
The state space in the problems of designing optimal control systems
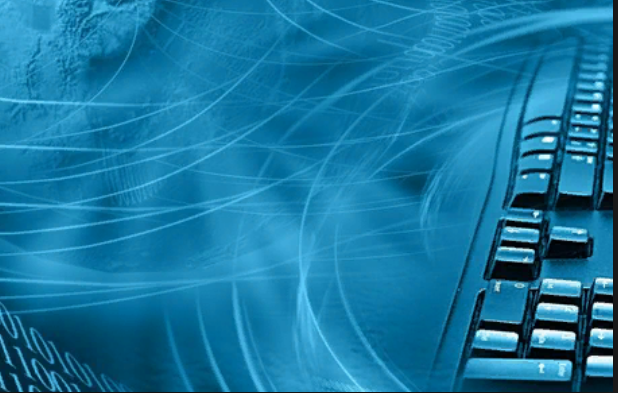
Introduction
The study of the control system in the time domain using state variables has been widely used recently due to the simplicity of the analysis.
The state of the system corresponds to a point in a certain Euclidean space, and the behavior of the system in time is characterized by the trajectory described by this point.
At the same time, the mathematical apparatus includes ready-made solutions for analog and discrete LQR and DLQR controllers, a Kalman filter, and all this with the use of matrices and vectors, which allows us to write down the equations of the control system in a generalized form, receiving additional information when solving them.
The purpose of this publication is to consider solving the problems of designing optimal control systems by describing the state space using Python software.
Theory briefly
The vector-matrix recording of the linear dynamic object model, taking into account the measurement equation, takes the form:

If the matrices A (t), B (t) and C (t) are time-independent, then the object is called an object with constant coefficients, or a stationary object . Otherwise, the object will be unsteady.
If there are errors in the measurement, the output (adjustable) signals are given by the linearized matrix equation:

where y (t) is the vector of adjustable (measured) values; C (t) is the matrix of the relation between the measurement vector and the state vector; v (t) is the vector of measurement errors (interference).
The structure of a linear continuous system that implements equations (1) and (2) is shown in the figure:

This structure corresponds to the mathematical model of an object constructed in the state space of its input x (t), u (t), output y (t) and internal, or phase coordinates x (t).
For example, consider a mathematical model of a DC motor with independent excitation from permanent magnets. The system of equations of the electric and mechanical parts of the engine for the case under consideration will look like this:
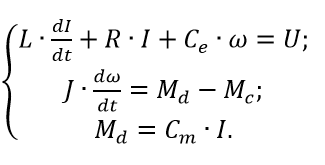
The first equation reflects the relationship between the variables in the armature chain, the second - the conditions of mechanical equilibrium. As generalized coordinates, we choose the armature current I and the frequency of rotation of the armature ω .
The control is the voltage at the armature U , the disturbance is the load resistance moment Mc. The model parameters are the active resistance and inductance of the circuit and the armature, respectively designated Rя , and Ля , as well as the reduced moment of inertia J and the structural constants ce and cm (in the SI system: Ce = Sm ).
Solving the original system with respect to the first derivatives, we obtain the equations of the engine in the state space.

In the matrix form, equations (4) will take the form (1):
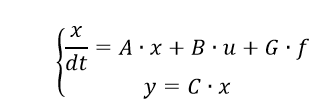
where is the vector of generalized coordinates


If we choose the rotation frequency as an adjustable quantity, then the measurement equation will be written in the form:

and the measurement matrix will take the form:
C = (0 1)
Let's form the engine model in Python. To do this, we first set the specific values of the engine parameters: U = 110 V; R = 0.2 ohms; L = 0.006 H; J = 0.1 kg / m2; Ce = Cm = 1.3 V / C and find the values by the coefficient of the matrix of the object from (6).
Development of a program forming an engine model with matrix testing for observability and controllability:
When developing the program, a special function def matrix_rank was used to determine the rank of the matrix and the functions shown in the table:

# -*- coding: utf8 -*-
from numpy import*
from control.matlab import *
from numpy.linalg import svd
from numpy import sum,where
import matplotlib.pyplot as plt
def matrix_rank(a, tol=1e-8):
s = svd(a, compute_uv=0)
return sum( where( s>tol, 1, 0) )
u=110 # Напряжение якоря
J=.1 # Момент инерции
c=1.3; # Конструктивный коэффициент
R=.2; L=.006 # Активное сопротивление и индуктивность якоря
A=matrix([[-R/L ,-c/L],[ c/J ,0]])
print ('Матрица А : \n %s'%A)
B=matrix([[1/L],[0]])
print ('Матрица B : \n %s '%B)
C=matrix([[0, 1]])
print ('Матрица C : \n %s '%C)
D=0
print ('Скаляр D : \n %s '%D)
sd=ss(A,B,C,D) #Задание модели объекта в пространстве состояний
wd=tf(sd) # Задание передаточной функции двигателя
print ('Передаточная функция двигателя : \n %s '%wd)
a=ctrb(A,B)
print(' Ранг матрицы управляемости : %s'%matrix_rank(a, tol=1e-8))
a=obsv(A,C)
print('Ранг матрицы наблюдаемости: %s'%matrix_rank(a, tol=1e-8))
y,x=step(wd) # Построение переходной характеристики
plt.plot(x,y,"b")
plt.title('Переходная характеристика двигателя ')
plt.ylabel('Частота вращения вала в рад/с')
plt.xlabel('Время С')
plt.grid(True)
plt.show()
The results of the program:
Matrix A:
[[-33.33333333 -216.66666667]
[13. 0.]]
Matrix B:
[[166.66666667]
[0.]]
Matrix C:
[[0 1]]
Scalar D:
0
Engine transfer function:
2167 / (s ^ 2 + 33.33 s + 2817)
Rank of controllability matrix: 2
Rank of observability matrix: 2

Conclusions:
1. Using a direct current motor with independent magnetic excitation as an example, we consider a control design technique in a state space;
2. As a result of the program, the transfer function, the transition characteristic, as well as the ranks of the matrices of controllability and observability are obtained. The ranks coincide with the dimensions of the state space, which confirms the controllability and observability of the model.
An example of designing an optimal control system with a discrete dlqr controller and full feedback
Definitions and terminology
Linear quadratic regulator (LQR) is one of the types of optimal controllers in control theory that uses the quadratic quality functional.
The task in which the system is described by linear differential equations, and the quality indicator is a quadratic functional, is called the linear-quadratic control problem.
Linear-quadratic controllers (LQR) and linear-quadratic Gaussian controllers (LQG) are widely used.
When starting a practical solution to a problem, you always need to remember the limitations
To synthesize an optimal discrete controller of linear stationary systems, you need a function to numerically solve the Bellman equation. There is no such function in the Python Control Systems library [1], but you can use the function to solve the Riccati equation given in [2]:
def dlqr(A,B,Q,R):
"""Solve the discrete time lqr controller.
x[k+1] = A x[k] + B u[k]
cost = sum x[k].T*Q*x[k] + u[k].T*R*u[k]
"""
#ref Bertsekas, p.151
#first, try to solve the ricatti equation
X = np.matrix(scipy.linalg.solve_discrete_are(A, B, Q, R))
#compute the LQR gain
K = np.matrix(scipy.linalg.inv(B.T*X*B+R)*(B.T*X*A))
eigVals, eigVecs = scipy.linalg.eig(A-B*K)
return K, X, eigVals
But we must also take into account the restrictions on the synthesis of the optimal controller given in [3]:
- the system defined by the matrices (A, B) must be stabilizable;
- inequalities S> 0, Q - N / R – NT> 0 must be satisfied, a pair of matrices (Q - N / R – NT,
A - B / R – BT) should not have observable modes with eigenvalues on the
real axis.
After digging into an extensive and ambiguous theory, which, for obvious reasons, I do not give, the problem was solved, and all the answers can be read directly in the comments to the code.
The block diagram of the control system controller with feedback on all state variables is shown in the figure:

For each initial state x0, the optimal linear controller generates the optimal program control u * (x, k) and the optimal trajectory x * (k).
The program forming the optimal control model with dlqr controller
from numpy import *
import scipy.linalg
import matplotlib.pyplot as plt
def dlqr(A,B,Q,R):
#Дискретное решение матричного уравнения Риккати x[k+1] = A x[k] + B u[k]
P= matrix(scipy.linalg.solve_discrete_are(A, B, Q, R))
#Дискретный контроллер DLQR
K = matrix(scipy.linalg.inv(B.T*P*B+R)*(B.T*P*A))
E, E1 = scipy.linalg.eig(A-B*K)
return K, P, E
"""Параметры системы"""
A=matrix([[1, 0],[ -2 ,1]])
B=matrix([[1, 0],[1, 0]]).T
"""Параметры качества управления"""
Q=matrix([[0.5, 0],[0, 0.5]])
R=matrix([[0.5,0],[0, 0.5]])
T =100# Время регулирования
SS=0.5# Величина шаг
N =int(T/SS)# Количество шагов
K, P, E=dlqr(A,B,Q,R)#Вычисление параметров контроллера
print("K= \n%s"%K)
print("P= \n%s"%P)
print("E= \n%s"%E)
x = zeros([2, N])
u= zeros([2, N-1])
x [0,0]=2
x [1,0]=1
for i in arange(0,N-2):
u[:, i]= dot(- K,x[:, i])
x[:, i+1]=dot(A,x[:, i])+dot(B,u[:, i])
x1= x[0,:]
x2= x[1,:]
t = arange(0,T,SS)
t1=arange(0.5,T,SS)
plt.subplot(221)
plt.plot(t, x1, 'b')
plt.grid(True)
plt.subplot(222)
plt.plot(t, x2, 'g')
plt.grid(True)
plt.subplot(223)
plt.plot(t1, u[0,:], 'y')
plt.grid(True)
plt.subplot(224)
plt.plot(t1, u[1,:], 'r')
plt.grid(True)
plt.show()
Calculation results:
K =
[[0.82287566 -0.17712434]
[0.82287566 -0.17712434]]
P =
[[3.73431348 -1.41143783]
[-1.41143783 1.16143783]]
E =
[0.17712434 + 0.17712434j 0.17712434-0.17712434j] x State
dynamics, and x2, u1, u2.
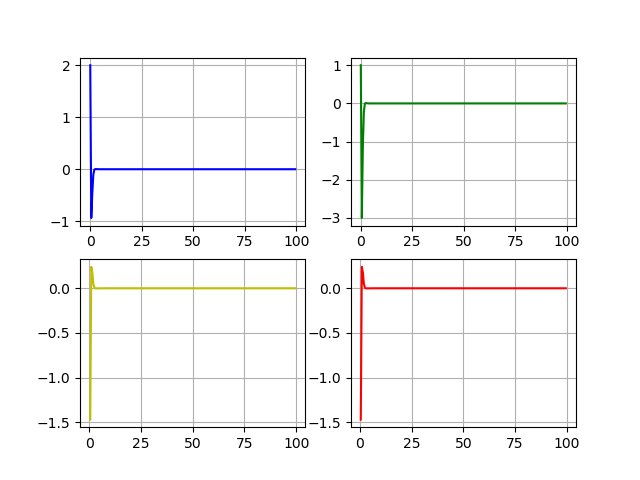
Conclusion
Separate optimal control problems, as described, can be solved using Python, combining the capabilities of the Python Control Systems, SciPy, NumPy libraries, which, of course, contributes to the popularization of Python, given that previously such problems could only be solved in paid mathematical packages.