Noam Chomsky: where did artificial intelligence go wrong?
- Transfer
Translator's commentary: A detailed interview with the legendary linguist, published 6 years ago, but in no way lost its relevance. Noam Chomsky - “modern Einstein”, as it is called, shares his thoughts on the structure of human thinking and language, artificial intelligence, the state of modern sciences. The other day he turned 90 years old, and this seems to be a sufficient reason to publish an article. The interview is conducted by a young cognitive scientist Yarden Kats, he himself is well versed in the subject, so the conversation is very informative, and the questions are as interesting as the answers.
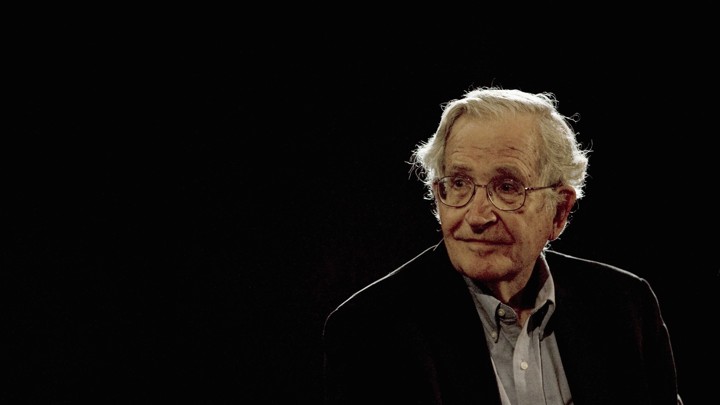
If we aim to make a list of the greatest and most unattainable intellectual tasks, then the task of “decoding” ourselves - understanding the internal structure of our minds and brains, and how the architecture of these elements is encoded in our genome - would definitely be at the top. However, the various areas of knowledge that were taken on this task, from philosophy and psychology to computer science and neuroscience, are overwhelmed by disagreement about which approach is correct.
In 1956, computer scientist John McCarthy introduced the phrase "artificial intelligence" (AI) to describe the science of studying the mind by recreating its key features on a computer. Creating a smart system using hand-made equipment, instead of our own “equipment” in the form of cells and tissues, should have become an illustration of complete understanding, and entail practical applications in the form of smart devices or even robots.
However, some of McCarthy’s colleagues from related disciplines were more interested in how the mind works in humans and other animals. Noam Chomsky and his colleagues worked on what later became known as cognitive science - the discovery of mental concepts and rules that underlie our cognitive and mental abilities. Chomsky and his colleagues overturned the dominant at that moment paradigm of behaviorism, led by Harvard psychologist B.F. Skinner, in which the behavior of animals was reduced to a simple set of associations between action and its effect in the form of encouragement or punishment. The shortcomings of Skinner's work in psychology became known from Chomsky’s 1959 criticism of his book Verbal Behavior, in which Skinner tried to explain language skills using behavioral principles.
Skinner's approach focused on the association between stimulus and animal response — an approach that is easily representable as an empirical statistical analysis that predicts the future as a consequence of the past. The concept of the Chomsky language, on the other hand, focused on the complexity of the internal representations encoded in the genome and their development in the course of obtaining data into a complex computer system that cannot be simply decomposed into a set of associations. The behavioral principle of associations could not explain the richness of language knowledge, our infinitely creative use of it, or why children quickly learn it from the minimal and noisy data that the environment provides them. “Language competence,” as Chomsky called it, was part of the body’s genetic makeup, as was the visual system, the immune system, the cardiovascular system,
David Marr, a specialist in neuroscience — Chomsky’s MIT colleague — identified a common approach to studying complex biological systems (such as the brain) in his sensational book, "Vision," and Chomsky’s language competence analysis more or less fits this approach. According to Marr, a complex biological system can be understood at three different levels. The first level (“computational level”) describes the system input and output, which define the task performed by the system. In the case of the visual system, the input can be an image projected on our retina, and the output can be the identification of objects in the image by our brain. The second level (“algorithmic level”) describes the procedure by which input turns into output, that is: how the image on our retina can be processed to achieve the task, described at the computational level. Finally, the third level (“implementation level”) describes how our biological equipment from cells performs the procedure described at the algorithmic level.
Chomsky and Marr’s approach to understanding how our mind works is as far from behaviorism as possible. Here, the emphasis is on the internal structure of the system, which allows it to accomplish a task, rather than on an external association between the past behavior of the system and the environment. The goal is to penetrate the “black box” that controls the system and describe its internal structure, something like a programmer can explain to you the principle of how a well-developed software product works, and also instruct how to run it on a home computer.
As is now commonly believed, the history of cognitive science is the history of Chomsky’s apparent victory over Skinner’s behavioral paradigm — an event often referred to as a “cognitive revolution”, although Chomsky himself denies such a name. This accurately reflects the situation in cognitive science and psychology, but in other related sciences, behavioral thinking is not going to die. Behaviorist experimental paradigms and associationist explanations of animal behavior are used by neuroscience specialists, whose goal is to study the neurobiology of laboratory animal behavior, such as rodents, where the three-level system approach proposed by Marr is not applicable.
In May 2011, in honor of the 150th anniversary of the Massachusetts Institute of Technology, a symposium “Brains, Minds and Machines” (Brains, Minds and Machines) was held, at which leading informatics scientists, psychologists and neuroscience specialists gathered to discuss past and future artificial intelligence and its connection to neuroscience.
The implication was that the meeting would inspire everyone with interdisciplinary enthusiasm for reviving the scientific question from which the whole field of artificial intelligence grew: How does the mind work? How did our brain create our cognitive abilities, and can it ever be embodied in a machine?
Noam Chomsky, speaking at the symposium, was not enthusiastic. Chomsky criticized the AI sphere for adopting an approach similar to behaviorism, only in a more modern, computationally complex form. Chomsky said that reliance on statistical techniques for finding patterns in large volumes of data is unlikely to give us the explanatory guesses that we expect from science. For Chomsky, a new AI — focused on using statistical training techniques for better data processing and making predictions based on them — is unlikely to give us general conclusions about the nature of rational beings or how thinking is arranged.
This criticism evoked Chomsky’s detailed response from Google’s research director and renowned AI researcher, Peter Norvig, who defended the use of statistical models and argued that the new AI methods and the definition of progress were not far from what was happening. and in other sciences.
Chomsky replied that the statistical approach may be of practical value, for example, for a useful search engine, and it is possible with fast computers that can process large amounts of data. But from a scientific point of view, Chomsky believes, this approach is inadequate, or, speaking more strictly, superficial. We have not taught a computer to understand what the phrase "physicist Sir Isaac Newton" means, even if we can build a search engine that returns plausible results to users who enter this phrase there.
It turns out that there are similar disputes among biologists who are trying to understand more traditional biological systems. As the computer revolution opened the way to the analysis of large amounts of data, on which the whole “new AI” holds, so the sequencing revolution in modern biology gave rise to flowering fields of genomics and system biology. High-throughput sequencing, a technique by which millions of DNA molecules can be quickly and cheaply read, has transformed the genome sequencing from an expensive 10-year-long enterprise into a laboratory procedure available to ordinary people. Instead of the painful study of separate isolated genes, we can now observe the behavior of a system of genes acting in cells as a whole, in hundreds, thousands of different conditions.
The sequencing revolution has just begun, and a huge amount of data has already been obtained, bringing with it excitement and new promising prospects for new therapies and diagnostics of human diseases. For example, when the usual medicine does not help a certain group of people, the answer may be in the genome of patients, and there may be some feature that does not allow the medicine to work. When enough data has been gathered to compare the relevant features of the genome in such patients, and the control groups are correctly selected, new customized medicines may appear leading to something like “personalized medicine”. It is understood that if there are sufficiently developed statistical tools and a sufficiently large data set, interesting signals can be pulled out of the noise,
The success of such phenomena as personalized medicine and other consequences of the sequencing revolution and the system-biological approach is based on our ability to work with what Chomsky calls the “mass of raw data” —and this puts biology at the center of the discussion, like the one in psychology and artificial intelligence since the 1960s.
Systems biology also met with skepticism. The great geneticist and Nobel laureate Sydney Brenner once defined it: “low input, high throughput, no output science” (loosely translated: “much ado about nothing, and no science at the end”). Brenner, the same age as Chomsky, who also participated in that symposium on AI, was just as skeptical about the new systemic approaches to understanding the brain. Describing a popular systems approach to mapping brain circuits, called Connectomics, which attempts to describe the connections of all neurons in the brain (that is, makes a diagram of how some nerve cells are connected to others), Brenner called it a "form of madness."
The ingenious attacks of Brenner on systems biology and related approaches in neuroscience are not far from Chomsky’s criticism in the direction of AI. Unlike externally, systems biology and artificial intelligence encounter the same fundamental task of reverse engineering of a highly complex system, whose internal structure is mostly a mystery. Yes, emerging technologies provide a large array of data related to the system, of which only a fraction can be relevant. Should we rely on powerful computational capabilities and statistical approaches to isolate the signal from noise, or should we look for more basic principles underlying the system and explaining its essence? The desire to collect more data is unstoppable, although it is not always clear what theory this data can fit into. These discussions raise the eternal question of the philosophy of science: What makes a scientific theory or explanation satisfactory? How is success determined in science?
We sat with Noam Chomsky on a April afternoon in a rather messy conversation, hiding in a secret corner of Frank Gehry’s dizzying Static Center MIT building. I wanted to better understand Chomsky’s criticism of artificial intelligence, and why, he believes, he is moving in the wrong direction. I also wanted to explore the application of this critique to other scientific areas, such as neuroscience and systems biology, which all work with the task of reverse engineering complex systems — and where scientists often find themselves in the midst of an infinitely expanding sea of data. Part of the motivation for the interview was that Chomsky is now rarely asked about science. Journalists are too interested in his opinion on US foreign policy, the Middle East, the Obama administration, and other common topics. Another reason was that Chomsky belongs to that rare and special kind of intellectuals that is rapidly dying out. Since the publication of the famous essay by Isaiah of Berlin, various thinkers and scholars began to put Fox-Hedgehog as a favorite entertainment in the academic environment: Hedgehog - meticulous and specialized, aimed at consistent progress in clearly defined framework, against Fox, more rapid, driven by ideas thinker who jumps from question to question, ignoring the scope of the subject area and applying his skills where they apply. Chomsky is special because he makes this distinction into an old and unnecessary cliché. Chomsky has no depth in return for flexibility or breadth, although, for the most part, he devoted his entire early scientific career to the study of certain topics in linguistics and cognitive sciences.
I want to start with a very simple question. At the dawn of artificial intelligence, people were optimistic about progress in this area, but everything turned out differently. Why is the task so difficult? If you ask specialists in the field of neuroscience why it is so difficult to understand the brain, they will give you absolutely unsatisfying answers: there are billions of cells in the brain, and we cannot read them all, and so on.
Chomsky:There is something in it. If you look at the development of science, all sciences are like a continuum, but they are divided into separate areas. The greatest progress is achieved by science, which studies the simplest systems. Take, for example, physics — there is tremendous progress in it. But one of the reasons is that physicists have an advantage that no other science has. If something gets too complicated, they pass it on to someone else.
For example, chemists?
Chomsky: If the molecule is too big, you give it to the chemists. Chemists, if for them the molecule is too large or the system becomes too large, give it to biologists. And if it’s too big for them, they give it to psychologists, and in the end it ends up in the hands of literary critics, and so on. So not everything that is said in neuroscience is completely wrong.
But maybe - and from my point of view it is very likely, although neuroscience doesn’t like it - that neuroscience has been on the wrong path for the last couple of hundred years. There are quite a new book very good neyrouchonogo the cognitive Randy Gallistela together with Adam King ( «the Memory and the Computational Brain: Why the Cognitive Science will of the Transform Neuroscience» - ca. Perevi.. ) In which he claims - in my view, plausibly - that neuroscience developed, being fascinated by associationism and related ideas about how people and animals work. As a result, they were looking for phenomena with associative psychology properties.
How with hebbov plasticity? [Theory attributed to Donald Hebb: associations between environmental stimuli and stimulus responses can be encoded by enhancing synaptic connections between neurons - approx. Ed.]
