Robots in journalism, or How to use artificial intelligence to create content
Machines are getting smarter. Already, they generate content of such quality that even a professional does not always distinguish it from “human.” About why journalists and editors should not be afraid of competition, and about the prospects for the automation of journalism at our conference " Content " said Sergey Marin from "Data Studio".
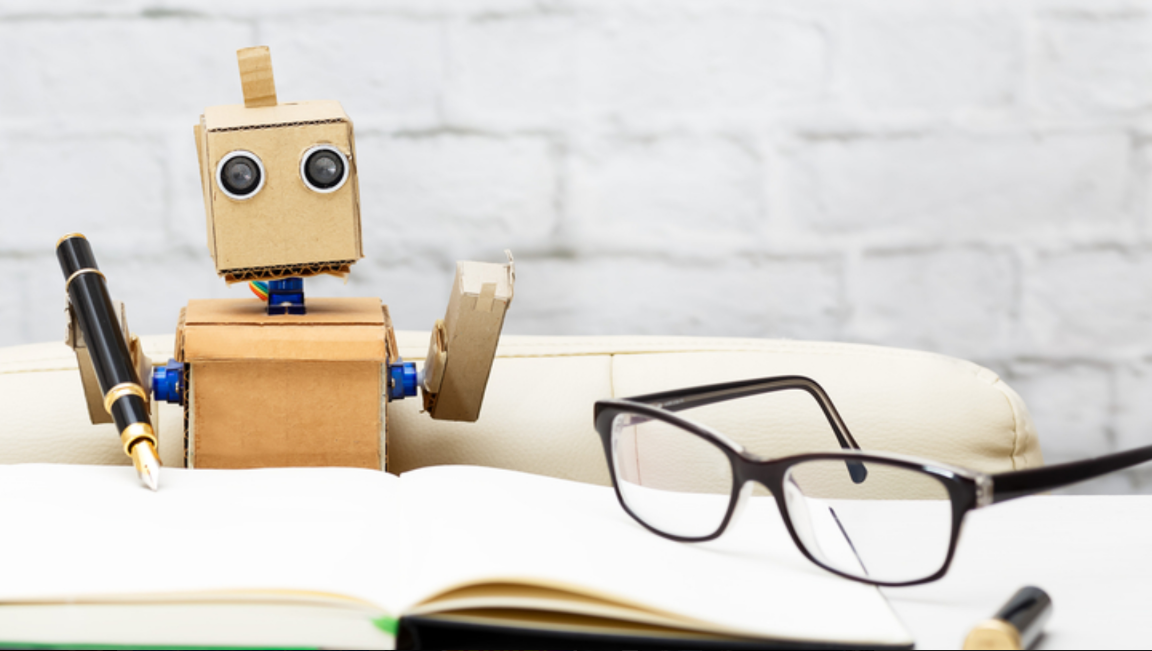
Under the cut the decoding of his report.
About Speaker
Sergey Marin is an expert in artificial intelligence, the director and founder of Data Studio .
If we are talking about artificial intelligence - in journalism or in any other field - you need, first of all, to understand its structure. The AI consists of three main components: machine learning, recommendation systems and neural networks. By the way, many people consider neural networks to be synonymous with artificial intelligence, but this is just one of the tools, not even the most popular: in each case, those algorithms that work most optimally are used.

Machine learning is used to search for hidden patterns in the data. Imagine that we have a set of infoprovodov or publications that need to be classified, that is, automatically assign them some tags. Or simply texts with a large number of words that need to be divided into certain classes, interests, moods, and so on. How do we do it? If we talk about machine learning, we are not looking for any keywords to draw conclusions based on them. Instead, we show the machine as much as possible of the texts we have already marked with a large number of classes. Then we give the new text, and the machine itself classifies it into the area to which it belongs. That is, we first teach, show many examples.

