How does Google's Night Sight system work on phones and why does it work so well
- Transfer
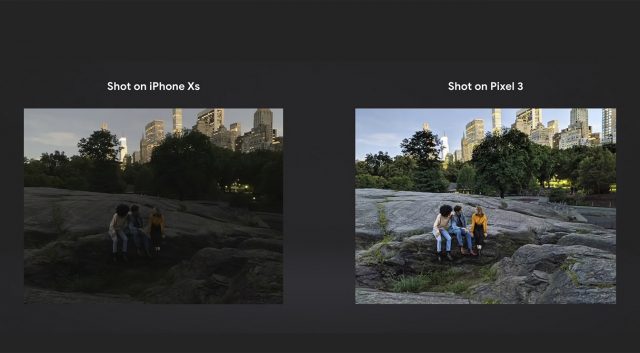
You can be forgiven for the fact that after reading all the accolades for the new night photography feature from Google, Night Sight, you decide that the company has just invented a color film. Night shooting modes did not appear yesterday, and many of the technologies on which they are based have been around for many years. But Google has done an amazing job of combining computational photography skills with unprecedented machine learning abilities to push the boundaries of possibilities beyond everything we have seen before. Let's look at the history of the technology of obtaining photos in low light using several shots taken in a row, think about how it is used in Google, and suppose what contribution AI makes to it.
The difficulty of photographing in low light

All cameras hardly cope with obtaining photos in low light. Without enough photons per pixel in the image it is easy to dominate noise. If you leave the shutter open for a longer time to collect more light and get a useful picture, it will also increase the amount of noise. Worse, it is rather difficult to get a clear picture without a stable tripod. Increasing the gain (ISO) will make the picture brighter, but it will also increase the amount of noise in it.
The traditional strategy was to use larger pixels in larger sensors. Unfortunately, in the cell phones of the phones, the sensors, and, therefore, the pixels, are tiny - they work well with good lighting, but quickly fail when the light level decreases.
As a result, the developers of telephone cameras are left with two options for improving the images obtained in low light. The first is to use multiple images to combine them into one, with noise reduction. An early implementation of such a strategy in a mobile device was the SRAW mode in the DxO ONE add-on for iPhone. He merged four RAW images into one enhanced one. The second option is to use ingenious post-processing (their latest versions are often equipped with machine learning) to reduce the noise level and improve the picture. Google Night Sight uses both approaches.
Multiple images in one shot
To date, we have become accustomed to how telephones and cameras combine several images into one, mainly to improve the dynamic range. Whether it's a group of images with bracketing , as most companies do, or Google's HDR +, which uses several images with a brief exposure, you can get a great picture as a result - if the artifacts generated by merging several images of moving objects can be minimized. Usually, the base frame that best describes the scene on which the useful parts of the other frames are superimposed is selected for this. Huawei, Google and others have also used this approach to create enhanced telephoto photos.. We recently saw the importance of choosing the right base frame when Apple explained that their mess with BeautyGate was due to a bug in which the wrong base frame was selected during photo processing.
It's clear why Google decided to combine these methods of using multiple images to get better photos in low light. At the same time, the company introduces several ingenious innovations in the field of image processing. Most likely, the roots of this lie in the application for Android from Mark Livoy, SeeInTheDark, and his work in 2015 "Obtaining extreme images using cell phones." Livoy was a pioneer in computational photography from Stanford, and now he is an honorary engineer, working on camera technology at Google. SeeInTheDark (a continuation of its earlier work, SynthCam applications for iOS) used a standard phone to accumulate frames, change each frame so that it matches the typed image, and then apply various noise reduction techniques and image enhancement to produce a surprisingly high-quality image with low lighting. In 2017, a programmer from Google, Florian Kants, used some of these concepts to showAs a phone, you can use it to produce professional-quality images even in very low light.
