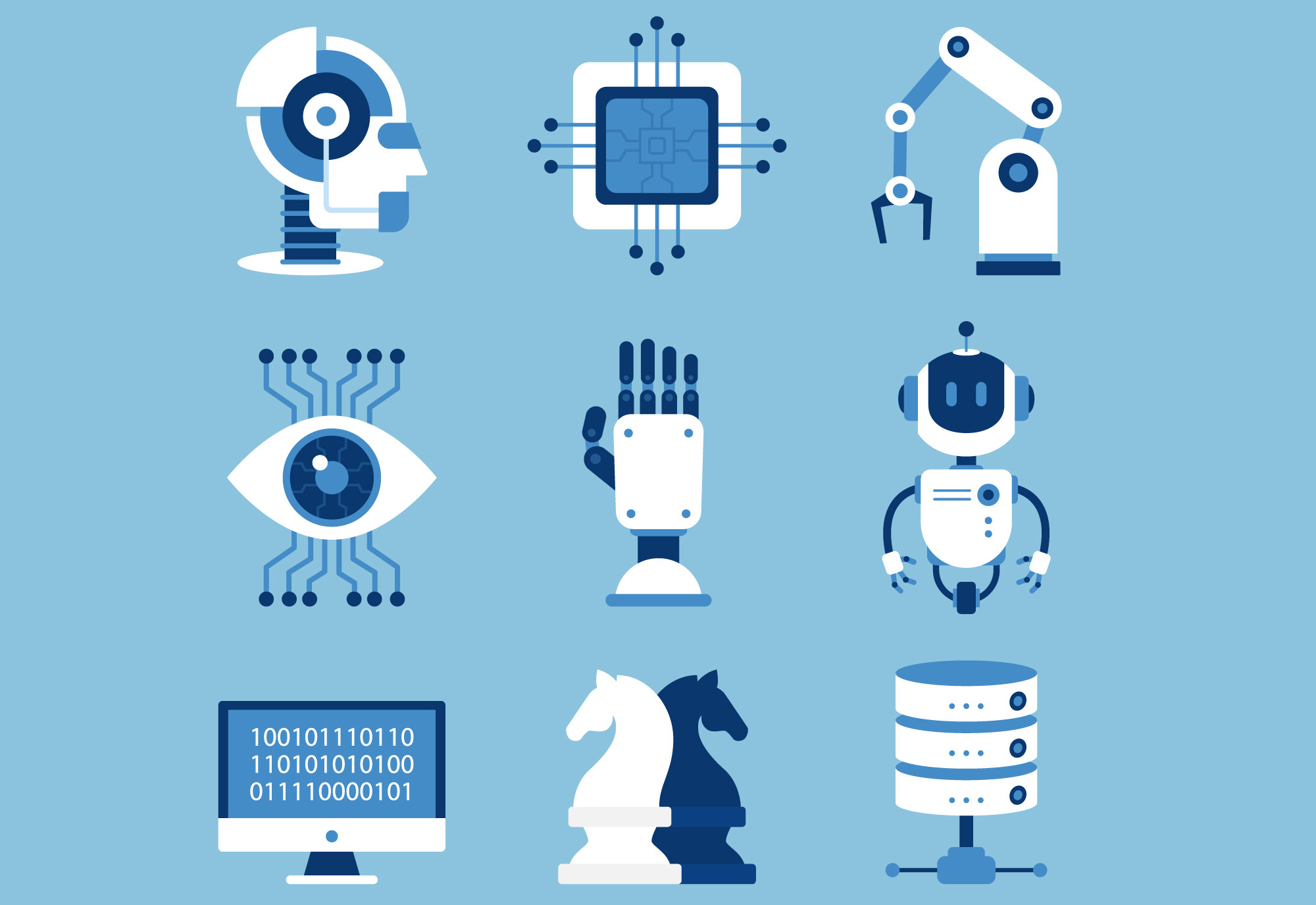
On the other hand, many companies noted a decrease in the volume of technical support calls last year (15%) compared to 2016 (10%). A key factor contributing to a decrease in the number of applications was independent technical support. However, HDI also reports that last year the application cost rose to $ 25, compared to $ 18 in 2016. This is not what most IT services aim for. Fortunately, automation based on analytics and machine learning can improve support processes and productivity by reducing errors and improving quality and speed. Sometimes this goes beyond human capabilities, and machine learning and analytics are a key foundation for an intelligent, capable, and operational IT support service.
This article looks in more detail at how machine learning can solve many support and ITSM problems associated with the volume and cost of applications, and how to create a faster and more automated support service that employees will enjoy using.