That is the main use of machine learning in journalism is classification. For example, we have a large number of information leads - from the Internet, social networks, news agencies - and we need to quickly classify them. We have trained our model in advance, and when we have a new information center, the machine understands where it belongs, what its subject matter, what mood it conveys, for which audience it can be applied. The popularity, the rating of some infopowder is predicted in the same way.
The main area of application of recommender systems is personalization. We want to show content that is relevant at least for a certain segment, and ideally - pick it up for each person. In this regard, the presentation of the content does not differ from sales. Recall the leaders in sales of targeted products: online stores like Amazon and online cinemas can recommend their products. And if we consider content as a product, it turns out that we already know how to recommend and target it well.

How do we do it? There are two basic principles. The first is recommendation systems, which in fact compare people to each other on the basis of their purchases, in this case, on the content that they consumed earlier. Take a simple example: Igor and Peter watched about the same films, and if one of the films was watched only by Igor, then it is logical to recommend it to Peter.
Another principle is much stronger in terms of recommending content - an assessment of its popularity, PageRank. The first such example is search, issue in Yandex, Google. How to determine that a certain page is meaningful? We count the number of links or references to this page on other resources and get a kind of rating that is assigned to it. But it's one thing when five unknown pages link to a publication, and quite another if the links are given by popular brands or major news agencies. It turns out that it is necessary to take into account the rating of those who link to our page - it turns out such a hierarchy.
Tinder works in the same way: when you scroll left-right, the rating is calculated both for you and for the people you are shown. You are shown a photo of those who have about the same rating with you - this is the advisory meaning of the service.

This is a very effective method of automated assessment of the significance of certain information. If you know how to count not only mentions, but also their significance, you can automatically sort all informational presentations for specific target audiences. Therefore, recommendations are used mainly for such level targeting.
The concept of neural networks is simple and boring. Until about the 60s of the last century, the study of the principles of the human brain painted the following picture: there is a certain set of neurons that receive signals at the input. After that, each neuron produces a small modification of the signal and transmits it further. To understand how these neurons are combined into groups within the brain, we decided to create a computer model - a set of neurons that are somehow connected to each other. Thus, the first neural networks originated, and in this form they are still used to solve machine learning problems. But if we are talking about something more advanced, then such a system is not suitable.

Somewhere in the 90s of the last century, scientists realized that the human brain does not work at all. Neurons do interact with each other, but everything is built hierarchically. For example, when I see a picture, information is collected from each of its areas, which is further aggregated into another, smaller group of neurons. And there it is stored as some kind of internal representation. In fact, we think of these inner ideas, and not the real pictures that we see. The theory was immediately recreated in neural networks, and now, by the classification of images, such neural networks work much better than humans. These neural networks are called convolutional - because the process of generalization occurs.

The second breakthrough came when they found out: a person perceives information not at the moment, but taking into account a certain context. To train computers to analyze the accumulated experience, they built the so-called recurrent neural networks. They use the work of previous neural networks, first to classify, and then to create some content. This is all used now in Sequence Modeling, and if it is simpler, in chat bots. For example, when Yandex selects similar words, these are recurrent neural networks that replicate how a person processes information.
The first application of neural networks is content generation. If we have a kind of information server, then a trained neural network allows us to determine the subject matter and write a quite intelligible text. Already there are companies that produce the appropriate software. There are publications that use it for routine infoprovodov - stock reports, financial performance of companies. For factual information — an earthquake passed here, a ship sailed there, and so on — it works fine. But if we are talking about more advanced infopowers, then we will have to seriously work to transform the content generated by the neural network into something really meaningful and adequate.

The second area is classification, it has already been mentioned above. The third is an assessment of perception or A / B testing, which is rarely used somewhere outside sales. In journalism, the principle is similar: we have several forms of publication, and we want to test how it will go down in different target groups. Using this kind of methods, this process can be fully automated.
The last direction will appeal to those who need to write the same content for different channels, resources, target audiences. To publish an article on Habré that has already been published in another edition, you can’t just copy-past. To adapt it, you can either attract a copywriter or use a neural network. For a computer, this is even simpler than machine translation: the text does not need to be converted to another language, syntax, and so on. But in general, all the same.
Where is it used? A pioneer among major agencies is the Associated Press. They use automatic content generation for financial news, in which there is little analytics, but a lot of numbers and factual data. There are three vendors who make such software: Narrative Science, Automated Insights and Article Forge. If you go to their sites, you can see a lot of real-life cases - examples of publications written by robots. All these articles are based on some actual data.