Chomsky:Yes, as the strengthening of synaptic connections. He spent years trying to explain: if you want to study the brain properly, you need, like Marr, to first ask what tasks he performs. Therefore, he is mainly interested in insects. So, if you want to study, say, the ant's neurology, you ask, what does an ant do? It turns out that ants do quite complex things, such as building a path. Look at the bees: their navigation requires quite complex calculations, including the position of the sun, and so on. But in general, with which he argues: if you take the cognitive abilities of an animal or person, these are computing systems. So you need to look at computational atomic units. Take the Turing machine, this is the simplest form of calculation, you need to find atoms that have “read” properties, "Write" and "address". These are minimal computational units, so you need to look for them in the brain. You will never find them if you look for the enhancement of synaptic connections or the properties of fields, and so on. You need to start with the following: see what is already there and what works, and you can see it from the highest level in the Marr hierarchy.
True, but most neuroscientists do not sit and do not describe the inputs and conclusions of the phenomenon they are studying. Instead, they put the mouse in a laboratory learning task, and record as many neurons as possible, or find out if the X gene is necessary for learning the task, and so on. Such assertions stem from their experiments.
Chomsky: Is that so ...
Is there a conceptual mistake in this?
Chomsky: Well, you can get useful information. But if there really is some kind of calculation that involves atomic units, you will not find them in this way. It's about how to look for lost keys under a different lantern, just because it’s lighter there (a reference to a well-known joke - approx. Transl.). This is a debatable question ... I do not think that the position of Gallistel was widely accepted by neurobiologists, but this is a plausible position, and it was made in the spirit of Marr’s analysis. So when you study vision, he says, you first ask what computational problems the system solves. Then you are looking for an algorithm that could perform these calculations, and in the end you are looking for mechanisms that allow you to make such an algorithm work. Otherwise, you can never find anything. There are many examples of this, even in the exact sciences, and most certainly in the humanities. People try to study what they know how to study - I mean, it looks reasonable. You have certain techniques of experiments, you have a certain level of understanding, you are trying to push the boundaries of the possible - and this is good, I do not criticize, people do what they can. On the other hand, It would be nice to know if you are moving in the right direction. And it may happen that if you take the point of view of Marra-Gallestel, which I personally sympathize with, then you will work differently, look for experiments of another kind.
So, I think the key idea of Marr is, as you said, to find suitable atomic units to describe the problem, in other words, the appropriate "level of abstraction", if you can say so. And if we take a concrete example of a new neuroscience area called Connectomics, where the goal is to find a diagram of the connections of very complex organisms, to find the connections of all the neurons of the cortex of a person’s brain or mouse. This approach was criticized by Sydney Brenner, who is to a large extent [historically] one of its authors. Advocates of this area do not stop and do not ask whether the diagram of links is a suitable level of abstraction — it may not be. What is your opinion about this?
Chomsky: There are much simpler questions. For example, here at MIT, there was an interdisciplinary program for studying nematodes (roundworm - approx. trans. ) C. elegans for several decades, and, as I understand it, even with this tiny creature that you know the whole diagram of connections, there are 800 neurons or so ...
I think 300 ..
Chomsky: ... Anyway, you are not you can predict what it is [nematode C. elegans] is going to do. Maybe you're just looking for the wrong place.

I would like to go on the topic of various methodologies in AI. So, “Good old artificial intelligence” (GOFAI), as it is called now, was based on strict formalisms in the tradition of Gottlob Frege and Bertrand Russell, on mathematical logic, for example, or its branches, as non-monotonic reasoning, and so on. From the point of view of the history of science, it is interesting that these approaches were almost completely excluded from the mainstream and were replaced - in the field that now calls itself AI - probabilistic and statistical models. My question is: how can this shift be explained, and is this a step in the right direction?
Chomsky: I listened to Pat Winston's report on this a year ago. He had one of the theses: AI and robotics have reached a stage where you can do really useful things, so attention turned to practical application, and therefore more fundamental scientific questions were put aside, simply because everyone is caught up in the success of technology and achieving certain goals.
That is, everything went into engineering ...
Chomsky: Yes, that is true ... And this is quite understandable, but, of course, it leads people away from the initial questions. I myself have to admit that I was very skeptical about these original works ( in the new paradigm of probabilistic AI - approx. Transl. ). It seemed to me that everything was too optimistic, it was assumed that you could achieve results that require real understanding of barely studied systems, and that you cannot come to their understanding simply by throwing a complicated machine there. If you try to do this, you come to the concept of self-reinforcing success, because you get the result, but it is very different from how it is done in the sciences.
For example, take the extreme case, suppose that someone wants to abolish the physics department, and do it right. And the “right” is to take a lot of videos about what is happening in the outside world and feed them to the largest and fastest computer, gigabytes of data, and make a comprehensive statistical analysis - well, you know, Bayesian methods, back and forth. (modern approach to data analysis based on probability theory - ed.)- and you get something like a prediction about what happens outside your window the next second. In fact, you will receive a much better prediction than the physics department could give you. Well, if success is determined to get the closest approximation on a mass of chaotic raw data, then of course this is a much better way than physics usually does — well, you know, no more thought experiments on a perfectly flat surface, and so on . But you will not get the level of understanding that has always been the goal of science - you only get an approximation to what is happening.
And so it is done everywhere. Suppose you want to predict the weather for tomorrow. One way: OK, I have statistical a priori probabilities, for example: there is a high probability that tomorrow the weather will be the same as it was yesterday in Cleveland, and I use it, and the sun's position will have some influence, and this I also use, so , you made a few such assumptions, you conduct an experiment, you look at the results again and again, you correct using Bayesian methods, you get the best prior probabilities. You get a pretty good approximation of what the weather will be like tomorrow. But this is not what meteorologists do - they want to understand how it works. And these are just two different concepts of what success is, what achievement is. In my science, the science of language, it is very often. In computational cognitive science, applied to the language, the concept of success is this. That is, you are getting more and more data, better statistics, getting more and more accurate approximation to some kind of gigantic body of text, for example, all the Wall Street Journal archives - but you don’t learn anything about the language.
A completely different approach, which I think is right, is to try to see if you can understand what the fundamental principles and their connection with key properties are, and see that in real life thousands of different variables will interfere with you - like what is happening now for window - and you will deal with them later, if you want a more accurate approximation. These are just two different concepts of science. The second is what science has been since Galileo, it is modern science. Approximation of the raw data is like a new approach, but in fact, such things existed in the past. This is a new approach that is accelerated by the existence of large amounts of memory, very fast processing, which allow you to do things that you previously could not do manually. But I think,
... in engineering?
Chomsky:... But leads away from understanding. Yes, maybe even effective engineering. And this, by the way, is interesting what happened with engineering. When I got to MIT in the 1950s, it was an engineering college. There was a very good faculty of mathematics, physics, but they were servicing faculties. They trained engineers for all sorts of tricks they could use. At the Faculty of Electronic Engineering, you studied how to assemble a circuit. But since the 1960s and to this day, everything is completely different. No matter what your engineering specialty is, you study all the same basic sciences and mathematics. And then maybe you are learning a little about how to apply it. But this is a completely different approach. It became possible due to the fact that for the first time in the history of mankind, the basic sciences, like physics, could really help engineers. Besides, Technologies began to change very quickly, so it makes little sense to study the technologies of today, if after 10 years they change anyway. Therefore, you are studying a fundamental science that will be applicable, no matter what happens next. And about the same thing happened in medicine. So, in the last century, again, for the first time, biology had something to say about practical medicine, and therefore you had to know biology, if you wanted to become a doctor, plus the technologies changed. I think that this is a transition from something like art that you learn to use - the analogy would be a comparison of incomprehensible data in some special way, and maybe even building something working - a transition to science, which appeared in modern times. , roughly speaking, the science of Galileo. if after 10 years they change anyway. Therefore, you are studying a fundamental science that will be applicable, no matter what happens next. And about the same thing happened in medicine. So, in the last century, again, for the first time, biology had something to say about practical medicine, and therefore you had to know biology, if you wanted to become a doctor, plus the technologies changed. I think that this is a transition from something like art that you learn to use - the analogy would be a comparison of incomprehensible data in some special way, and maybe even building something working - a transition to science, which appeared in modern times. , roughly speaking, the science of Galileo. if after 10 years they change anyway. Therefore, you are studying a fundamental science that will be applicable, no matter what happens next. And about the same thing happened in medicine. So, in the last century, again, for the first time, biology had something to say about practical medicine, and therefore you had to know biology, if you wanted to become a doctor, plus the technologies changed. I think that this is a transition from something like art that you learn to use - the analogy would be a comparison of incomprehensible data in some special way, and maybe even building something working - a transition to science, which appeared in modern times. , roughly speaking, the science of Galileo. And about the same thing happened in medicine. So, in the last century, again, for the first time, biology had something to say about practical medicine, and therefore you had to know biology, if you wanted to become a doctor, plus the technologies changed. I think that this is a transition from something like art that you learn to use - the analogy would be a comparison of incomprehensible data in some special way, and maybe even building something working - a transition to science, which appeared in modern times. , roughly speaking, the science of Galileo. And about the same thing happened in medicine. So, in the last century, again, for the first time, biology had something to say about practical medicine, and therefore you had to know biology, if you wanted to become a doctor, plus the technologies changed. I think that this is a transition from something like art that you learn to use - the analogy would be a comparison of incomprehensible data in some special way, and maybe even building something working - a transition to science, which appeared in modern times. , roughly speaking, the science of Galileo.
Clear. Returning to the topic of Bayesian statistics in models of language and cognition. There was a famous dispute with your participation, you argued that talking about the likelihood of a proposal is unwise in itself ...
Chomsky: ... Well, you can get a number if you want, but it means nothing.
It means nothing. But it seems that there is an almost trivial way to unify the probabilistic method, assuming that there are very rich internal mental representations consisting of rules and other symbolic structures, and the goal of the theory of probability is simply to link the noisy, fragmentary data of our world with these internal symbolic structures. And you are not required to say something about how these structures appeared - they could exist initially, or if some parameters are adjusted there - depends on your concept. But probability theory works like a glue between noisy data and very rich mental notions.
Chomsky: There is nothing wrong with probability theory, statistics.
But does she have a role?
Chomsky:If you can use it, fine. But the question is, what are you using it for? First of all, the very first question, is there any point in understanding the noisy data? Does it make sense to understand what is happening outside, outside the window?
But we are bombarded with this data. This is one example of Marr: we encounter noisy data constantly, starting from our retina and up to ...
Chomsky:This is true. But here is what he says: Let's ask ourselves how the biological system selects from the noise important. Retina is not trying to duplicate incoming noise. She says: I will now look for this in the image, this and this. This is about language learning. The newborn baby is surrounded by various noises, as William James said, “a blooming and buzzing mess.” If a monkey, a kitten, a bird, anyone, hears this noise, that's where it ends. However, the child in some way, immediately, reflexively, chooses from the noise a separate part, which is connected with the language. This is the first step. How he does it? Not with statistical analysis, because the monkey can also roughly perform the same probabilistic analysis. He is looking for a specific thing. So, psycholinguists, neuro-linguists and others are trying to uncover specific details of the computing system and neuropsychology that are somehow related to specific aspects of the environment. So, it turns out that there really are neural circuits that react to certain types of rhythm, which manifests itself in the language - like the length of syllables and so on. And there is some evidence that one of the first things that a child’s brain is looking for is rhythmic structures. And going back to Gallistel and Marr, the brain has some kind of computing system inside that says: “Okay, this is what I’ll do with these things,” and after about nine months a typical child has already eliminated — removed from his supply — those phonetic differences, which is not in its own language. That is, it turns out, from the very beginning, any child is tuned to any language. But let's say A Japanese child at the age of nine months will not react to the difference between “P” and “L”, it is sort of dropped out. So the system considers a lot of possibilities and limits them only to those that are part of the language, and this is already quite a narrow set. You can come up with an anti-language in which the child can never do it, and much more interesting. For example, if we talk about the more abstract side of the language, at the moment there is solid evidence that such a simple thing as the linear word order — what follows it — is not part of the syntactic and semantic computing systems, their design is simply such that they are not looking for a linear order. It can be seen that more abstract concepts of distance are predominantly used, and this is not a linear distance, and neurophysiological confirmation can be found for this. We can give an example: if you come up with an artificial language in which the linear word order is used, as, for example, you make a negative sentence with an affirmative sentence, doing something with the third word in a row. People will be able to solve this puzzle, but apparently the standard language areas of the brain are not activated - other areas are activated, that is, people perceive it as a puzzle, and not as a language task. And to solve it, people have to strain more ... that is, people perceive it as a puzzle, and not as a language task. And to solve it, people have to strain more ... that is, people perceive it as a puzzle, and not as a language task. And to solve it, people have to strain more ...
