
How does a relational database work?
- Transfer
- Tutorial

In fact, few people really understand how relational databases work. And many developers really don’t like it when they don’t understand something. If relational databases use about 40 years, then there is a reason. RDB is a very interesting thing, because it is based on useful and widely used concepts. If you would like to understand how RDBs work, then this article is for you.
It must be emphasized right away: from this material you will NOT learn how to use the database. However, to master the material, you must be able to write at least a simple connection request and a CRUD request. This is enough to understand the article, the rest will be explained.
The article consists of three parts:
- Overview of low-level and high-level database components.
- Overview of the query optimization process.
- Overview of transaction management and buffer pool.
1. The basics
Once upon a time (in a distant, distant galaxy), developers had to keep in mind the exact number of operations that they code. They wholeheartedly felt the algorithms and data structures because they could not afford to waste processor and memory resources on their slow computers of the past. In this chapter, we recall some concepts that are necessary for understanding the operation of the database.
1.1. O (1) vs O (n 2 )
Today, many developers do not really think about the time complexity of algorithms ... and they are right! But when you have to work with a large amount of data, or if you need to save milliseconds, then the time complexity becomes extremely important.
1.1.1. Concept
Time complexity is used to evaluate the performance of an algorithm, how long the algorithm will run for input data of a certain size. The time complexity is designated as “O” (read as “O big”). This designation is used in conjunction with a function that describes the number of operations performed by an algorithm to process input data.
For example, if you say “this algorithm is O big of some function”, then this means that for a certain amount of input data, the algorithm needs to perform a number of operations proportional to the value of the function of this particular amount of data.
What matters here is not the amount of data, but the dynamics of increasing the number of operations with increasing volume. The time complexity does not allow us to calculate the exact quantity, but it is a good way to estimate it.

In this graph, you can see how different difficulty classes change. For clarity, a logarithmic scale is used. In other words, the amount of data is rapidly increasing from 1 to 1,000,000. We see that:
- O (1) is “constant time”, that is, its value is constant.
- O (log (n)) - logarithmic time, increases very slowly.
- Worst of all, O (n 2 ) is the quadratic time.
- The other two difficulty classes are increasing rapidly.
1.1.2. Examples
With a small amount of input, the difference between O (1) and O (n 2 ) is negligible. Let's say we have an algorithm that needs to process 2000 elements.
- An algorithm with O (1) will require 1 operation.
- O (log (n)) - 7 operations.
- O (n) - 2000 operations.
- O (n * log (n)) - 14,000 operations.
- O (n 2 ) - 4,000,000 operations.
It seems that the difference between O (1) and O (n 2 ) is huge, but in reality you will lose no more than 2 ms. You don’t have time to blink an eye, literally. Modern processors perform hundreds of millions of operations per second , and that is why in many projects, developers neglect to optimize performance.
But now let's take 1,000,000 elements, which is not too much by the standards of the database:
- An algorithm with O (1) will need 1 operation to process.
- O (log (n)) - 14 operations.
- O (n) - 1,000,000 operations.
- O (n * log (n)) - 14 million operations.
- O (n 2 ) - 1,000,000,000,000 operations.
In the case of the last two algorithms, you can have time to drink coffee. And if you add another 0 to the number of elements, then during processing you can take a nap.
1.1.3. Some more details
so that you can navigate:
- Finding an item in a good hash table takes O (1).
- In a well balanced tree - O (log (n)).
- In the array, O (n).
- The best sorting algorithms have O (n * log (n)) complexity.
- Bad sorting algorithms are O (n 2 ).
Below we look at these algorithms and data structures.
There are several classes of time complexity:
- Medium difficulty
- Complexity at best
- Worst case difficulty
The most common third class. By the way, the concept of "complexity" is also applicable to the consumption of memory and disk I / O by the algorithm.
There are difficulties far worse than n 2 :
- n 4 : typical of some bad algorithms.
- 3 n : worse! Below we will consider one algorithm with a similar time complexity.
- n !: generally hellish trash. You are tortured to wait for the result even on small amounts of data.
- n n : if you have waited for the result of the execution of this algorithm, then it’s time to ask yourself a question, but should you even do this?
1.2. Merge Sort
What do you do when you need to sort a collection? Call the sort () function, right? But do you understand how it works?