Is there a difference between copyright and generated content? Studies were conducted in the United States and in Germany, during which a large number of articles were shown to groups of journalists - in English and German, respectively. Half of the texts were written by people, half by machines. On average, people could not distinguish between them. And when the subjects were asked to classify texts according to authenticity and interestingness, it turned out that they find more authentic texts written by the machine. When interviewed, they noted that reading them is not as interesting as “human” articles.
It turns out that people are better engaged in entertainment content. And if you need to present some news - use the machine, they will believe it more.
Robots allow you to focus on the content that you want to attach to the content, and not on the tedious process of adapting it to different formats. Another advantage of the machines is the speed of reaction: if you need to quickly process the info pipelines, then this is your tool. We have already said about user personalization, this is a definite plus. The fourth advantage is crowdsourcing: if you use a large number of sources, the machine will be able to automatically classify the information obtained from them, distinguish the good from the bad, choose the adequate one.

There are also potential dangers. The first is an echo camera. The content that is shown to me is personalized based on the similarity of my interests - taking into account what I have already read and the interests of people like me. Thus, after a certain number of iterations, I begin to boil in my own closed information field.
The second danger is information bubbles. If you create some kind of fictional situation, an event, the machine can write under it a lot of different publication options that look authentic. With the help of bots, social networks and so on, such misinformation can be spread to huge audiences.

Now they are talking about the so-called adversial attacks on the neural network. An example is given with the KFC logo: if you show such a picture to a self-driving car, it will immediately rise - artificial intelligence recognizes the image as a stop sign. If such manipulations are possible with texts, then a meaningless set of words corresponding to a certain algorithm will be able to get a high rating of neural networks, and the reader will see some kind of abracadabra.

Fortunately, in practice it is very difficult to carry out such an attack. Recall that the neural network - like our brain - brings any image into line with the internal representation. Look at the picture: on the left of the face, as we see them, and on the right - how it sees the neural network. Having access to the neural network itself, the pictures can be selected, as in the example with the KFC logo. In fact, the problem is known from cryptography, because it is an analogue of the hash function. The neural network in this case is a hash function: you convert some long text into a small internal representation. If you pick up something that matches - hack. But in order to be able to search, you need to access the algorithm.
Virtually all publications on this topic raise the problem of the need for journalists in the future. The question, it seems to me, is not quite correct: someone will be replaced, someone else will not, but it is clear that all journalism cannot be replaced with machines. A person will give them only some basic, banal, simple publications. The problem is different: since basic publications can be created automatically and made easy, the percentage of generated content in a very short time will be much more than that written by people. As we have already found out, the generated content is perceived better from the point of view of reliability - and this allows you to create the most powerful tool for manipulating consciousness, perception. This is probably the worst and most important thing.