You consider this to be a convincing evidence that activation or lack of activation of a brain area ...
Chomsky: ...This is evidence, and of course, you want more. But this evidence is such that you see from the linguistic side how languages work — there are no such things in them as the third word in a sentence. Take a simple sentence: “Instinctively flying eagles swim,” here “instinctively” is associated with the word “swim,” and not with the word “flying,” even though the entire sentence does not make sense. And here is the action of the reflex. “Instinctively,” adverb, does not search for the nearest verb, it searches for a structurally more suitable verb. This is a much more complicated calculation. But this is the only calculation that is generally used. Linear order is a very simple calculation, but it is never used. There is a lot of evidence like this, and very little neuro-linguistic evidence, but they point in the same direction.
This, in my opinion, is a way to understand how the system actually works, as it happened with the vision system in Marr's laboratory: people like Shimon Ulman discovered quite remarkable things like the principle of rigidity. You can’t find it with statistical data analysis. He found this through carefully planned experiments. Then you look in neurophysiology and see if you can find something that performs these calculations. I think the same thing in language, the same thing in the study of our arithmetic abilities, planning, almost everywhere. Just working with raw data - you will not come anywhere with it, and Galileo would not have come. In fact, if you come back to this, in the 17th century it was not easy for people like Galileo and other great scientists to convince the National Science Foundation of those times - namely, aristocrats - that their work had a meaning. I mean: why study how the ball rolls on a perfectly flat plane without friction, because they do not exist. Why not study how flowers grow? If you tried to study the growth of flowers in those times, you would probably get a statistical analysis of how everything works.
It is important to remember that in cognitive science we are still in the pre-Galilean era, we are just starting to make discoveries. And I think that one can learn something from the history of science. One of the main experiments in the history of chemistry in 1640 or so, when someone proved, to the pleasure of the entire scientific world up to Newton, that water can be turned into living matter. That's how they did it - of course, no one knew anything about photosynthesis - they took a lot of land, and heated it so that all the water evaporated. It was weighed, a willow branch was inserted into it, and poured over with water, measuring the volume of this water. When everything is ready and the willow tree has grown, you again take the earth and evaporate water from it - just as before. Thus, you have shown that water can turn into an oak or something else. This is an experiment, and it seems to be even true, but you do not know what you are looking for. And it was not known until Priestley discovered that air is a component of the world, there is nitrogen in it, and so on, and you learned about photosynthesis and so on. Then you can repeat the experiment and understand what is happening. But you can easily be led away in the wrong direction by an experiment that seems successful because you do not understand well what you should be looking for. And you will go even further in the wrong direction if you try to study the growth of trees like this: just take an array of data on how trees grow, feed it to a powerful computer, conduct a statistical analysis and get an approximation of what happened. But you can easily be led away in the wrong direction by an experiment that seems successful because you do not understand well what you should be looking for. And you will go even further in the wrong direction if you try to study the growth of trees like this: just take an array of data on how trees grow, feed it to a powerful computer, conduct a statistical analysis and get an approximation of what happened. But you can easily be led away in the wrong direction by an experiment that seems successful because you do not understand well what you should be looking for. And you will go even further in the wrong direction if you try to study the growth of trees like this: just take an array of data on how trees grow, feed it to a powerful computer, conduct a statistical analysis and get an approximation of what happened.
In biology, do you assess Mendel’s work as a successful example of how to get noisy data — it’s important that the numbers are — and jump to the postulation of a theoretical object ...
Chomsky: ... And throwing out a huge amount of data that did not work.
... But after seeing the relation that made sense, develop a theory.
Chomsky:Yes, he did everything right. He allowed the theory to manage data. There were more data that contradicted the theory, which were more or less discarded, well, you understand - which usually will not be included in the article. And he, of course, talked about things that no one could find, how it was impossible to find the units, the existence of which he argued. But yes, that's how science works. Also in chemistry. Chemistry, before my childhood, not so long ago, was considered as the science of computing. Because it can not be reduced to physics. Therefore, it is just a way to calculate the result of experiments. Atom Bora so perceived. The way to calculate the result of experiments, but this cannot be a real science, because it cannot be reduced to physics, and suddenly, it turned out to be true, because physics was wrong. When quantum physics appeared, it became possible to combine it with no change in chemistry. That is, the whole project with the reduction was simply wrong. The correct project was to see how you can combine these two world views. And it turned out that, surprise - they were united by radical changes in the downstream science. Maybe exactly the same thing with psychology and neuroscience. I mean, neuroscience is not even nearly as advanced now as physics a century ago.
And it will be a departure from the reductionist approach with the search for molecules ....
Chomsky: Yes. In fact, the reductionist approach has been wrong several times. The unification approach makes sense. But the unification may differ from the reduction, since there may be a flaw in the basic science, as in the case of physics and chemistry, and I suspect with a high degree of probability the same in the case of neuroscience and psychology. If Gallistel is right, then it would make sense to say that yes, they can be combined, but with a different approach to neuroscience.
Should we strive for speedy unification, or is it better to continue developing these areas in parallel?
Chomsky:Unification is such an intuitive pursuit of an ideal, part of scientific mysticism, if you like. It is like a search for a general theory of the world. Maybe it does not exist, maybe different parts work differently, but there is an assumption, until I was given a convincing refutation, my assumption is that there is a general theory of the world, and my task is to try to find it. Unification may not manifest itself through reduction, and often it happens. This is the leading logic of David Marr's approach: what you discover at the computational level should be combined with what you once find at the level of mechanisms, but maybe not in the terms in which we now understand these mechanisms.
And Marr implies that you cannot work at all three levels in parallel [computational, algorithmic and implementation level], you need to move from top to bottom, and this is a very strict requirement, given that science is usually not the case.
Chomsky: He could not say such that everything should be tough. For example, discovering something new about mechanisms may lead you to change the concept of computing. The logical order does not necessarily coincide with the order of research, since in research everything happens at the same time. But I think that in a rough approximation, the picture is correct. Although I must say that Marr's concept was developed for input systems ...
Information processing systems ...
Chomsky:Yes, like sight. There is data - this is a data processing system - and something happens in it. And this does not work well for cognitive systems. Take your ability to do arithmetic ...
She's very weak, but okay ...
Chomsky: Good [laughs]. But this is an internal ability, you know that your brain is a control node of something like a Turing machine, and it has access to external data, such as memory, time ... Theoretically, you can multiply anything, but in practice, of course , not this way. If you try to learn what your internal system is, then Marr's hierarchy does not work very well. You can talk about the computational level: maybe the rules are inside me, these are Peano's axioms . ed.: The mathematical theory (named after the Italian mathematician Giuseppe Peano), describing the core of the basic rules of arithmetic and natural numbers, from which you can derive a lot of useful arithmetic facts] or something else, it does not matter - this is a computational level. Theoretically, although we do not know how, you can only talk about the neurophysiological level, nobody knows how, but there is no real algorithmic level. Because there is no calculus of knowledge, it is just a system of knowledge. It is not clear how to understand the nature of the system of knowledge: there is no algorithm there, because there is no process there. This can be done only by using a knowledge system in which there is a process, but this will already be something completely different.
But since we make mistakes, does this mean that the process is going wrong?
Chomsky:This is the process of using the internal system. But the internal system itself is not a process, since it does not have an algorithm. Take the usual math. If you take Peano's axioms and the rules of inference, they define all arithmetic calculations, but there is no algorithm there. If you ask how a number theory specialist applies them, there are, of course, many options: for example, you start not with axioms, but with the rules of inference. You take a theorem and see if it is possible to derive a lemma, and if it works, then see if it turns out to base this lemma on something, and at the end you get a proof - a geometric object.
But this is a fundamentally different activity, different from the addition of small numbers in my head - and of course, I have some sort of algorithm in my head.
Chomsky:Not necessary. There is an algorithm for this process in both cases. But there is no algorithm of the system itself, this is a categorical error. You are not asking what process defines Peano's axioms and the rules of inference; there is no process there. There may be a process to use them. And this can be a complicated process, and this is true of your calculations. The internal system that you have - here the question of the process does not arise. But if you use your internal system, the question arises, and you can perform multiplication in different ways. For example, if you add 7 and 6, one algorithm says: "I will see how much it takes to get to 10" - it takes 3, and now there are 3 more left, so I will move from 10 and add 3 more, and 13. This is an addition algorithm - in fact, as I was taught in kindergarten. This is one way to add numbers.
But there are other ways to add - there is no correct algorithm. These are the algorithms for performing the cognitive system process in your head. And now for this system you do not ask about the algorithms. You can ask about the computing level, about the level of mechanisms. But the algorithmic level for this system does not exist. The same with the language. Language is like arithmetic ability. There is a system that determines the sound and meaning of an infinite array of possible sentences. But there is no question about what kind of algorithm is there. There is also no question of what formal arithmetic system tells you how to prove theorems. Using the system is a process, and you can study it in terms of Marr levels. But it is important to conceptualize these differences.
It just seems like an incredible task to move from the theory of computational level, like Peano's axioms, to Marrow level 3 ...
Chomsky: mechanisms ...
... mechanisms and realizations ...
Chomsky: Yes. And ...
... without an algorithm, at least.
Chomsky:I think this is wrong. Maybe the information on how the system is used and tell you something about the mechanisms. But some higher intelligence - maybe higher than ours - will see that there is an internal system, and it has a physiological basis, and it will be possible to study this physiological basis. Even without looking at the process in which this system is used. Maybe watching the process gives you useful information about where to go. But conceptually this is another task. The question is which way to conduct research is better. So, maybe the best way to study the connection between Peano's axioms and neurons is to observe how mathematicians prove theorems. But this is only because I will give you supporting information. The real end result will be an understanding of the brain system, its physiological basis, without reference to any algorithm. Algorithms are all about the processes that use them, and they can help you get answers. Perhaps, as inclined surfaces can tell you something about the speed of falling, but if you look at the laws of Newton, they say nothing about inclined planes.
Good. The logic of studying cognitive and language systems using the Marr approach is understandable, but since you do not recognize language competence as a genetic feature, you can apply this logic to other biological systems — the immune system, the cardiovascular system ...
Chomsky: Exactly, I think that is very similar. You can say the same about the immune system.
And it may even be easier to do this with these systems than with thinking.
Chomsky:But you will expect other answers. You can do this with the digestive system. Suppose someone is studying the digestive system. It is unlikely that he will study what happens when you have gastric flu, or when you have eaten a Big Mac, or something else. Let's return to photographing what is happening outside the window. One way to study the digestive system is to collect all sorts of data about what the digestive system does in various circumstances, enter data into a computer, conduct a statistical analysis - you will get something. But it will not be what the biologist does. He wants from the very beginning to abstract from what is considered - perhaps falsely, because you can always make a mistake - irrelevant variables, like if you have gastric flu.
But this is exactly what biologists do: they take sick people with a sick digestive system, compare them with healthy ones, and measure molecular properties.
Chomsky: They do it at a more advanced stage. They already know a lot about the structure of the digestive system before comparing patients. Otherwise they will not know what to compare, and why one is sick and the other is not.
They rely on statistical analysis to identify the distinctive features. This is a very well-funded approach, because you declare that you study patients.
Chomsky:This may well be a way to obtain funding. This is how to seek funding for linguistics that this may help to treat autism. This is another question altogether [laughs]. But the search logic is to start exploring the system, abstracting from what you, with a high degree of probability, consider irrelevant noise. You are trying to find a basic entity, and then you wonder what happens if we bring something else, the same stomach flu.