My favorite definition of machine learning is MathWorks :
“Machine learning teaches computers how to do what is natural for humans and animals to learn from their own experience. Machine learning algorithms use computational methods to study information directly from data, without relying on a predefined equation as a model. The algorithms adaptively improve their own efficiency as the number of samples available for study increases. ”
The following options are available for some ITSM tools based on machine learning and Big Data analytics:
Ultimately, machine learning and analytics transform ITSM systems with intelligent assumptions and recommendations about application problems and the change process that help agents and IT support groups describe, diagnose, predict and prescribe what happened, what happens and what happens. End users receive proactive, personal, and dynamic analytic evaluations and quick solutions. However, much is done automatically, i.e. without human intervention. And as technology learns over time, processes only get better. It is important to note that all the smart features described in this article are available today.
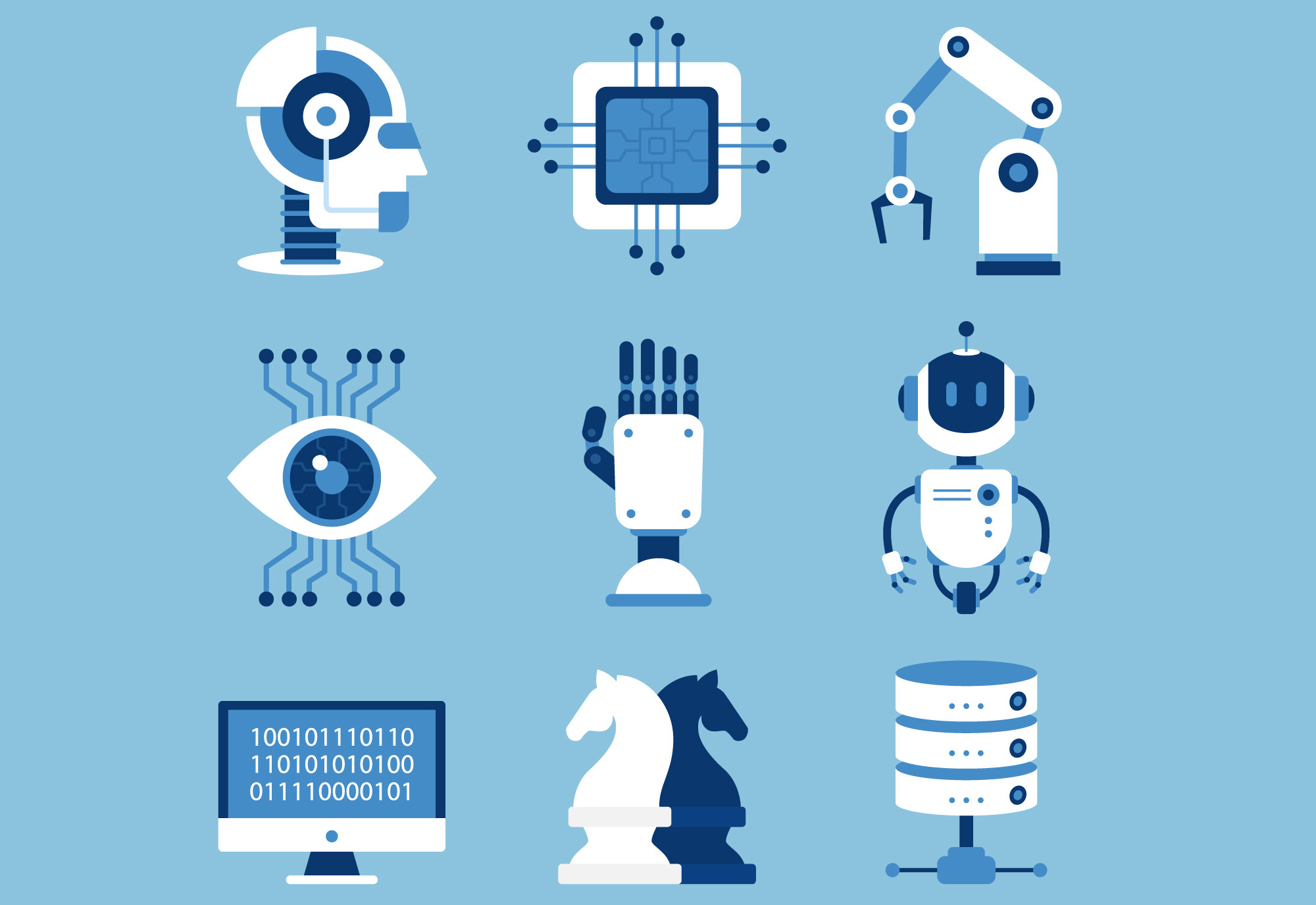
On the other hand, many companies noted a decrease in the volume of technical support calls last year (15%) compared to 2016 (10%). A key factor contributing to a decrease in the number of applications was independent technical support. However, HDI also reports that last year the application cost rose to $ 25, compared to $ 18 in 2016. This is not what most IT services aim for. Fortunately, automation based on analytics and machine learning can improve support processes and productivity by reducing errors and improving quality and speed. Sometimes this goes beyond human capabilities, and machine learning and analytics are a key foundation for an intelligent, capable, and operational IT support service.