To create content using machine learning, the process of “man-machine” interaction is used - not separately, but together, in pairs. First, the machine searches for informational guides, classifies them, predicts importance, generates content ... This is a case for the case when we have a large flow of all sorts of information and we want to respond to it quickly. If you have time to think, and so on, this is a completely different scenario. The content prepared by the machine goes to a journalist or editor who looks, evaluates, appends. Then the text can go to the publication or again to the robot in order to form different versions of the publication for different target audiences. After that, the car is engaged in personalization, chooses for each person what to show him. Of course, this is not always implemented all together
Man from the process of preparing content is not excluded. Robots - nothing more than additional tools that speed up and simplify the process, remove our routine tasks from us.
Reports from “ Content ” in video format can be ordered here . For Habr users, discount on promo code habr_online_promo.
We thank the sponsors:
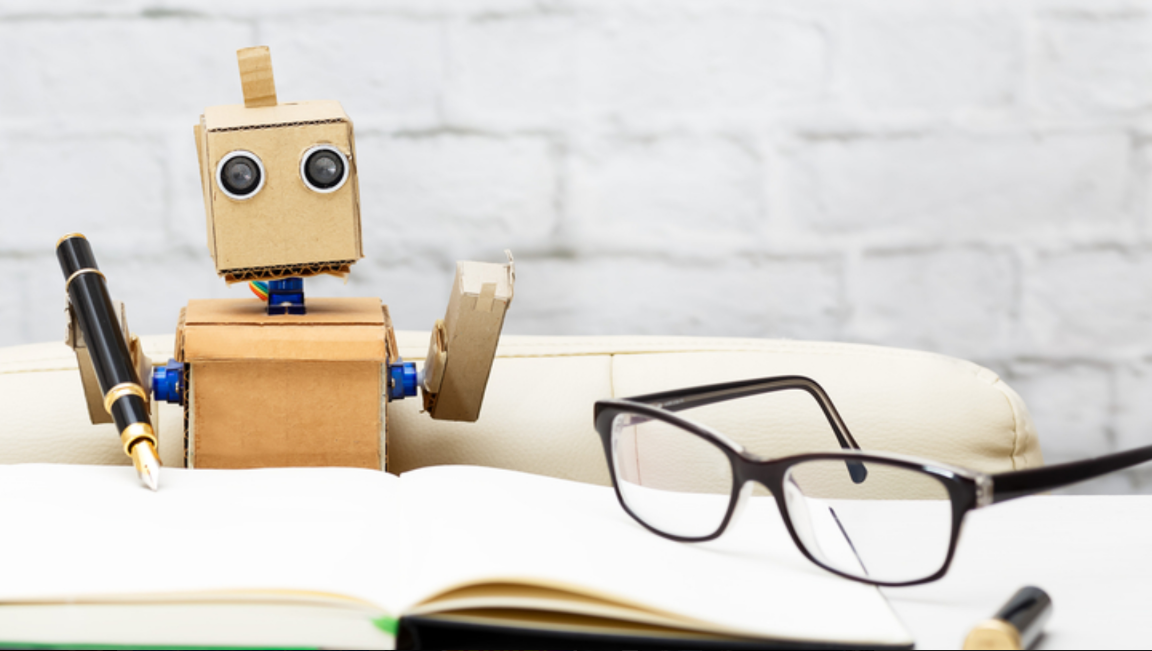
Under the cut the decoding of his report.
About Speaker
Sergey Marin is an expert in artificial intelligence, the director and founder of Data Studio .
Three whales of artificial intelligence
If we are talking about artificial intelligence - in journalism or in any other field - you need, first of all, to understand its structure. The AI consists of three main components: machine learning, recommendation systems and neural networks. By the way, many people consider neural networks to be synonymous with artificial intelligence, but this is just one of the tools, not even the most popular: in each case, those algorithms that work most optimally are used.

Machine Learning: Sort By
Machine learning is used to search for hidden patterns in the data. Imagine that we have a set of infoprovodov or publications that need to be classified, that is, automatically assign them some tags. Or simply texts with a large number of words that need to be divided into certain classes, interests, moods, and so on. How do we do it? If we talk about machine learning, we are not looking for any keywords to draw conclusions based on them. Instead, we show the machine as much as possible of the texts we have already marked with a large number of classes. Then we give the new text, and the machine itself classifies it into the area to which it belongs. That is, we first teach, show many examples.

That is the main use of machine learning in journalism is classification. For example, we have a large number of information leads - from the Internet, social networks, news agencies - and we need to quickly classify them. We have trained our model in advance, and when we have a new information center, the machine understands where it belongs, what its subject matter, what mood it conveys, for which audience it can be applied. The popularity, the rating of some infopowder is predicted in the same way.
Recommender systems: find a personal approach
The main area of application of recommender systems is personalization. We want to show content that is relevant at least for a certain segment, and ideally - pick it up for each person. In this regard, the presentation of the content does not differ from sales. Recall the leaders in sales of targeted products: online stores like Amazon and online cinemas can recommend their products. And if we consider content as a product, it turns out that we already know how to recommend and target it well.