It still seems that there is a difficulty in applying Marr levels to systems of this type. If you ask what kind of computational problem the brain solves, then there seems to be an answer, it works approximately like a computer. But if you ask what kind of computational problem the lung solves, it’s even hard to think about it - this is obviously not the task of processing information.
Chomsky: It is, but there is no reason to believe that all biology is computational. There may be reasons to think that thinking is such. And in fact, Gallistel does not say that everything that is in the body should be studied through the search for read / write / address units.
It just seems counterintuitive in terms of evolution. These systems evolved together, reusing similar parts, molecules, trajectories. Cells are computing devices.
Chomsky:You do not study the lung by asking questions about what cells calculate. You study the immune system and the visual system, but you do not expect to find the same answer. The organism is a highly modular system, it has many complex subsystems that are more or less internally combined. They act according to different laws. Biology is also modular. You can not assume that this is all just a huge mess of objects that behave the same way.
Of course not, but I mean that one could apply the same approach to study each of the modules.
Chomsky: Not necessarily, because the modules are different. Some of the modules may be computational, some may not.
Do you think an adequate theory that would have explanatory, rather than just predictive power through statistics ... What could be an adequate theory of these systems that are not computational - can we understand them at all?
Chomsky:Of course. You can understand a lot about, for example, what causes an embryo to turn into a chicken, and not, say, a mouse. This is a very confusing system, including all kinds of chemical interactions and other things. Even with a nematode, the fact that everything is determined simply by the neural network is completely unclear, and there is research data on this matter. You need to look at the complex chemical interactions that occur in the brain, in the nervous system. It is necessary to peer into each system separately. These chemical interactions may not be related to your arithmetic abilities - most likely so. But they can very easily be connected with whether you decide to raise your hand or lower it.
Although, if you begin to study chemical interactions, it will lead you to what you call the repeated description of the phenomenon, only in other words.
Chomsky: Or for an explanation. Because perhaps they are very important, critical, connected.
But if you make an explanation in terms of "substance X must be activated" or "gene X must be present", you really do not explain how the body works. You just found the lever and click on it.
Chomsky: But then you look further, and find what makes this gene work like that in such conditions, or work differently in other conditions.
But if genes are the wrong level of abstraction, then you are in flight.
Chomsky:Then you do not get the right answer. Or maybe not. For example, it is well known that it is difficult to calculate how an organism develops from the genome. There are various kinds of processes taking place in the cell. If you just look at the action of a gene, you may be at the wrong level of abstraction. It is never clear, therefore, it is necessary to study it. I do not think that there is an algorithm for answering such questions.
I would like to shift the conversation towards evolution. You criticized a very interesting point of view, which you called "phylogenetic empiricism." You criticized this position for lack of explanatory power. She simply states the following: so, thinking is what it is, because such adaptations to the environment were chosen. Selected by natural selection. You argued that this explains nothing, because you can always appeal to these two principles - mutation and selection.
Chomsky: Well, you can also give up on them, but they may be right. It may happen that the development of your arithmetic abilities has grown out of random mutations and selection. If it turns out to be so, well, fine.
It sounds like a truism ( well-known truth - approx. Transl. ).
Chomsky: And I'm not saying that this is wrong. Truisms are true [laughs].
But they do not explain.
Chomsky:Maybe this is the highest level of explanation you can get. You can invent a world - I do not think it will be our world - but you can invent a world in which nothing happens except random changes in objects and selection based on external forces. I do not think that our world is like this, and I do not think that there is at least one biologist who thinks so. There are many ways in which natural forces determine the channels through which selection can take place, some things happen, some don't. Very many things in the body do not work that way. Take at least the first step, meiosis: why cells are divided into spheres, and not cubes? These are not random mutations or natural selection: they are the laws of physics. There is no reason to think that the laws of physics stop here, they work everywhere.
Yes, of course, they limit biology.
Chomsky: Okay, that is, it's still not just random mutations and selection. These are: random mutations, selection and everything else that matters, for example, the laws of physics.
Is there a place for these approaches, which are now called "comparative genomics"? The Broadau Institute here [at MIT / Harvard] creates large amounts of data from different genomes from different animals, from different cells in different circumstances, and sequences any molecule that is possible. Is there something that can be learned about high-level cognitive tasks from these comparative evolutionary experiences, or is it an immature approach?
Chomsky: I'm not saying that this is the wrong approach, but I do not know what can be learned from this. Like you.
Do you have examples in which this evolutionary analysis reported something important? For example, mutations Foxp2? [ Approx. Ed: A gene that was thought to be associated with speech or language abilities. In families with typical speech disorders, genetic mutations have been discovered that destroy this gene. It has several mutations that are unique to different stages of human evolution.]
Chomsky:Foxp2 is interesting, but it has nothing to do with the language. It is associated with fine motor skills and similar things. This is related to the use of language, for example, when you say that you control your lips and so on, but this is very peripheral with regard to the language, and this is already known. So, for example, if you use articulating organs or signs, well, for example, a hand gesture is the same language. In fact, it is even analyzed and produced in the same part of the brain, although in one case the arms move, in the other the lips. So whatever the externalization is, it's all on the periphery. I think it is rather difficult to talk about it, but if you look at the language structure, you will get evidence of this. There are interesting examples in language learning, where there is a conflict between computational efficiency and communicative efficiency.
Take this example, which I have already mentioned, with linear order. If you want to know to which verb an adverb is attached, the child reflexively uses the minimum structural distance, and not the minimum linear distance. Yes, it is simpler to use the minimum linear distance from the computational point of view, but for this it is necessary to have the notion of linear order. And if the linear order is only a reflex of the sensorimotor system, which seems reasonable, then it will not be. Here is evidence that the projection of the internal system onto the sensorimotor system is peripheral with respect to the operation of the computing system.
But can it be that the computing system introduces its limitations, how does physics limit meiosis?
Chomsky:Maybe, but there is no evidence. For example, the left end - the left one in the earlier sense - the sentences have different characteristics than the right one. If you want to ask a question, for example: “Whom do you see?” You put the word “Whom” at the beginning, not at the end. In fact, in any language in which the interrogation group — who, whose book — moves elsewhere, it moves to the left, not to the right. This is very likely a limitation of information processing. The offer begins with what the listener tells you: this is what I look like. If you were standing at the end, then you would have a completely declarative sentence, and only at the end would you know what information I ask you. If you say this, it is a limitation to the processing of information. So, if this is the case, then externalization affects the computational nature of syntax and semantics.
There are cases in which you find obvious conflicts between computational and communicative efficiency. Take a simple example: if I say “Visiting relatives can be a burden” - this is ambiguous. Do relatives visit you? Or are you going to visit relatives? It turns out that in every known case, ambiguity arises simply from the fact that we allow the rules to function freely, without restrictions. So it is computationally efficient, but inefficient for communication, because it leads to intractable ambiguity.
Or take the example of sentences with the effect of a garden path leading in the wrong direction. Proposals like: “The horse raced past the barn fell.” ( Horse, which was sent for the barn, fell down - approx. Transl.). People, when they see such a proposal, do not understand it, because it is built in such a way that takes you along the garden path. “The horse raced past the barn” sounds like a sentence, and then you are puzzled: what does the word “fell” do at the end? On the other hand, if you think about it, this is an absolutely correctly formed sentence. It means that the horse, which was directed by the barn by someone, fell. But the rules of the language, when they simply function, may give you incomprehensible sentences due to the phenomenon of the garden path.
And there are many such examples. There are things that you simply cannot say for some reason. If I say: the mechanics fixed the cars. And you will say: "They were interested in whether the mechanics of the machine were repaired." You can ask questions about the machines: “How many machines about which they were interested, did the mechanics repair them?” More or less possible. Suppose you want to ask a question about mechanics. “How many mechanics, they were interested, did they repair the cars?” For some reason it doesn’t work that way, it’s impossible to say so. This is a clear idea, but you cannot say it. If you study this case in detail, the most efficient computational rules prevent you from saying this. But for the expression of thoughts, for communication, it would be better if you could say that - hence the conflict.
And in fact, in each case of such a conflict, computational efficiency wins. Externalization is inferior in all cases of ambiguity, but just for computational considerations, it seems that the system inside does not worry about externalization. I may not be able to show that enough, but just if you say it out loud, it will be a convincing enough argument.
This tells us something about evolution. What is stated by this fact: in the course of the evolution of the language, the computing system developed, and only then it was externalized. And if you think about how a language could develop, you have almost come to this position. At some point of human evolution, and this is obvious, quite recently, if you look at the archaeological data - maybe in the last hundred thousand years ago, and this is just nothing - at some point a computing system appeared with new properties that were not in other organisms, such an arithmetic type of property ...
That is, it allowed you to think better before externalizing?
Chomsky:She gives you thinking. A small flashing of the brain that occurs in an individual, not in a group. That person had the ability to think, the group did not. So there is no sense in externalizing. Then, if this genetic change spreads, and suppose many people have it, then it makes sense to look for a way to project it onto the sensorimotor system, and this is externalization, but this is a secondary process.
Only if externalization and the internal system of thinking are not connected in an unpredictable way.
Chomsky:We do not predict, and this makes little sense. Why should she be connected to an external system? For example, your ability to arithmetic is not connected with it. And there are many other animals, such as songbirds, that have an internal computing system, a bird song. This is not the same system, but it is some kind of internal computing system. And it is externalized, but sometimes not. The chick in some species masters the song of this species, but does not reproduce it until maturity. In this early period he has a song, but there is no externalization system. The same is true of people: a human child understands much more than it can reproduce - quite a lot of experimental evidence - which indicates that the child has an internal system, but he cannot externalize it. Maybe he doesn't have enough memory
I would like to finish with one question about the philosophy of science. In a recent interview, you said that part of the problem is that scientists do not think very much about what they do. You mentioned that you taught philosophy in MIT, and people read, for example, Willard van Orman Quine, and it flew into one ear and into another flew off, and people returned to do their own science just as before. What important insights can be gleaned in the philosophy of science, the most relevant for biologists who want to give an explanatory theory, not a retelling of the phenomenon? What do you expect from such a theory, and what insights will help direct science in such a direction? Instead of leading the science towards behaviorism - an intuition that many neuroscientists are guided by.
Chomsky:The philosophy of science is a very interesting discipline, but I do not think that it really makes a contribution to science - it learns from science. She is trying to understand what the sciences are doing, why achievements are happening in them, which ways are wrong, can it be codified and understood. What I consider important in the history of science. I think we will learn a lot from the history of science of what may be very important for the developing sciences. Especially when we understand that in the cognitive sciences we are still in the pre-Galilean stage. We do not know that we are looking for something that Galileo has already found, and there is something to learn. For example, one startling fact from the early sciences, not necessarily from Galileo, but in general from the time of Galilean discoveries is that simple things can be very confusing. So I hold this cup, and if the water boils, steam will rise, but if I remove my hand, the cup will fall. Why does the cup fall and steam rises? For a thousand years in a row, this was a completely satisfactory answer: they aspire to their natural state.
How in Aristotle's physics?
Chomsky:This is Aristotelian physics. The best and greatest scientists believed that the answer was exactly that. Galileo allowed himself to doubt. As soon as you allow yourself to doubt, you immediately discover that your intuition is wrong. As a fall of a small mass and a large mass, and so on. All your intuitions deceive you - riddles everywhere you look. In the history of science there is something to study. Take the same example that I gave you, "instinctively flying eagles are swimming." No one ever thought it was a mystery. But if you think about it, it is very mysterious, you use complex calculations instead of simple ones. If you let yourself be surprised at this, like dropping a cup, you ask the question “Why?”, And then you get on the path of rather interesting answers. As, for example: linear order is not part of the computing system, which is an important assumption about the architecture of thinking - it says that the linear order is only part of the externalization system, that is, the secondary system. And this opens up a huge number of other ways.
Or take another example: the difference between reduction and unification. The history of science provides some very interesting illustrations in chemistry and physics, and I think they are quite relevant to the state of the cognitive and neurophysiological sciences of modern times.