Наложение нескольких изображений, полученных при низком освещении, это хорошо известная технология
Photographers imposed several images to improve the quality of images taken in low light, from the very appearance of digital photography (I suspect that someone did this with film). As for me, I started by doing this manually, and then I used the clever software tool Image Stacker. since the very first digital SLRs were useless at high ISOs, the only way to get a normal night shot was to take a few frames and superimpose them. Some classic photographs, like stellar tracks, were originally obtained that way. Today, this is not so often used when using DSLRs and mirrorless cameras, since modern models have excellent tools for maintaining high ISO and reducing noise from long exposures.
Therefore, it is reasonable that phone manufacturers should do the same. However, unlike patient photographers who shoot stellar tracks with a tripod, the average phone user needs immediate satisfaction, and he will almost never use a tripod. Therefore, the phone has additional difficulties associated with the need to quickly obtain images in low light and minimize blur from camera shake - and ideally, from the movement of the object being shot. Even the optical stabilization available on many top models has its limitations.
I'm not sure which phone maker first applied using multiple shots to get low-light images, but I was the first to use Huawei Mate 10 Pro. In the Night Shot mode, he takes several frames in 4-5 seconds, and then merges them into one photo. Since Huawei real-time preview remains enabled, you can see how it uses several different exposure options, in essence, creating a few frames of bracketing.
