Single-channel voice splitter: on the way to the product (preview)
Voice, sound, sound wave analysis: acoustics is one of the most interesting and complex data collection channels in the multimodal logic of detecting and recognizing human emotions. Among other things, referring to this source of information poses the researchers different tasks, the solution of which opens up new scientific and technological prospects. We at the Neurodata Lab , dealing with the topic of emotions, managed to deal with the fundamental problem along the way: single-channel separation of votes, reaching accuracy exceeding 91-93%, for English, Russian and some other key languages (experiments are continued on them, the first two are given priority).
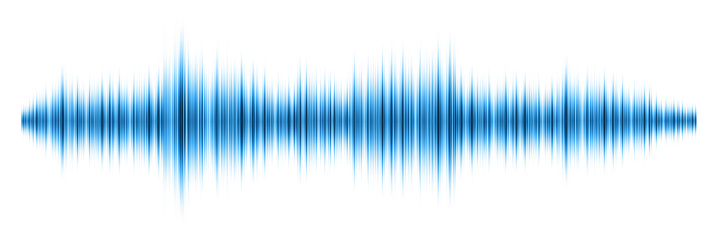
Of course, at the moment we are at the stage of preparing a full-fledged article, as well as assembling and packaging a future commercial product, so here we only briefly outline our activities in this area with an invitation to discuss the results after their publication and presentation at conferences in the first half of 2018.
So, what do we have as of today. A working prototype of a system designed to solve the following tasks under the following conditions:
The technological basis of the solution are three subsystems:
The phrase in this context refers to a continuous section of speech between two micro-pauses. The concept is inaccurate, conditional, the result of the use of the phrase extractor strongly depends on the pronunciation features (jerky or “smoothed”, continuous speech), on the parameters of “micropause”, etc. Typical settings of the phrase extractor lead to the fact that the phrase, as a rule, is a sequence of phonemes, syllables, sometimes words lasting from 0.2 to several seconds. The exact settings of the phrase identifier will be given in its technical description.
The meaning of using the phrase extractor is as follows. If we remove from the speech the moments of simultaneous sounding of two voices, then the remaining record will be alternating (without overlapping) sections of one-voice speech, while in most cases the speaker will change at the border of phrases.
This assumption is not entirely true, in practice there is a pause-free transition of speech from one speaker to another. However, these cases are indeed rare, and in the proposed prototype, the main negative effect of such pause-free transitions is reduced to the incorrect formation of basic support fragments of the voices of two people and partially stopped by the principle of the formation of such support fragments.
Thus, modulo the absence of phrases with the transition of voices, further work (after highlighting phrases and throwing out moments of simultaneous speech) is reduced to the task of voice identification of phrases.
In addition to the original function (we only need fragments of one-voice speech), the detector allows you to leave only those phrases (or parts of them) where one voice sounds (modulo phrases with voice transition, which was higher), thereby reducing the problem to the problem of voice identification .
The operation of the simultaneous speech detector is based on visual observation: the log spectrogram or its time derivative in the areas of simultaneous speech contains characteristic irregularities that are absent in single-voice areas and are easily distinguishable by the eye. Examples will be given in the detector description.
In connection with this observation, the solution is based on 2D convolution networks, which are designed to highlight graphic features. However, the current prototype contains additional, 1D-convolutional neural network solutions to improve the quality of detection.
The idea underlying the detector turned out to be quite successful in the sense that not only moments of simultaneous speech are determined, but, as a rule, other harmful sound events: applause, laughter (especially laughter in the audience), etc.
The result of the detector's operation is a number from 0 to 1. For classification, it is assumed that if this number is less than 0.5, then in the considered fragment of the recording there are no two voices simultaneously, otherwise there will be an “overlap” of voices.
The main limitation in the use of the detector now is recordings with a noticeable reverberation (“booming” rooms, a noticeable echo, etc.), in which, in a sense, the effect of simultaneous speaking is reproduced.
This is one of the main subsystems of the prototype, which solves the following problem. Given two one-voice fragments of speech of arbitrary length, it is required to determine whether they belong to the voice of one person, or whether they are the voices of different people.
It is based on a neural network solution, trained on the basis of 100 male and 100 female votes (samples are continuously expanding and diversifying). The result is a number from 0 to 1. If it is less than 0.5, then it is believed that the fragments belong to the voice of one person, otherwise to different people.
The quality of the solution directly depends on the length of the speech fragments: the shorter they are, the lower the quality. In practice, the error on fragments lasting less than 0.3-0.4 seconds becomes significant. We will tell you more about this in the technical description of the identifier and in the article.
At the moment, the grinding of the solution for the shortest possible speech fragments continues, and the results are certainly encouraging.
Graphically, the diagram is shown in the figure:

Project curator: Mikhail Grinenko, Ph.D., Neurodata Lab scientific adviser on deep training and data analysis.