Afterword of the translator: during the time that has passed since the interview, other interesting materials came out for Chomsky - you can offer to get acquainted with the 2.5-hour conversation with the American physicist Lawrence Krauss, or with the new book by Chomsky and Berwick “A Man Speaking” if you are interested evolution and language.
Translated by Tatyana Volkova.
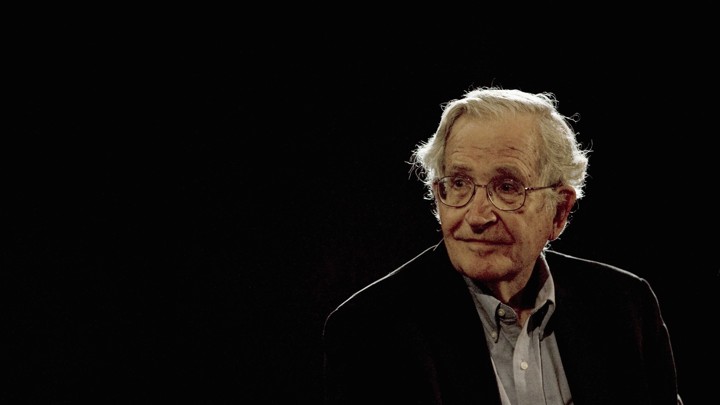
If we aim to make a list of the greatest and most unattainable intellectual tasks, then the task of “decoding” ourselves - understanding the internal structure of our minds and brains, and how the architecture of these elements is encoded in our genome - would definitely be at the top. However, the various areas of knowledge that were taken on this task, from philosophy and psychology to computer science and neuroscience, are overwhelmed by disagreement about which approach is correct.
In 1956, computer scientist John McCarthy introduced the phrase "artificial intelligence" (AI) to describe the science of studying the mind by recreating its key features on a computer. Creating a smart system using hand-made equipment, instead of our own “equipment” in the form of cells and tissues, should have become an illustration of complete understanding, and entail practical applications in the form of smart devices or even robots.
However, some of McCarthy’s colleagues from related disciplines were more interested in how the mind works in humans and other animals. Noam Chomsky and his colleagues worked on what later became known as cognitive science - the discovery of mental concepts and rules that underlie our cognitive and mental abilities. Chomsky and his colleagues overturned the dominant at that moment paradigm of behaviorism, led by Harvard psychologist B.F. Skinner, in which the behavior of animals was reduced to a simple set of associations between action and its effect in the form of encouragement or punishment. The shortcomings of Skinner's work in psychology became known from Chomsky’s 1959 criticism of his book Verbal Behavior, in which Skinner tried to explain language skills using behavioral principles.
Skinner's approach focused on the association between stimulus and animal response — an approach that is easily representable as an empirical statistical analysis that predicts the future as a consequence of the past. The concept of the Chomsky language, on the other hand, focused on the complexity of the internal representations encoded in the genome and their development in the course of obtaining data into a complex computer system that cannot be simply decomposed into a set of associations. The behavioral principle of associations could not explain the richness of language knowledge, our infinitely creative use of it, or why children quickly learn it from the minimal and noisy data that the environment provides them. “Language competence,” as Chomsky called it, was part of the body’s genetic makeup, as was the visual system, the immune system, the cardiovascular system,
David Marr, a specialist in neuroscience — Chomsky’s MIT colleague — identified a common approach to studying complex biological systems (such as the brain) in his sensational book, "Vision," and Chomsky’s language competence analysis more or less fits this approach. According to Marr, a complex biological system can be understood at three different levels. The first level (“computational level”) describes the system input and output, which define the task performed by the system. In the case of the visual system, the input can be an image projected on our retina, and the output can be the identification of objects in the image by our brain. The second level (“algorithmic level”) describes the procedure by which input turns into output, that is: how the image on our retina can be processed to achieve the task, described at the computational level. Finally, the third level (“implementation level”) describes how our biological equipment from cells performs the procedure described at the algorithmic level.
Chomsky and Marr’s approach to understanding how our mind works is as far from behaviorism as possible. Here, the emphasis is on the internal structure of the system, which allows it to accomplish a task, rather than on an external association between the past behavior of the system and the environment. The goal is to penetrate the “black box” that controls the system and describe its internal structure, something like a programmer can explain to you the principle of how a well-developed software product works, and also instruct how to run it on a home computer.
As is now commonly believed, the history of cognitive science is the history of Chomsky’s apparent victory over Skinner’s behavioral paradigm — an event often referred to as a “cognitive revolution”, although Chomsky himself denies such a name. This accurately reflects the situation in cognitive science and psychology, but in other related sciences, behavioral thinking is not going to die. Behaviorist experimental paradigms and associationist explanations of animal behavior are used by neuroscience specialists, whose goal is to study the neurobiology of laboratory animal behavior, such as rodents, where the three-level system approach proposed by Marr is not applicable.
In May 2011, in honor of the 150th anniversary of the Massachusetts Institute of Technology, a symposium “Brains, Minds and Machines” (Brains, Minds and Machines) was held, at which leading informatics scientists, psychologists and neuroscience specialists gathered to discuss past and future artificial intelligence and its connection to neuroscience.
The implication was that the meeting would inspire everyone with interdisciplinary enthusiasm for reviving the scientific question from which the whole field of artificial intelligence grew: How does the mind work? How did our brain create our cognitive abilities, and can it ever be embodied in a machine?
Noam Chomsky, speaking at the symposium, was not enthusiastic. Chomsky criticized the AI sphere for adopting an approach similar to behaviorism, only in a more modern, computationally complex form. Chomsky said that reliance on statistical techniques for finding patterns in large volumes of data is unlikely to give us the explanatory guesses that we expect from science. For Chomsky, a new AI — focused on using statistical training techniques for better data processing and making predictions based on them — is unlikely to give us general conclusions about the nature of rational beings or how thinking is arranged.
This criticism evoked Chomsky’s detailed response from Google’s research director and renowned AI researcher, Peter Norvig, who defended the use of statistical models and argued that the new AI methods and the definition of progress were not far from what was happening. and in other sciences.
Chomsky replied that the statistical approach may be of practical value, for example, for a useful search engine, and it is possible with fast computers that can process large amounts of data. But from a scientific point of view, Chomsky believes, this approach is inadequate, or, speaking more strictly, superficial. We have not taught a computer to understand what the phrase "physicist Sir Isaac Newton" means, even if we can build a search engine that returns plausible results to users who enter this phrase there.
It turns out that there are similar disputes among biologists who are trying to understand more traditional biological systems. As the computer revolution opened the way to the analysis of large amounts of data, on which the whole “new AI” holds, so the sequencing revolution in modern biology gave rise to flowering fields of genomics and system biology. High-throughput sequencing, a technique by which millions of DNA molecules can be quickly and cheaply read, has transformed the genome sequencing from an expensive 10-year-long enterprise into a laboratory procedure available to ordinary people. Instead of the painful study of separate isolated genes, we can now observe the behavior of a system of genes acting in cells as a whole, in hundreds, thousands of different conditions.
The sequencing revolution has just begun, and a huge amount of data has already been obtained, bringing with it excitement and new promising prospects for new therapies and diagnostics of human diseases. For example, when the usual medicine does not help a certain group of people, the answer may be in the genome of patients, and there may be some feature that does not allow the medicine to work. When enough data has been gathered to compare the relevant features of the genome in such patients, and the control groups are correctly selected, new customized medicines may appear leading to something like “personalized medicine”. It is understood that if there are sufficiently developed statistical tools and a sufficiently large data set, interesting signals can be pulled out of the noise,
The success of such phenomena as personalized medicine and other consequences of the sequencing revolution and the system-biological approach is based on our ability to work with what Chomsky calls the “mass of raw data” —and this puts biology at the center of the discussion, like the one in psychology and artificial intelligence since the 1960s.
Systems biology also met with skepticism. The great geneticist and Nobel laureate Sydney Brenner once defined it: “low input, high throughput, no output science” (loosely translated: “much ado about nothing, and no science at the end”). Brenner, the same age as Chomsky, who also participated in that symposium on AI, was just as skeptical about the new systemic approaches to understanding the brain. Describing a popular systems approach to mapping brain circuits, called Connectomics, which attempts to describe the connections of all neurons in the brain (that is, makes a diagram of how some nerve cells are connected to others), Brenner called it a "form of madness."
The ingenious attacks of Brenner on systems biology and related approaches in neuroscience are not far from Chomsky’s criticism in the direction of AI. Unlike externally, systems biology and artificial intelligence encounter the same fundamental task of reverse engineering of a highly complex system, whose internal structure is mostly a mystery. Yes, emerging technologies provide a large array of data related to the system, of which only a fraction can be relevant. Should we rely on powerful computational capabilities and statistical approaches to isolate the signal from noise, or should we look for more basic principles underlying the system and explaining its essence? The desire to collect more data is unstoppable, although it is not always clear what theory this data can fit into. These discussions raise the eternal question of the philosophy of science: What makes a scientific theory or explanation satisfactory? How is success determined in science?
We sat with Noam Chomsky on a April afternoon in a rather messy conversation, hiding in a secret corner of Frank Gehry’s dizzying Static Center MIT building. I wanted to better understand Chomsky’s criticism of artificial intelligence, and why, he believes, he is moving in the wrong direction. I also wanted to explore the application of this critique to other scientific areas, such as neuroscience and systems biology, which all work with the task of reverse engineering complex systems — and where scientists often find themselves in the midst of an infinitely expanding sea of data. Part of the motivation for the interview was that Chomsky is now rarely asked about science. Journalists are too interested in his opinion on US foreign policy, the Middle East, the Obama administration, and other common topics. Another reason was that Chomsky belongs to that rare and special kind of intellectuals that is rapidly dying out. Since the publication of the famous essay by Isaiah of Berlin, various thinkers and scholars began to put Fox-Hedgehog as a favorite entertainment in the academic environment: Hedgehog - meticulous and specialized, aimed at consistent progress in clearly defined framework, against Fox, more rapid, driven by ideas thinker who jumps from question to question, ignoring the scope of the subject area and applying his skills where they apply. Chomsky is special because he makes this distinction into an old and unnecessary cliché. Chomsky has no depth in return for flexibility or breadth, although, for the most part, he devoted his entire early scientific career to the study of certain topics in linguistics and cognitive sciences.
I want to start with a very simple question. At the dawn of artificial intelligence, people were optimistic about progress in this area, but everything turned out differently. Why is the task so difficult? If you ask specialists in the field of neuroscience why it is so difficult to understand the brain, they will give you absolutely unsatisfying answers: there are billions of cells in the brain, and we cannot read them all, and so on.
Chomsky:There is something in it. If you look at the development of science, all sciences are like a continuum, but they are divided into separate areas. The greatest progress is achieved by science, which studies the simplest systems. Take, for example, physics — there is tremendous progress in it. But one of the reasons is that physicists have an advantage that no other science has. If something gets too complicated, they pass it on to someone else.
For example, chemists?
Chomsky: If the molecule is too big, you give it to the chemists. Chemists, if for them the molecule is too large or the system becomes too large, give it to biologists. And if it’s too big for them, they give it to psychologists, and in the end it ends up in the hands of literary critics, and so on. So not everything that is said in neuroscience is completely wrong.
But maybe - and from my point of view it is very likely, although neuroscience doesn’t like it - that neuroscience has been on the wrong path for the last couple of hundred years. There are quite a new book very good neyrouchonogo the cognitive Randy Gallistela together with Adam King ( «the Memory and the Computational Brain: Why the Cognitive Science will of the Transform Neuroscience» - ca. Perevi.. ) In which he claims - in my view, plausibly - that neuroscience developed, being fascinated by associationism and related ideas about how people and animals work. As a result, they were looking for phenomena with associative psychology properties.
How with hebbov plasticity? [Theory attributed to Donald Hebb: associations between environmental stimuli and stimulus responses can be encoded by enhancing synaptic connections between neurons - approx. Ed.]