In the work describing the first HDR +, Livoy claims that frames with different exposures are harder to align (why HDR + uses several frames with the same exposure), therefore, most likely, Google Night Sight, like SeeInTheDark, also uses several frames with the same exposure. . However, Google (at least in the pre-release version of the application) does not display a real-time image on the screen, so I can only guess. Samsung in the Galaxy S9 and S9 + used a different tactic with the main lens of the double aperture. It can switch to an impressive f / 1.5 in low light to improve picture quality.
Comparison of the capabilities of Huawei and Google in low light
I don't have Pixel 3 or Mate 20 yet, but I have access to Mate 10 Pro with Night Shot and Pixel 2 with the pre-release version of Night Sight. So I decided to compare them myself. In the tests, Google apparently surpassed Huawei, showing less noise and a clearer picture. Here is one of the test sequences:

Huawei Mate 10 Pro in the afternoon

Google Pixel 2 in the afternoon

This is what you can get by photographing the same scene in almost complete darkness without Night Shot mode on Mate 10 Pro. The shutter is open for 6 seconds, so you can see the blur
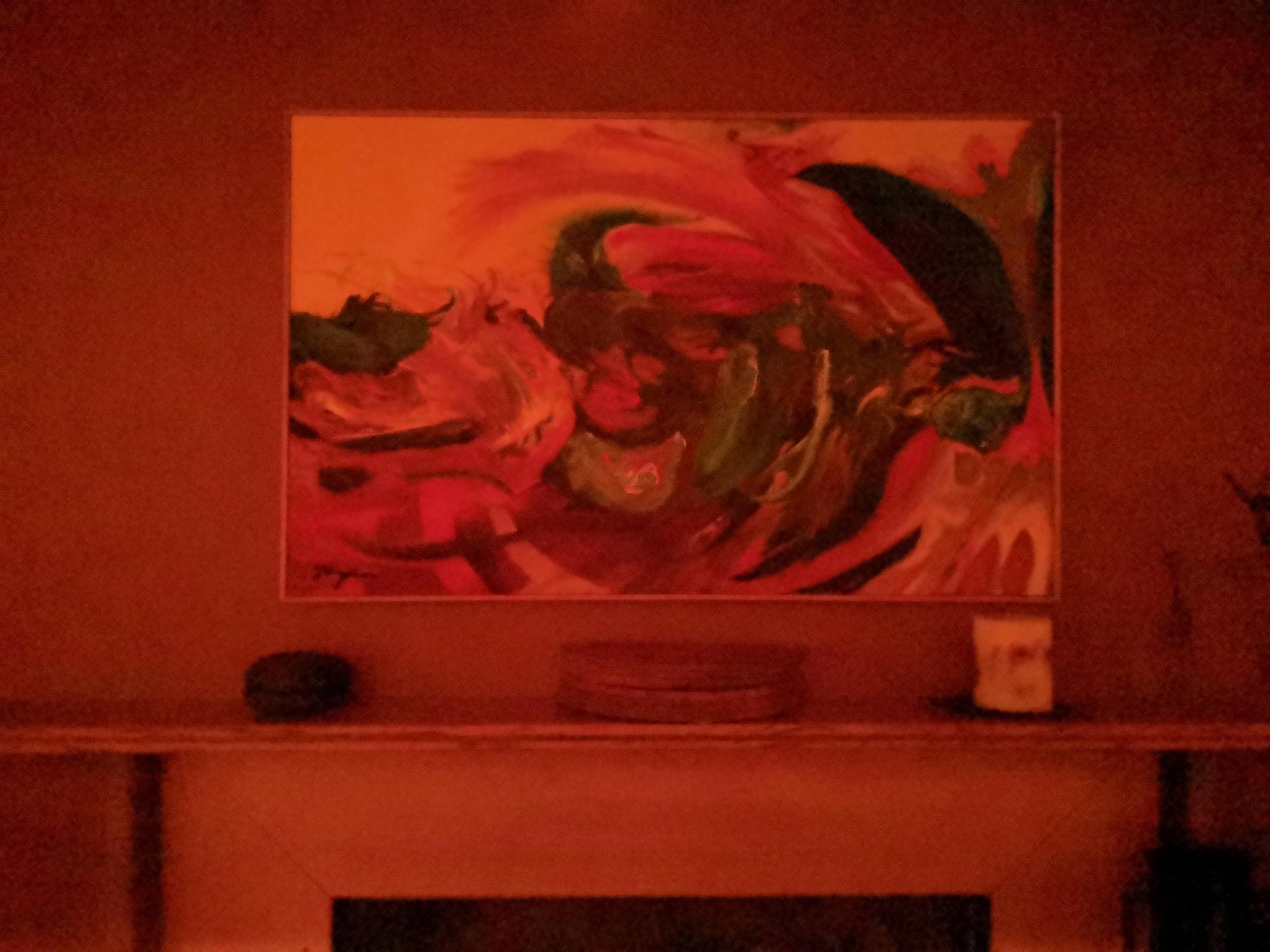
The picture is almost in complete darkness using Night Shot on Huawei Mate 10 Pro. EXIF data shows ISO3200 and 3 seconds of exposure in total.
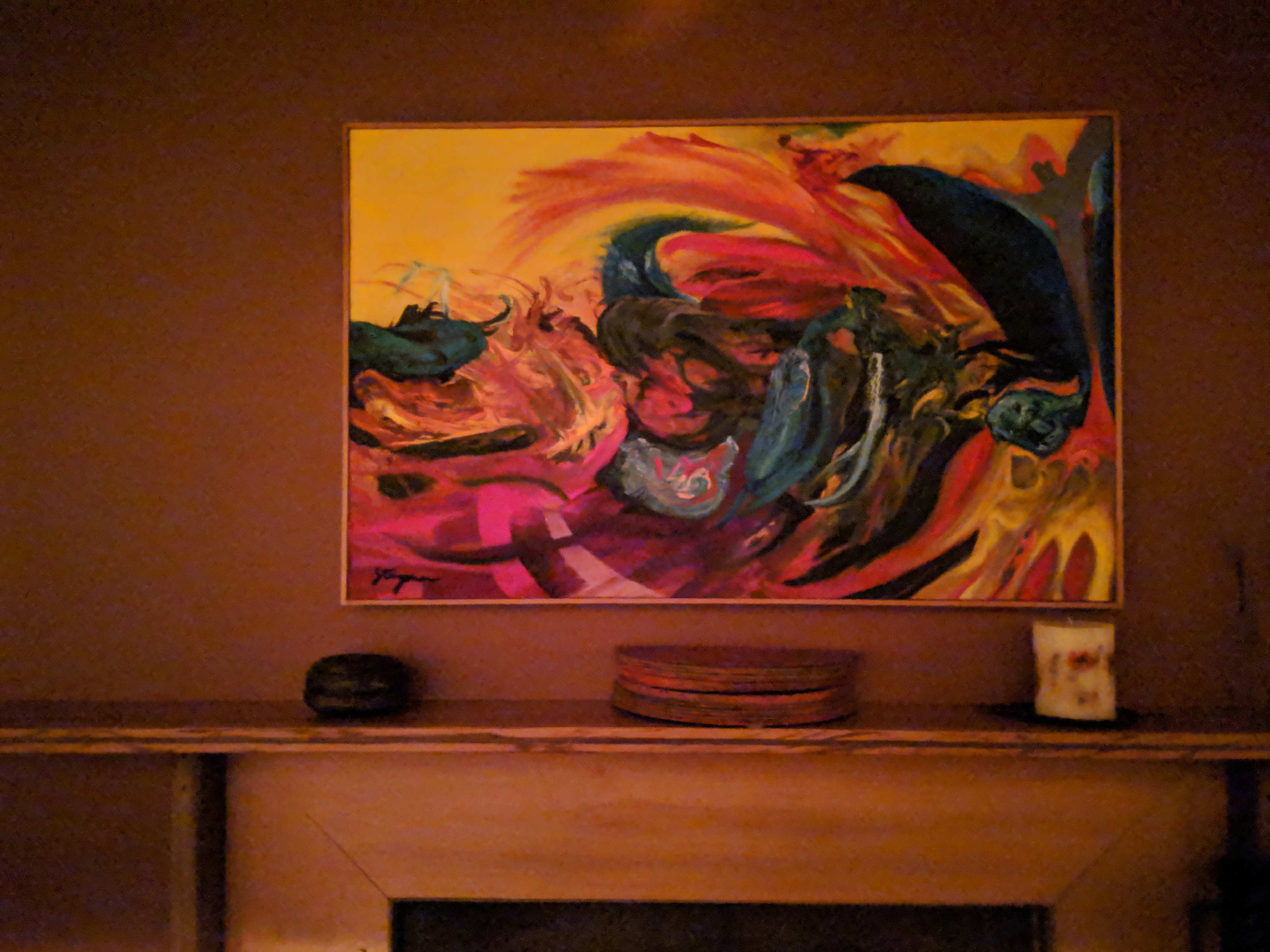
The same scene with the help of the pre-release version of Night Sight on Pixel 2. More precisely, the colors are clearer. EXIF shows ISO5962 and 1/4 s exposure (probably for each of several frames).
Is machine learning the secret component of Night Sight?