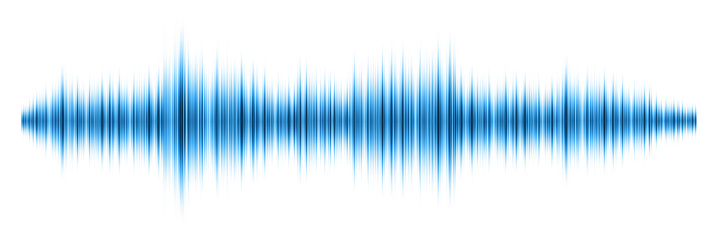
Of course, at the moment we are at the stage of preparing a full-fledged article, as well as assembling and packaging a future commercial product, so here we only briefly outline our activities in this area with an invitation to discuss the results after their publication and presentation at conferences in the first half of 2018.
So, what do we have as of today. A working prototype of a system designed to solve the following tasks under the following conditions:
- At the entrance there is a single-channel recording of a conversation of two (potentially more) people in WAV format;
- All fragments are deleted from the recording, where two (or more) voices simultaneously sound; removal is associated with the need to further process the speech of a particular person, for example, to determine the characteristics of the voice and emotional state of the speaker;
- The remaining fragments of the record are divided into two groups so that each of them contains the speech of only one specific person;
- At the output there are two audio channels: in the first one, one person speaks, in the second - the other person; timing is saved.
The technological basis of the solution are three subsystems:
- Highlight (speech) phrases;
- Simultaneous speech detector;
- Voice ID
Phrase selector
The phrase in this context refers to a continuous section of speech between two micro-pauses. The concept is inaccurate, conditional, the result of the use of the phrase extractor strongly depends on the pronunciation features (jerky or “smoothed”, continuous speech), on the parameters of “micropause”, etc. Typical settings of the phrase extractor lead to the fact that the phrase, as a rule, is a sequence of phonemes, syllables, sometimes words lasting from 0.2 to several seconds. The exact settings of the phrase identifier will be given in its technical description.
The meaning of using the phrase extractor is as follows. If we remove from the speech the moments of simultaneous sounding of two voices, then the remaining record will be alternating (without overlapping) sections of one-voice speech, while in most cases the speaker will change at the border of phrases.
This assumption is not entirely true, in practice there is a pause-free transition of speech from one speaker to another. However, these cases are indeed rare, and in the proposed prototype, the main negative effect of such pause-free transitions is reduced to the incorrect formation of basic support fragments of the voices of two people and partially stopped by the principle of the formation of such support fragments.
Thus, modulo the absence of phrases with the transition of voices, further work (after highlighting phrases and throwing out moments of simultaneous speech) is reduced to the task of voice identification of phrases.
Simultaneous speech detector
In addition to the original function (we only need fragments of one-voice speech), the detector allows you to leave only those phrases (or parts of them) where one voice sounds (modulo phrases with voice transition, which was higher), thereby reducing the problem to the problem of voice identification .
The operation of the simultaneous speech detector is based on visual observation: the log spectrogram or its time derivative in the areas of simultaneous speech contains characteristic irregularities that are absent in single-voice areas and are easily distinguishable by the eye. Examples will be given in the detector description.
In connection with this observation, the solution is based on 2D convolution networks, which are designed to highlight graphic features. However, the current prototype contains additional, 1D-convolutional neural network solutions to improve the quality of detection.
The idea underlying the detector turned out to be quite successful in the sense that not only moments of simultaneous speech are determined, but, as a rule, other harmful sound events: applause, laughter (especially laughter in the audience), etc.
The result of the detector's operation is a number from 0 to 1. For classification, it is assumed that if this number is less than 0.5, then in the considered fragment of the recording there are no two voices simultaneously, otherwise there will be an “overlap” of voices.
The main limitation in the use of the detector now is recordings with a noticeable reverberation (“booming” rooms, a noticeable echo, etc.), in which, in a sense, the effect of simultaneous speaking is reproduced.
ID of votes
This is one of the main subsystems of the prototype, which solves the following problem. Given two one-voice fragments of speech of arbitrary length, it is required to determine whether they belong to the voice of one person, or whether they are the voices of different people.
It is based on a neural network solution, trained on the basis of 100 male and 100 female votes (samples are continuously expanding and diversifying). The result is a number from 0 to 1. If it is less than 0.5, then it is believed that the fragments belong to the voice of one person, otherwise to different people.
The quality of the solution directly depends on the length of the speech fragments: the shorter they are, the lower the quality. In practice, the error on fragments lasting less than 0.3-0.4 seconds becomes significant. We will tell you more about this in the technical description of the identifier and in the article.
At the moment, the grinding of the solution for the shortest possible speech fragments continues, and the results are certainly encouraging.
Graphically, the diagram is shown in the figure:

Project curator: Mikhail Grinenko, Ph.D., Neurodata Lab scientific adviser on deep training and data analysis.