How do we do it? There are two basic principles. The first is recommendation systems, which in fact compare people to each other on the basis of their purchases, in this case, on the content that they consumed earlier. Take a simple example: Igor and Peter watched about the same films, and if one of the films was watched only by Igor, then it is logical to recommend it to Peter.
Another principle is much stronger in terms of recommending content - an assessment of its popularity, PageRank. The first such example is search, issue in Yandex, Google. How to determine that a certain page is meaningful? We count the number of links or references to this page on other resources and get a kind of rating that is assigned to it. But it's one thing when five unknown pages link to a publication, and quite another if the links are given by popular brands or major news agencies. It turns out that it is necessary to take into account the rating of those who link to our page - it turns out such a hierarchy.
Tinder works in the same way: when you scroll left-right, the rating is calculated both for you and for the people you are shown. You are shown a photo of those who have about the same rating with you - this is the advisory meaning of the service.

This is a very effective method of automated assessment of the significance of certain information. If you know how to count not only mentions, but also their significance, you can automatically sort all informational presentations for specific target audiences. Therefore, recommendations are used mainly for such level targeting.
Neural networks: imitation of the brain
The concept of neural networks is simple and boring. Until about the 60s of the last century, the study of the principles of the human brain painted the following picture: there is a certain set of neurons that receive signals at the input. After that, each neuron produces a small modification of the signal and transmits it further. To understand how these neurons are combined into groups within the brain, we decided to create a computer model - a set of neurons that are somehow connected to each other. Thus, the first neural networks originated, and in this form they are still used to solve machine learning problems. But if we are talking about something more advanced, then such a system is not suitable.

Somewhere in the 90s of the last century, scientists realized that the human brain does not work at all. Neurons do interact with each other, but everything is built hierarchically. For example, when I see a picture, information is collected from each of its areas, which is further aggregated into another, smaller group of neurons. And there it is stored as some kind of internal representation. In fact, we think of these inner ideas, and not the real pictures that we see. The theory was immediately recreated in neural networks, and now, by the classification of images, such neural networks work much better than humans. These neural networks are called convolutional - because the process of generalization occurs.

The second breakthrough came when they found out: a person perceives information not at the moment, but taking into account a certain context. To train computers to analyze the accumulated experience, they built the so-called recurrent neural networks. They use the work of previous neural networks, first to classify, and then to create some content. This is all used now in Sequence Modeling, and if it is simpler, in chat bots. For example, when Yandex selects similar words, these are recurrent neural networks that replicate how a person processes information.
How neural networks are used in journalism
The first application of neural networks is content generation. If we have a kind of information server, then a trained neural network allows us to determine the subject matter and write a quite intelligible text. Already there are companies that produce the appropriate software. There are publications that use it for routine infoprovodov - stock reports, financial performance of companies. For factual information — an earthquake passed here, a ship sailed there, and so on — it works fine. But if we are talking about more advanced infopowers, then we will have to seriously work to transform the content generated by the neural network into something really meaningful and adequate.

The second area is classification, it has already been mentioned above. The third is an assessment of perception or A / B testing, which is rarely used somewhere outside sales. In journalism, the principle is similar: we have several forms of publication, and we want to test how it will go down in different target groups. Using this kind of methods, this process can be fully automated.
The last direction will appeal to those who need to write the same content for different channels, resources, target audiences. To publish an article on Habré that has already been published in another edition, you can’t just copy-past. To adapt it, you can either attract a copywriter or use a neural network. For a computer, this is even simpler than machine translation: the text does not need to be converted to another language, syntax, and so on. But in general, all the same.
Where is it used? A pioneer among major agencies is the Associated Press. They use automatic content generation for financial news, in which there is little analytics, but a lot of numbers and factual data. There are three vendors who make such software: Narrative Science, Automated Insights and Article Forge. If you go to their sites, you can see a lot of real-life cases - examples of publications written by robots. All these articles are based on some actual data.