There are some good sorting algorithms, but here we will look at just one of them: merge sorting. Perhaps this knowledge does not seem useful to you now, but you will change your mind after the chapters about query optimization. Moreover, understanding the operation of this algorithm will help to understand the principle of an important operation - merge join.
1.2.1. Merge
The merge sort algorithm is based on one trick: merging two sorted arrays of size N / 2 each requires only N operations, called merging.
Consider an example of a merge operation:

As you can see, to create a sorted array of 8 elements, for each of them you need to do one iteration in two source arrays. Since they are already pre-sorted, then:
- First, they compare the available elements,
- the smallest of them is transferred to a new array,
- and then it returns to the array where the previous element was taken from.
- Steps 1-3 are repeated until only one element remains in one of the source arrays.
- After that, all the remaining elements are transferred from the second array.
This algorithm works well only because both source arrays were sorted in advance, so it does not need to return to their beginning during the next iteration.
Example merge sort pseudo code:
array mergeSort(array a)
if(length(a)==1)
return a[0];
end if
//recursive calls
[left_array right_array] := split_into_2_equally_sized_arrays(a);
array new_left_array := mergeSort(left_array);
array new_right_array := mergeSort(right_array);
//merging the 2 small ordered arrays into a big one
array result := merge(new_left_array,new_right_array);
return result;
This algorithm breaks down the task into a number of small subtasks (the divide and conquer paradigm). If you do not understand the essence, then try to imagine that the algorithm consists of two phases:
- Division phase - the array is divided into smaller arrays.
- Sorting phase - small arrays get together (merge).
1.2.2. Division phase
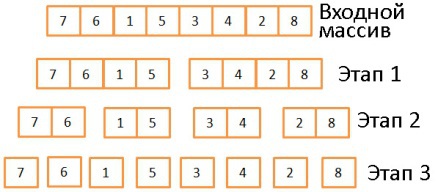
In three stages, the initial array is divided into subarrays consisting of one element. In general, the number of division steps is defined as log (N) (in this case, N = 8, log (N) = 3). The idea is to divide incoming arrays in half at each stage. That is, it is described by the logarithm with base 2.
1.2.3. Sorting phase
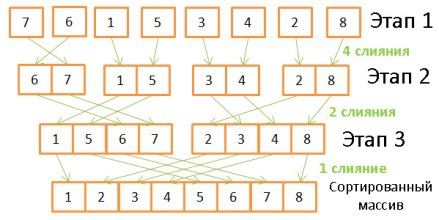
Here the reverse operation takes place, combining arrays with a twofold increase in their size. To do this, you need to do 8 operations at each stage:
- At the first stage, there are 4 mergers, 2 operations each.
- On the second - 2 mergers in 4 operations.
- On the third - 1 merger of 8 operations.
Since the number of steps is defined as log (N), the total number of steps is defined as N * log (N).
1.2.4. Merge Sorting
Features What are the benefits of this algorithm?
- It can be modified to reduce memory consumption if you do not want to create new arrays, but modify the incoming one (the so-called “ substitution algorithm ”).
- It can be modified to reduce memory consumption and use of the disk subsystem, reducing the number of input / output operations. The idea is that only those pieces of data that are processed at a particular point in time are in memory. This is especially important when you need to sort multi-gigabyte tables, and only a megabyte buffer of 100 is available. This algorithm is called “ external sorting ”.
- It can be modified to run in multiple parallel processes / threads / servers
- For example, distributed merge sort is a key feature of Hadoop .
This algorithm can practically turn lead into gold ! It is used in most (if not all) DBMSs, although it is not alone. If you're interested in more dirty details, you can read one research paper that looks at the pros and cons of common sorting algorithms.
1.3. Array, tree and hash table
So, with the time complexity and sorting sorted out. Now let's discuss the three data structures on which modern databases are held.
1.3.1. Array A
two-dimensional array is the simplest data structure. The table can be represented as an array:
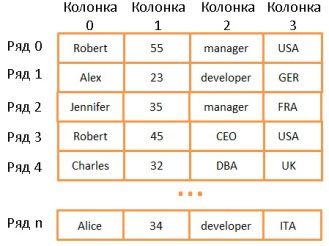
Here, each row represents an object, a column is a property of the object. Moreover, different columns contain different types of data (integers, strings, dates, etc.).