Chomsky:Yes, as the strengthening of synaptic connections. He spent years trying to explain: if you want to study the brain properly, you need, like Marr, to first ask what tasks he performs. Therefore, he is mainly interested in insects. So, if you want to study, say, the ant's neurology, you ask, what does an ant do? It turns out that ants do quite complex things, such as building a path. Look at the bees: their navigation requires quite complex calculations, including the position of the sun, and so on. But in general, with which he argues: if you take the cognitive abilities of an animal or person, these are computing systems. So you need to look at computational atomic units. Take the Turing machine, this is the simplest form of calculation, you need to find atoms that have “read” properties, "Write" and "address". These are minimal computational units, so you need to look for them in the brain. You will never find them if you look for the enhancement of synaptic connections or the properties of fields, and so on. You need to start with the following: see what is already there and what works, and you can see it from the highest level in the Marr hierarchy.
True, but most neuroscientists do not sit and do not describe the inputs and conclusions of the phenomenon they are studying. Instead, they put the mouse in a laboratory learning task, and record as many neurons as possible, or find out if the X gene is necessary for learning the task, and so on. Such assertions stem from their experiments.
Chomsky: Is that so ...
Is there a conceptual mistake in this?
Chomsky: Well, you can get useful information. But if there really is some kind of calculation that involves atomic units, you will not find them in this way. It's about how to look for lost keys under a different lantern, just because it’s lighter there (a reference to a well-known joke - approx. Transl.). This is a debatable question ... I do not think that the position of Gallistel was widely accepted by neurobiologists, but this is a plausible position, and it was made in the spirit of Marr’s analysis. So when you study vision, he says, you first ask what computational problems the system solves. Then you are looking for an algorithm that could perform these calculations, and in the end you are looking for mechanisms that allow you to make such an algorithm work. Otherwise, you can never find anything. There are many examples of this, even in the exact sciences, and most certainly in the humanities. People try to study what they know how to study - I mean, it looks reasonable. You have certain techniques of experiments, you have a certain level of understanding, you are trying to push the boundaries of the possible - and this is good, I do not criticize, people do what they can. On the other hand, It would be nice to know if you are moving in the right direction. And it may happen that if you take the point of view of Marra-Gallestel, which I personally sympathize with, then you will work differently, look for experiments of another kind.
So, I think the key idea of Marr is, as you said, to find suitable atomic units to describe the problem, in other words, the appropriate "level of abstraction", if you can say so. And if we take a concrete example of a new neuroscience area called Connectomics, where the goal is to find a diagram of the connections of very complex organisms, to find the connections of all the neurons of the cortex of a person’s brain or mouse. This approach was criticized by Sydney Brenner, who is to a large extent [historically] one of its authors. Advocates of this area do not stop and do not ask whether the diagram of links is a suitable level of abstraction — it may not be. What is your opinion about this?
Chomsky: There are much simpler questions. For example, here at MIT, there was an interdisciplinary program for studying nematodes (roundworm - approx. trans. ) C. elegans for several decades, and, as I understand it, even with this tiny creature that you know the whole diagram of connections, there are 800 neurons or so ...
I think 300 ..
Chomsky: ... Anyway, you are not you can predict what it is [nematode C. elegans] is going to do. Maybe you're just looking for the wrong place.

I would like to go on the topic of various methodologies in AI. So, “Good old artificial intelligence” (GOFAI), as it is called now, was based on strict formalisms in the tradition of Gottlob Frege and Bertrand Russell, on mathematical logic, for example, or its branches, as non-monotonic reasoning, and so on. From the point of view of the history of science, it is interesting that these approaches were almost completely excluded from the mainstream and were replaced - in the field that now calls itself AI - probabilistic and statistical models. My question is: how can this shift be explained, and is this a step in the right direction?
Chomsky: I listened to Pat Winston's report on this a year ago. He had one of the theses: AI and robotics have reached a stage where you can do really useful things, so attention turned to practical application, and therefore more fundamental scientific questions were put aside, simply because everyone is caught up in the success of technology and achieving certain goals.
That is, everything went into engineering ...
Chomsky: Yes, that is true ... And this is quite understandable, but, of course, it leads people away from the initial questions. I myself have to admit that I was very skeptical about these original works ( in the new paradigm of probabilistic AI - approx. Transl. ). It seemed to me that everything was too optimistic, it was assumed that you could achieve results that require real understanding of barely studied systems, and that you cannot come to their understanding simply by throwing a complicated machine there. If you try to do this, you come to the concept of self-reinforcing success, because you get the result, but it is very different from how it is done in the sciences.
For example, take the extreme case, suppose that someone wants to abolish the physics department, and do it right. And the “right” is to take a lot of videos about what is happening in the outside world and feed them to the largest and fastest computer, gigabytes of data, and make a comprehensive statistical analysis - well, you know, Bayesian methods, back and forth. (modern approach to data analysis based on probability theory - ed.)- and you get something like a prediction about what happens outside your window the next second. In fact, you will receive a much better prediction than the physics department could give you. Well, if success is determined to get the closest approximation on a mass of chaotic raw data, then of course this is a much better way than physics usually does — well, you know, no more thought experiments on a perfectly flat surface, and so on . But you will not get the level of understanding that has always been the goal of science - you only get an approximation to what is happening.
And so it is done everywhere. Suppose you want to predict the weather for tomorrow. One way: OK, I have statistical a priori probabilities, for example: there is a high probability that tomorrow the weather will be the same as it was yesterday in Cleveland, and I use it, and the sun's position will have some influence, and this I also use, so , you made a few such assumptions, you conduct an experiment, you look at the results again and again, you correct using Bayesian methods, you get the best prior probabilities. You get a pretty good approximation of what the weather will be like tomorrow. But this is not what meteorologists do - they want to understand how it works. And these are just two different concepts of what success is, what achievement is. In my science, the science of language, it is very often. In computational cognitive science, applied to the language, the concept of success is this. That is, you are getting more and more data, better statistics, getting more and more accurate approximation to some kind of gigantic body of text, for example, all the Wall Street Journal archives - but you don’t learn anything about the language.
A completely different approach, which I think is right, is to try to see if you can understand what the fundamental principles and their connection with key properties are, and see that in real life thousands of different variables will interfere with you - like what is happening now for window - and you will deal with them later, if you want a more accurate approximation. These are just two different concepts of science. The second is what science has been since Galileo, it is modern science. Approximation of the raw data is like a new approach, but in fact, such things existed in the past. This is a new approach that is accelerated by the existence of large amounts of memory, very fast processing, which allow you to do things that you previously could not do manually. But I think,
... in engineering?
Chomsky:... But leads away from understanding. Yes, maybe even effective engineering. And this, by the way, is interesting what happened with engineering. When I got to MIT in the 1950s, it was an engineering college. There was a very good faculty of mathematics, physics, but they were servicing faculties. They trained engineers for all sorts of tricks they could use. At the Faculty of Electronic Engineering, you studied how to assemble a circuit. But since the 1960s and to this day, everything is completely different. No matter what your engineering specialty is, you study all the same basic sciences and mathematics. And then maybe you are learning a little about how to apply it. But this is a completely different approach. It became possible due to the fact that for the first time in the history of mankind, the basic sciences, like physics, could really help engineers. Besides, Technologies began to change very quickly, so it makes little sense to study the technologies of today, if after 10 years they change anyway. Therefore, you are studying a fundamental science that will be applicable, no matter what happens next. And about the same thing happened in medicine. So, in the last century, again, for the first time, biology had something to say about practical medicine, and therefore you had to know biology, if you wanted to become a doctor, plus the technologies changed. I think that this is a transition from something like art that you learn to use - the analogy would be a comparison of incomprehensible data in some special way, and maybe even building something working - a transition to science, which appeared in modern times. , roughly speaking, the science of Galileo. if after 10 years they change anyway. Therefore, you are studying a fundamental science that will be applicable, no matter what happens next. And about the same thing happened in medicine. So, in the last century, again, for the first time, biology had something to say about practical medicine, and therefore you had to know biology, if you wanted to become a doctor, plus the technologies changed. I think that this is a transition from something like art that you learn to use - the analogy would be a comparison of incomprehensible data in some special way, and maybe even building something working - a transition to science, which appeared in modern times. , roughly speaking, the science of Galileo. if after 10 years they change anyway. Therefore, you are studying a fundamental science that will be applicable, no matter what happens next. And about the same thing happened in medicine. So, in the last century, again, for the first time, biology had something to say about practical medicine, and therefore you had to know biology, if you wanted to become a doctor, plus the technologies changed. I think that this is a transition from something like art that you learn to use - the analogy would be a comparison of incomprehensible data in some special way, and maybe even building something working - a transition to science, which appeared in modern times. , roughly speaking, the science of Galileo. And about the same thing happened in medicine. So, in the last century, again, for the first time, biology had something to say about practical medicine, and therefore you had to know biology, if you wanted to become a doctor, plus the technologies changed. I think that this is a transition from something like art that you learn to use - the analogy would be a comparison of incomprehensible data in some special way, and maybe even building something working - a transition to science, which appeared in modern times. , roughly speaking, the science of Galileo. And about the same thing happened in medicine. So, in the last century, again, for the first time, biology had something to say about practical medicine, and therefore you had to know biology, if you wanted to become a doctor, plus the technologies changed. I think that this is a transition from something like art that you learn to use - the analogy would be a comparison of incomprehensible data in some special way, and maybe even building something working - a transition to science, which appeared in modern times. , roughly speaking, the science of Galileo.
Clear. Returning to the topic of Bayesian statistics in models of language and cognition. There was a famous dispute with your participation, you argued that talking about the likelihood of a proposal is unwise in itself ...
Chomsky: ... Well, you can get a number if you want, but it means nothing.
It means nothing. But it seems that there is an almost trivial way to unify the probabilistic method, assuming that there are very rich internal mental representations consisting of rules and other symbolic structures, and the goal of the theory of probability is simply to link the noisy, fragmentary data of our world with these internal symbolic structures. And you are not required to say something about how these structures appeared - they could exist initially, or if some parameters are adjusted there - depends on your concept. But probability theory works like a glue between noisy data and very rich mental notions.
Chomsky: There is nothing wrong with probability theory, statistics.
But does she have a role?
Chomsky:If you can use it, fine. But the question is, what are you using it for? First of all, the very first question, is there any point in understanding the noisy data? Does it make sense to understand what is happening outside, outside the window?
But we are bombarded with this data. This is one example of Marr: we encounter noisy data constantly, starting from our retina and up to ...
Chomsky:This is true. But here is what he says: Let's ask ourselves how the biological system selects from the noise important. Retina is not trying to duplicate incoming noise. She says: I will now look for this in the image, this and this. This is about language learning. The newborn baby is surrounded by various noises, as William James said, “a blooming and buzzing mess.” If a monkey, a kitten, a bird, anyone, hears this noise, that's where it ends. However, the child in some way, immediately, reflexively, chooses from the noise a separate part, which is connected with the language. This is the first step. How he does it? Not with statistical analysis, because the monkey can also roughly perform the same probabilistic analysis. He is looking for a specific thing. So, psycholinguists, neuro-linguists and others are trying to uncover specific details of the computing system and neuropsychology that are somehow related to specific aspects of the environment. So, it turns out that there really are neural circuits that react to certain types of rhythm, which manifests itself in the language - like the length of syllables and so on. And there is some evidence that one of the first things that a child’s brain is looking for is rhythmic structures. And going back to Gallistel and Marr, the brain has some kind of computing system inside that says: “Okay, this is what I’ll do with these things,” and after about nine months a typical child has already eliminated — removed from his supply — those phonetic differences, which is not in its own language. That is, it turns out, from the very beginning, any child is tuned to any language. But let's say A Japanese child at the age of nine months will not react to the difference between “P” and “L”, it is sort of dropped out. So the system considers a lot of possibilities and limits them only to those that are part of the language, and this is already quite a narrow set. You can come up with an anti-language in which the child can never do it, and much more interesting. For example, if we talk about the more abstract side of the language, at the moment there is solid evidence that such a simple thing as the linear word order — what follows it — is not part of the syntactic and semantic computing systems, their design is simply such that they are not looking for a linear order. It can be seen that more abstract concepts of distance are predominantly used, and this is not a linear distance, and neurophysiological confirmation can be found for this. We can give an example: if you come up with an artificial language in which the linear word order is used, as, for example, you make a negative sentence with an affirmative sentence, doing something with the third word in a row. People will be able to solve this puzzle, but apparently the standard language areas of the brain are not activated - other areas are activated, that is, people perceive it as a puzzle, and not as a language task. And to solve it, people have to strain more ... that is, people perceive it as a puzzle, and not as a language task. And to solve it, people have to strain more ... that is, people perceive it as a puzzle, and not as a language task. And to solve it, people have to strain more ...