Is there a difference between copyright and generated content? Studies were conducted in the United States and in Germany, during which a large number of articles were shown to groups of journalists - in English and German, respectively. Half of the texts were written by people, half by machines. On average, people could not distinguish between them. And when the subjects were asked to classify texts according to authenticity and interestingness, it turned out that they find more authentic texts written by the machine. When interviewed, they noted that reading them is not as interesting as “human” articles.
It turns out that people are better engaged in entertainment content. And if you need to present some news - use the machine, they will believe it more.
Benefits and hazards
Robots allow you to focus on the content that you want to attach to the content, and not on the tedious process of adapting it to different formats. Another advantage of the machines is the speed of reaction: if you need to quickly process the info pipelines, then this is your tool. We have already said about user personalization, this is a definite plus. The fourth advantage is crowdsourcing: if you use a large number of sources, the machine will be able to automatically classify the information obtained from them, distinguish the good from the bad, choose the adequate one.

There are also potential dangers. The first is an echo camera. The content that is shown to me is personalized based on the similarity of my interests - taking into account what I have already read and the interests of people like me. Thus, after a certain number of iterations, I begin to boil in my own closed information field.
The second danger is information bubbles. If you create some kind of fictional situation, an event, the machine can write under it a lot of different publication options that look authentic. With the help of bots, social networks and so on, such misinformation can be spread to huge audiences.

Now they are talking about the so-called adversial attacks on the neural network. An example is given with the KFC logo: if you show such a picture to a self-driving car, it will immediately rise - artificial intelligence recognizes the image as a stop sign. If such manipulations are possible with texts, then a meaningless set of words corresponding to a certain algorithm will be able to get a high rating of neural networks, and the reader will see some kind of abracadabra.

Fortunately, in practice it is very difficult to carry out such an attack. Recall that the neural network - like our brain - brings any image into line with the internal representation. Look at the picture: on the left of the face, as we see them, and on the right - how it sees the neural network. Having access to the neural network itself, the pictures can be selected, as in the example with the KFC logo. In fact, the problem is known from cryptography, because it is an analogue of the hash function. The neural network in this case is a hash function: you convert some long text into a small internal representation. If you pick up something that matches - hack. But in order to be able to search, you need to access the algorithm.
Not a competitor, but an assistant
Virtually all publications on this topic raise the problem of the need for journalists in the future. The question, it seems to me, is not quite correct: someone will be replaced, someone else will not, but it is clear that all journalism cannot be replaced with machines. A person will give them only some basic, banal, simple publications. The problem is different: since basic publications can be created automatically and made easy, the percentage of generated content in a very short time will be much more than that written by people. As we have already found out, the generated content is perceived better from the point of view of reliability - and this allows you to create the most powerful tool for manipulating consciousness, perception. This is probably the worst and most important thing.

To create content using machine learning, the process of “man-machine” interaction is used - not separately, but together, in pairs. First, the machine searches for informational guides, classifies them, predicts importance, generates content ... This is a case for the case when we have a large flow of all sorts of information and we want to respond to it quickly. If you have time to think, and so on, this is a completely different scenario. The content prepared by the machine goes to a journalist or editor who looks, evaluates, appends. Then the text can go to the publication or again to the robot in order to form different versions of the publication for different target audiences. After that, the car is engaged in personalization, chooses for each person what to show him. Of course, this is not always implemented all together
Man from the process of preparing content is not excluded. Robots - nothing more than additional tools that speed up and simplify the process, remove our routine tasks from us.
Reports from “ Content ” in video format can be ordered here . For Habr users, discount on promo code habr_online_promo.
We thank the sponsors:
- mega- sponsor RuVDS
- water partner "Borjomi"
- of the Letters Metter partner
- conference of Oleg Bunin
- video partner FU2RE
- information partners Adindex, Pressfeed and Media2
Friends, for another 10 days we accept applications for technical tests on the subject of “State and IT” and invite all technical authors to participate. You can declare a story about technology, development, refinement of services, device of various systems and applications, interviews with an expert, a collection of life hacks, a review and other materials on the topic - the main thing is that they should be published on Habré. Detailed information on the contest page .