This article looks in more detail at how machine learning can solve many support and ITSM problems associated with the volume and cost of applications, and how to create a faster and more automated support service that employees will enjoy using.
Effective ITSM through machine learning and analytics
My favorite definition of machine learning is MathWorks :
“Machine learning teaches computers how to do what is natural for humans and animals to learn from their own experience. Machine learning algorithms use computational methods to study information directly from data, without relying on a predefined equation as a model. The algorithms adaptively improve their own efficiency as the number of samples available for study increases. ”
The following options are available for some ITSM tools based on machine learning and Big Data analytics:
- Support through the bot. Virtual agents and chatbots can automatically offer news, articles, services and support offers from data directories and public requests. Such 24/7 support in the form of end-user training programs offered helps solve issues much faster. The key advantages of the bot are an improved user interface and fewer incoming calls.
- Smart news and notifications. These tools let you notify users in advance of potential problems. In addition, IT professionals can recommend workarounds for resolving problems with personalized notifications that provide end-users with up-to-date and useful information about problems they may encounter, as well as tips on how to avoid them. Informed users will appreciate the active IT support, and the number of incoming calls will decrease.
- Smart search.When end users seek information or services, a context-sensitive knowledge management system can provide recommendations, articles, and links. End users usually skip some of the results, preferring others over them. These clicks and the number of views are included in the “weighting” criteria when re-indexing content over time, so the search capabilities are dynamically tuned. Since end users provide feedback in the form of a “like / dislike” vote, this also affects the rating of the content that they and other users can find. In terms of benefits, end users can quickly find answers and feel quite confident
- Analytics of popular topics.Here, analytic capabilities reveal patterns for structured and unstructured data sources. Information about popular topics is graphically displayed in the form of a heatmap, where the size of the segments corresponds to the frequency of certain topics or groups of keywords requested by users. Recurring incidents will be detected instantly, grouped and resolved together. Analytics of popular topics also detects incident clusters with a common root cause and significantly reduces the time to identify and solve the main problem. The technology can also automatically create knowledge base articles based on similar interactions or similar problems. Finding trends in any data increases the activity of the IT department, prevents the recurrence of incidents and, therefore,
- Smart application.End users expect that submitting an application is no more difficult than writing a tweet, namely a short message in natural language describing the problem or request that can be sent by email. Or even just attach a photo of the problem and send it from your mobile device. Registering a smart application speeds up the process of creating a call by automatically filling in all the fields based on what the end user wrote or the scan of the image processed using the optical character recognition (OCR) program. Using a set of observational data, the technology automatically classifies and addresses requests to the appropriate support agents. Agents can redirect requests to various support groups and can overwrite automatically filled fields, if the machine learning model is not optimal for this case. The system learns from new templates, which allows you to better cope with emerging problems in the future. All this means that end users can easily and quickly open applications, which leads to increased satisfaction when using work tools. This feature also reduces manual work and errors and helps reduce time and resolution costs.
- Smart email. This tool resembles a smart application. The end user can send a letter to support and describe the problem in a natural language. The support service tool creates an application based on the contents of the email, and also automatically responds to the end user with links to the proposed solutions. End users are satisfied because opening applications and inquiries is easy and convenient, and IT agents have less manual work.
- Smart change management.Machine learning also supports modern analytics and change management. Considering the frequent number of changes that enterprises today require, intelligent systems can provide agents or change managers with proposals aimed at optimizing the environment and increasing the percentage of successful changes in the future. Agents can describe the necessary changes in a natural language, and analytic capabilities will check the content for the presence of affected configuration elements. All changes are regulated, and automatic indicators inform the change manager if there are any problems with the change, such as risk, planning in an unplanned window or the status is “not approved”. A key benefit of smart change management is faster payback times with fewer configurations.
Ultimately, machine learning and analytics transform ITSM systems with intelligent assumptions and recommendations about application problems and the change process that help agents and IT support groups describe, diagnose, predict and prescribe what happened, what happens and what happens. End users receive proactive, personal, and dynamic analytic evaluations and quick solutions. However, much is done automatically, i.e. without human intervention. And as technology learns over time, processes only get better. It is important to note that all the smart features described in this article are available today.