You consider this to be a convincing evidence that activation or lack of activation of a brain area ...
Chomsky: ...This is evidence, and of course, you want more. But this evidence is such that you see from the linguistic side how languages work — there are no such things in them as the third word in a sentence. Take a simple sentence: “Instinctively flying eagles swim,” here “instinctively” is associated with the word “swim,” and not with the word “flying,” even though the entire sentence does not make sense. And here is the action of the reflex. “Instinctively,” adverb, does not search for the nearest verb, it searches for a structurally more suitable verb. This is a much more complicated calculation. But this is the only calculation that is generally used. Linear order is a very simple calculation, but it is never used. There is a lot of evidence like this, and very little neuro-linguistic evidence, but they point in the same direction.
This, in my opinion, is a way to understand how the system actually works, as it happened with the vision system in Marr's laboratory: people like Shimon Ulman discovered quite remarkable things like the principle of rigidity. You can’t find it with statistical data analysis. He found this through carefully planned experiments. Then you look in neurophysiology and see if you can find something that performs these calculations. I think the same thing in language, the same thing in the study of our arithmetic abilities, planning, almost everywhere. Just working with raw data - you will not come anywhere with it, and Galileo would not have come. In fact, if you come back to this, in the 17th century it was not easy for people like Galileo and other great scientists to convince the National Science Foundation of those times - namely, aristocrats - that their work had a meaning. I mean: why study how the ball rolls on a perfectly flat plane without friction, because they do not exist. Why not study how flowers grow? If you tried to study the growth of flowers in those times, you would probably get a statistical analysis of how everything works.
It is important to remember that in cognitive science we are still in the pre-Galilean era, we are just starting to make discoveries. And I think that one can learn something from the history of science. One of the main experiments in the history of chemistry in 1640 or so, when someone proved, to the pleasure of the entire scientific world up to Newton, that water can be turned into living matter. That's how they did it - of course, no one knew anything about photosynthesis - they took a lot of land, and heated it so that all the water evaporated. It was weighed, a willow branch was inserted into it, and poured over with water, measuring the volume of this water. When everything is ready and the willow tree has grown, you again take the earth and evaporate water from it - just as before. Thus, you have shown that water can turn into an oak or something else. This is an experiment, and it seems to be even true, but you do not know what you are looking for. And it was not known until Priestley discovered that air is a component of the world, there is nitrogen in it, and so on, and you learned about photosynthesis and so on. Then you can repeat the experiment and understand what is happening. But you can easily be led away in the wrong direction by an experiment that seems successful because you do not understand well what you should be looking for. And you will go even further in the wrong direction if you try to study the growth of trees like this: just take an array of data on how trees grow, feed it to a powerful computer, conduct a statistical analysis and get an approximation of what happened. But you can easily be led away in the wrong direction by an experiment that seems successful because you do not understand well what you should be looking for. And you will go even further in the wrong direction if you try to study the growth of trees like this: just take an array of data on how trees grow, feed it to a powerful computer, conduct a statistical analysis and get an approximation of what happened. But you can easily be led away in the wrong direction by an experiment that seems successful because you do not understand well what you should be looking for. And you will go even further in the wrong direction if you try to study the growth of trees like this: just take an array of data on how trees grow, feed it to a powerful computer, conduct a statistical analysis and get an approximation of what happened.
In biology, do you assess Mendel’s work as a successful example of how to get noisy data — it’s important that the numbers are — and jump to the postulation of a theoretical object ...
Chomsky: ... And throwing out a huge amount of data that did not work.
... But after seeing the relation that made sense, develop a theory.
Chomsky:Yes, he did everything right. He allowed the theory to manage data. There were more data that contradicted the theory, which were more or less discarded, well, you understand - which usually will not be included in the article. And he, of course, talked about things that no one could find, how it was impossible to find the units, the existence of which he argued. But yes, that's how science works. Also in chemistry. Chemistry, before my childhood, not so long ago, was considered as the science of computing. Because it can not be reduced to physics. Therefore, it is just a way to calculate the result of experiments. Atom Bora so perceived. The way to calculate the result of experiments, but this cannot be a real science, because it cannot be reduced to physics, and suddenly, it turned out to be true, because physics was wrong. When quantum physics appeared, it became possible to combine it with no change in chemistry. That is, the whole project with the reduction was simply wrong. The correct project was to see how you can combine these two world views. And it turned out that, surprise - they were united by radical changes in the downstream science. Maybe exactly the same thing with psychology and neuroscience. I mean, neuroscience is not even nearly as advanced now as physics a century ago.
And it will be a departure from the reductionist approach with the search for molecules ....
Chomsky: Yes. In fact, the reductionist approach has been wrong several times. The unification approach makes sense. But the unification may differ from the reduction, since there may be a flaw in the basic science, as in the case of physics and chemistry, and I suspect with a high degree of probability the same in the case of neuroscience and psychology. If Gallistel is right, then it would make sense to say that yes, they can be combined, but with a different approach to neuroscience.
Should we strive for speedy unification, or is it better to continue developing these areas in parallel?
Chomsky:Unification is such an intuitive pursuit of an ideal, part of scientific mysticism, if you like. It is like a search for a general theory of the world. Maybe it does not exist, maybe different parts work differently, but there is an assumption, until I was given a convincing refutation, my assumption is that there is a general theory of the world, and my task is to try to find it. Unification may not manifest itself through reduction, and often it happens. This is the leading logic of David Marr's approach: what you discover at the computational level should be combined with what you once find at the level of mechanisms, but maybe not in the terms in which we now understand these mechanisms.
And Marr implies that you cannot work at all three levels in parallel [computational, algorithmic and implementation level], you need to move from top to bottom, and this is a very strict requirement, given that science is usually not the case.
Chomsky: He could not say such that everything should be tough. For example, discovering something new about mechanisms may lead you to change the concept of computing. The logical order does not necessarily coincide with the order of research, since in research everything happens at the same time. But I think that in a rough approximation, the picture is correct. Although I must say that Marr's concept was developed for input systems ...
Information processing systems ...
Chomsky:Yes, like sight. There is data - this is a data processing system - and something happens in it. And this does not work well for cognitive systems. Take your ability to do arithmetic ...
She's very weak, but okay ...
Chomsky: Good [laughs]. But this is an internal ability, you know that your brain is a control node of something like a Turing machine, and it has access to external data, such as memory, time ... Theoretically, you can multiply anything, but in practice, of course , not this way. If you try to learn what your internal system is, then Marr's hierarchy does not work very well. You can talk about the computational level: maybe the rules are inside me, these are Peano's axioms . ed.: The mathematical theory (named after the Italian mathematician Giuseppe Peano), describing the core of the basic rules of arithmetic and natural numbers, from which you can derive a lot of useful arithmetic facts] or something else, it does not matter - this is a computational level. Theoretically, although we do not know how, you can only talk about the neurophysiological level, nobody knows how, but there is no real algorithmic level. Because there is no calculus of knowledge, it is just a system of knowledge. It is not clear how to understand the nature of the system of knowledge: there is no algorithm there, because there is no process there. This can be done only by using a knowledge system in which there is a process, but this will already be something completely different.
But since we make mistakes, does this mean that the process is going wrong?
Chomsky:This is the process of using the internal system. But the internal system itself is not a process, since it does not have an algorithm. Take the usual math. If you take Peano's axioms and the rules of inference, they define all arithmetic calculations, but there is no algorithm there. If you ask how a number theory specialist applies them, there are, of course, many options: for example, you start not with axioms, but with the rules of inference. You take a theorem and see if it is possible to derive a lemma, and if it works, then see if it turns out to base this lemma on something, and at the end you get a proof - a geometric object.
But this is a fundamentally different activity, different from the addition of small numbers in my head - and of course, I have some sort of algorithm in my head.
Chomsky:Not necessary. There is an algorithm for this process in both cases. But there is no algorithm of the system itself, this is a categorical error. You are not asking what process defines Peano's axioms and the rules of inference; there is no process there. There may be a process to use them. And this can be a complicated process, and this is true of your calculations. The internal system that you have - here the question of the process does not arise. But if you use your internal system, the question arises, and you can perform multiplication in different ways. For example, if you add 7 and 6, one algorithm says: "I will see how much it takes to get to 10" - it takes 3, and now there are 3 more left, so I will move from 10 and add 3 more, and 13. This is an addition algorithm - in fact, as I was taught in kindergarten. This is one way to add numbers.
But there are other ways to add - there is no correct algorithm. These are the algorithms for performing the cognitive system process in your head. And now for this system you do not ask about the algorithms. You can ask about the computing level, about the level of mechanisms. But the algorithmic level for this system does not exist. The same with the language. Language is like arithmetic ability. There is a system that determines the sound and meaning of an infinite array of possible sentences. But there is no question about what kind of algorithm is there. There is also no question of what formal arithmetic system tells you how to prove theorems. Using the system is a process, and you can study it in terms of Marr levels. But it is important to conceptualize these differences.
It just seems like an incredible task to move from the theory of computational level, like Peano's axioms, to Marrow level 3 ...
Chomsky: mechanisms ...
... mechanisms and realizations ...
Chomsky: Yes. And ...
... without an algorithm, at least.
Chomsky:I think this is wrong. Maybe the information on how the system is used and tell you something about the mechanisms. But some higher intelligence - maybe higher than ours - will see that there is an internal system, and it has a physiological basis, and it will be possible to study this physiological basis. Even without looking at the process in which this system is used. Maybe watching the process gives you useful information about where to go. But conceptually this is another task. The question is which way to conduct research is better. So, maybe the best way to study the connection between Peano's axioms and neurons is to observe how mathematicians prove theorems. But this is only because I will give you supporting information. The real end result will be an understanding of the brain system, its physiological basis, without reference to any algorithm. Algorithms are all about the processes that use them, and they can help you get answers. Perhaps, as inclined surfaces can tell you something about the speed of falling, but if you look at the laws of Newton, they say nothing about inclined planes.
Good. The logic of studying cognitive and language systems using the Marr approach is understandable, but since you do not recognize language competence as a genetic feature, you can apply this logic to other biological systems — the immune system, the cardiovascular system ...
Chomsky: Exactly, I think that is very similar. You can say the same about the immune system.
And it may even be easier to do this with these systems than with thinking.
Chomsky:But you will expect other answers. You can do this with the digestive system. Suppose someone is studying the digestive system. It is unlikely that he will study what happens when you have gastric flu, or when you have eaten a Big Mac, or something else. Let's return to photographing what is happening outside the window. One way to study the digestive system is to collect all sorts of data about what the digestive system does in various circumstances, enter data into a computer, conduct a statistical analysis - you will get something. But it will not be what the biologist does. He wants from the very beginning to abstract from what is considered - perhaps falsely, because you can always make a mistake - irrelevant variables, like if you have gastric flu.
But this is exactly what biologists do: they take sick people with a sick digestive system, compare them with healthy ones, and measure molecular properties.
Chomsky: They do it at a more advanced stage. They already know a lot about the structure of the digestive system before comparing patients. Otherwise they will not know what to compare, and why one is sick and the other is not.
They rely on statistical analysis to identify the distinctive features. This is a very well-funded approach, because you declare that you study patients.
Chomsky:This may well be a way to obtain funding. This is how to seek funding for linguistics that this may help to treat autism. This is another question altogether [laughs]. But the search logic is to start exploring the system, abstracting from what you, with a high degree of probability, consider irrelevant noise. You are trying to find a basic entity, and then you wonder what happens if we bring something else, the same stomach flu.
It still seems that there is a difficulty in applying Marr levels to systems of this type. If you ask what kind of computational problem the brain solves, then there seems to be an answer, it works approximately like a computer. But if you ask what kind of computational problem the lung solves, it’s even hard to think about it - this is obviously not the task of processing information.