Considering how long the overlapping of several images has existed, and how many versions of this technology have been used by camera and phone manufacturers, it becomes interesting why Google's Night Sight seems so much better than the rest. Firstly, even the technology in the original work of Livoya is very difficult, so those years that Google had to improve it should have given the company a good edge. But the company also announced that Night Sight uses machine learning to select the right colors in the image based on what was in the frame.
It sounds cool, but foggy. It is not clear whether the technology highlights individual objects, knowing that their color should be monotonous, or color known objects accordingly, or recognize the general type of scene, as intelligent automatic exposure algorithms do, and decide how a similar scene should look like (green foliage, white snow, blue sky). I am sure that after the release of the final version, the photographers will get more experience with this opportunity, and we will be able to learn the details of how the technology uses the MO.
Another place where MO can be useful is the initial calculation of the exposure. HDR +’s underlying Night Sight technology, as described by Google’s SIGGRAPH, relies on a hand-marked set of thousands of photos to help it choose the right exposure. In this area, MOs can bring some improvements, especially by performing exposure calculations in low light conditions, where scene objects are noisy and difficult to distinguish. Also, Google experimented with using neural networks to improve the quality of photos on phones, so it would not be a surprise when some of these technologies begin to be implemented.
Whatever combination of these technologies is used in Google, the result is definitely the best of all cameras capable of shooting in low light today. I wonder if the Huawei P20 family will be able to give something to bring the quality of their Night Shot technology to what they did at Google.