Chomsky: It is, but there is no reason to believe that all biology is computational. There may be reasons to think that thinking is such. And in fact, Gallistel does not say that everything that is in the body should be studied through the search for read / write / address units.
It just seems counterintuitive in terms of evolution. These systems evolved together, reusing similar parts, molecules, trajectories. Cells are computing devices.
Chomsky:You do not study the lung by asking questions about what cells calculate. You study the immune system and the visual system, but you do not expect to find the same answer. The organism is a highly modular system, it has many complex subsystems that are more or less internally combined. They act according to different laws. Biology is also modular. You can not assume that this is all just a huge mess of objects that behave the same way.
Of course not, but I mean that one could apply the same approach to study each of the modules.
Chomsky: Not necessarily, because the modules are different. Some of the modules may be computational, some may not.
Do you think an adequate theory that would have explanatory, rather than just predictive power through statistics ... What could be an adequate theory of these systems that are not computational - can we understand them at all?
Chomsky:Of course. You can understand a lot about, for example, what causes an embryo to turn into a chicken, and not, say, a mouse. This is a very confusing system, including all kinds of chemical interactions and other things. Even with a nematode, the fact that everything is determined simply by the neural network is completely unclear, and there is research data on this matter. You need to look at the complex chemical interactions that occur in the brain, in the nervous system. It is necessary to peer into each system separately. These chemical interactions may not be related to your arithmetic abilities - most likely so. But they can very easily be connected with whether you decide to raise your hand or lower it.
Although, if you begin to study chemical interactions, it will lead you to what you call the repeated description of the phenomenon, only in other words.
Chomsky: Or for an explanation. Because perhaps they are very important, critical, connected.
But if you make an explanation in terms of "substance X must be activated" or "gene X must be present", you really do not explain how the body works. You just found the lever and click on it.
Chomsky: But then you look further, and find what makes this gene work like that in such conditions, or work differently in other conditions.
But if genes are the wrong level of abstraction, then you are in flight.
Chomsky:Then you do not get the right answer. Or maybe not. For example, it is well known that it is difficult to calculate how an organism develops from the genome. There are various kinds of processes taking place in the cell. If you just look at the action of a gene, you may be at the wrong level of abstraction. It is never clear, therefore, it is necessary to study it. I do not think that there is an algorithm for answering such questions.
I would like to shift the conversation towards evolution. You criticized a very interesting point of view, which you called "phylogenetic empiricism." You criticized this position for lack of explanatory power. She simply states the following: so, thinking is what it is, because such adaptations to the environment were chosen. Selected by natural selection. You argued that this explains nothing, because you can always appeal to these two principles - mutation and selection.
Chomsky: Well, you can also give up on them, but they may be right. It may happen that the development of your arithmetic abilities has grown out of random mutations and selection. If it turns out to be so, well, fine.
It sounds like a truism ( well-known truth - approx. Transl. ).
Chomsky: And I'm not saying that this is wrong. Truisms are true [laughs].
But they do not explain.
Chomsky:Maybe this is the highest level of explanation you can get. You can invent a world - I do not think it will be our world - but you can invent a world in which nothing happens except random changes in objects and selection based on external forces. I do not think that our world is like this, and I do not think that there is at least one biologist who thinks so. There are many ways in which natural forces determine the channels through which selection can take place, some things happen, some don't. Very many things in the body do not work that way. Take at least the first step, meiosis: why cells are divided into spheres, and not cubes? These are not random mutations or natural selection: they are the laws of physics. There is no reason to think that the laws of physics stop here, they work everywhere.
Yes, of course, they limit biology.
Chomsky: Okay, that is, it's still not just random mutations and selection. These are: random mutations, selection and everything else that matters, for example, the laws of physics.
Is there a place for these approaches, which are now called "comparative genomics"? The Broadau Institute here [at MIT / Harvard] creates large amounts of data from different genomes from different animals, from different cells in different circumstances, and sequences any molecule that is possible. Is there something that can be learned about high-level cognitive tasks from these comparative evolutionary experiences, or is it an immature approach?
Chomsky: I'm not saying that this is the wrong approach, but I do not know what can be learned from this. Like you.
Do you have examples in which this evolutionary analysis reported something important? For example, mutations Foxp2? [ Approx. Ed: A gene that was thought to be associated with speech or language abilities. In families with typical speech disorders, genetic mutations have been discovered that destroy this gene. It has several mutations that are unique to different stages of human evolution.]
Chomsky:Foxp2 is interesting, but it has nothing to do with the language. It is associated with fine motor skills and similar things. This is related to the use of language, for example, when you say that you control your lips and so on, but this is very peripheral with regard to the language, and this is already known. So, for example, if you use articulating organs or signs, well, for example, a hand gesture is the same language. In fact, it is even analyzed and produced in the same part of the brain, although in one case the arms move, in the other the lips. So whatever the externalization is, it's all on the periphery. I think it is rather difficult to talk about it, but if you look at the language structure, you will get evidence of this. There are interesting examples in language learning, where there is a conflict between computational efficiency and communicative efficiency.
Take this example, which I have already mentioned, with linear order. If you want to know to which verb an adverb is attached, the child reflexively uses the minimum structural distance, and not the minimum linear distance. Yes, it is simpler to use the minimum linear distance from the computational point of view, but for this it is necessary to have the notion of linear order. And if the linear order is only a reflex of the sensorimotor system, which seems reasonable, then it will not be. Here is evidence that the projection of the internal system onto the sensorimotor system is peripheral with respect to the operation of the computing system.
But can it be that the computing system introduces its limitations, how does physics limit meiosis?
Chomsky:Maybe, but there is no evidence. For example, the left end - the left one in the earlier sense - the sentences have different characteristics than the right one. If you want to ask a question, for example: “Whom do you see?” You put the word “Whom” at the beginning, not at the end. In fact, in any language in which the interrogation group — who, whose book — moves elsewhere, it moves to the left, not to the right. This is very likely a limitation of information processing. The offer begins with what the listener tells you: this is what I look like. If you were standing at the end, then you would have a completely declarative sentence, and only at the end would you know what information I ask you. If you say this, it is a limitation to the processing of information. So, if this is the case, then externalization affects the computational nature of syntax and semantics.
There are cases in which you find obvious conflicts between computational and communicative efficiency. Take a simple example: if I say “Visiting relatives can be a burden” - this is ambiguous. Do relatives visit you? Or are you going to visit relatives? It turns out that in every known case, ambiguity arises simply from the fact that we allow the rules to function freely, without restrictions. So it is computationally efficient, but inefficient for communication, because it leads to intractable ambiguity.
Or take the example of sentences with the effect of a garden path leading in the wrong direction. Proposals like: “The horse raced past the barn fell.” ( Horse, which was sent for the barn, fell down - approx. Transl.). People, when they see such a proposal, do not understand it, because it is built in such a way that takes you along the garden path. “The horse raced past the barn” sounds like a sentence, and then you are puzzled: what does the word “fell” do at the end? On the other hand, if you think about it, this is an absolutely correctly formed sentence. It means that the horse, which was directed by the barn by someone, fell. But the rules of the language, when they simply function, may give you incomprehensible sentences due to the phenomenon of the garden path.
And there are many such examples. There are things that you simply cannot say for some reason. If I say: the mechanics fixed the cars. And you will say: "They were interested in whether the mechanics of the machine were repaired." You can ask questions about the machines: “How many machines about which they were interested, did the mechanics repair them?” More or less possible. Suppose you want to ask a question about mechanics. “How many mechanics, they were interested, did they repair the cars?” For some reason it doesn’t work that way, it’s impossible to say so. This is a clear idea, but you cannot say it. If you study this case in detail, the most efficient computational rules prevent you from saying this. But for the expression of thoughts, for communication, it would be better if you could say that - hence the conflict.
And in fact, in each case of such a conflict, computational efficiency wins. Externalization is inferior in all cases of ambiguity, but just for computational considerations, it seems that the system inside does not worry about externalization. I may not be able to show that enough, but just if you say it out loud, it will be a convincing enough argument.
This tells us something about evolution. What is stated by this fact: in the course of the evolution of the language, the computing system developed, and only then it was externalized. And if you think about how a language could develop, you have almost come to this position. At some point of human evolution, and this is obvious, quite recently, if you look at the archaeological data - maybe in the last hundred thousand years ago, and this is just nothing - at some point a computing system appeared with new properties that were not in other organisms, such an arithmetic type of property ...
That is, it allowed you to think better before externalizing?
Chomsky:She gives you thinking. A small flashing of the brain that occurs in an individual, not in a group. That person had the ability to think, the group did not. So there is no sense in externalizing. Then, if this genetic change spreads, and suppose many people have it, then it makes sense to look for a way to project it onto the sensorimotor system, and this is externalization, but this is a secondary process.
Only if externalization and the internal system of thinking are not connected in an unpredictable way.
Chomsky:We do not predict, and this makes little sense. Why should she be connected to an external system? For example, your ability to arithmetic is not connected with it. And there are many other animals, such as songbirds, that have an internal computing system, a bird song. This is not the same system, but it is some kind of internal computing system. And it is externalized, but sometimes not. The chick in some species masters the song of this species, but does not reproduce it until maturity. In this early period he has a song, but there is no externalization system. The same is true of people: a human child understands much more than it can reproduce - quite a lot of experimental evidence - which indicates that the child has an internal system, but he cannot externalize it. Maybe he doesn't have enough memory
I would like to finish with one question about the philosophy of science. In a recent interview, you said that part of the problem is that scientists do not think very much about what they do. You mentioned that you taught philosophy in MIT, and people read, for example, Willard van Orman Quine, and it flew into one ear and into another flew off, and people returned to do their own science just as before. What important insights can be gleaned in the philosophy of science, the most relevant for biologists who want to give an explanatory theory, not a retelling of the phenomenon? What do you expect from such a theory, and what insights will help direct science in such a direction? Instead of leading the science towards behaviorism - an intuition that many neuroscientists are guided by.
Chomsky:The philosophy of science is a very interesting discipline, but I do not think that it really makes a contribution to science - it learns from science. She is trying to understand what the sciences are doing, why achievements are happening in them, which ways are wrong, can it be codified and understood. What I consider important in the history of science. I think we will learn a lot from the history of science of what may be very important for the developing sciences. Especially when we understand that in the cognitive sciences we are still in the pre-Galilean stage. We do not know that we are looking for something that Galileo has already found, and there is something to learn. For example, one startling fact from the early sciences, not necessarily from Galileo, but in general from the time of Galilean discoveries is that simple things can be very confusing. So I hold this cup, and if the water boils, steam will rise, but if I remove my hand, the cup will fall. Why does the cup fall and steam rises? For a thousand years in a row, this was a completely satisfactory answer: they aspire to their natural state.
How in Aristotle's physics?
Chomsky:This is Aristotelian physics. The best and greatest scientists believed that the answer was exactly that. Galileo allowed himself to doubt. As soon as you allow yourself to doubt, you immediately discover that your intuition is wrong. As a fall of a small mass and a large mass, and so on. All your intuitions deceive you - riddles everywhere you look. In the history of science there is something to study. Take the same example that I gave you, "instinctively flying eagles are swimming." No one ever thought it was a mystery. But if you think about it, it is very mysterious, you use complex calculations instead of simple ones. If you let yourself be surprised at this, like dropping a cup, you ask the question “Why?”, And then you get on the path of rather interesting answers. As, for example: linear order is not part of the computing system, which is an important assumption about the architecture of thinking - it says that the linear order is only part of the externalization system, that is, the secondary system. And this opens up a huge number of other ways.
Or take another example: the difference between reduction and unification. The history of science provides some very interesting illustrations in chemistry and physics, and I think they are quite relevant to the state of the cognitive and neurophysiological sciences of modern times.
Afterword of the translator: during the time that has passed since the interview, other interesting materials came out for Chomsky - you can offer to get acquainted with the 2.5-hour conversation with the American physicist Lawrence Krauss, or with the new book by Chomsky and Berwick “A Man Speaking” if you are interested evolution and language.
Translated by Tatyana Volkova.