This is a very convenient way to store and visualize information, but very inappropriate for cases when you need to find some specific value. Let's say you need to find all the employees working in the UK. To do this, you have to look through all the lines to find those with the value “UK”. This will require N operations (N is the number of rows). Not so bad, but it would be nice and faster. And here the trees will help us.
Note: most modern DBMSs use advanced types of arrays that make it possible to store tables more efficiently (for example, based on heaps or indexes). But this does not solve the problem of column search speed.
1.3.2. Database tree and index
A binary search tree is a tree in which the key in each node should be:
- More than any of the keys in the branch on the left.
- Less than any of the keys in the branch on the right.
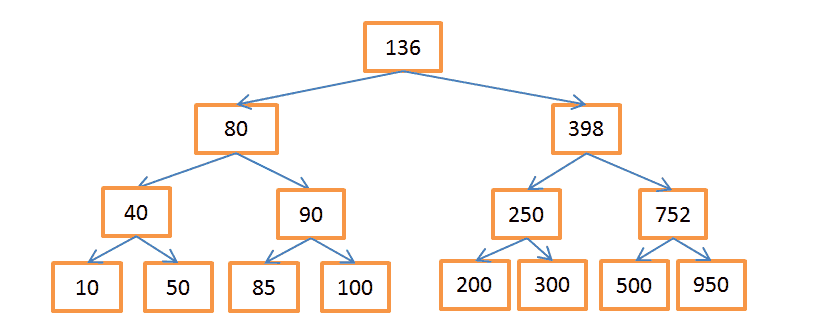
Each node represents a pointer to a row in a related table.
The above tree contains N = 15 elements. Let's say we need to find a node with a value of 208:
- Let's start with the node containing the key 136. Since 136 <208, then follow the right branch of the tree.
- 398> 208, then we go down the left branch coming from this node.
- 250> 208, again go along the left branch.
- 200 <208, you need to go on the right branch. But since the node with key 200 does not have branches, the desired value does not exist. If it existed, then node-200 would have branches.
Suppose now you need to find a node with a value of 40:
- Again, start with the root node-136. Once 136> 40, then we go along the left branch.
- 80> 40, again "turn left."
- 40 = 40, such a node exists. We extract the row ID stored in this node (this is not the key value), and look for it in the related table.
- Knowing the row ID, you can very quickly retrieve the data you need.
Each search procedure consists of a certain number of operations as you travel through the branches of a tree. The number of operations is defined as Log (N), as is the case with merge sort.
But back to our problem.
Suppose we have string values corresponding to the names of different states. Accordingly, the nodes of the tree contain string keys. To find employees working in “UK”, you need to look at all the nodes to find the appropriate one, and extract from it the ID of all rows containing this value. Unlike array searches, here we need only log (N) operations. The described construction is called the database index.
Such an index can be created for any group of columns (for string, integer, their combinations, dates, etc.), if you have a function for comparing keys (i.e. groups of columns). This way you can distribute the keys in a specific order.
B + tree index
Despite the high search speed, the tree does not work well in cases where you need to get several elements within two values . The time complexity will be equal to O (N), because we have to look at each node and compare it with the given conditions. Moreover, this procedure requires a large number of input / output operations, because you need to read the entire tree. That is, we need a more efficient way to request a range. In modern databases, a modified version of the tree is used for this - B + tree. Its peculiarity is that only the lowest nodes (“leaves”) store information (row IDs of the linked table), and all other nodes are designed to optimize the search in the tree.

As you can see, there are twice as many nodes. Decision Nodes help you find the nodes you need that contain the row IDs in the table. But the search complexity is still O (log (N)) (one level of nodes has been added). However, the main feature of the B-tree is that the “leaves” form hereditary bonds .
Let's say we need to find values in the range from 40 to 100:
- First, look for everything that is greater than 40 or the value closest to it if 40 does not exist. Just like in a normal tree.
- And then, using the inheritance relations, we select everything that is less than 100.
Let the tree contain N nodes, and we need to select M heirs. Finding a specific node will require log (N) operations, as in a regular tree. But finding him, we spend only M operations on the search for M heirs. That is, in total we need M + log (N) operations, unlike N in the case of a normal tree. We don’t even need to read the whole tree, but only M + log (N) nodes, so the disk system is used less. If the value of M is small (for example, 200), and N, on the contrary, is large, (1,000,000), then the gain in search speed will be very significant.
But there is a fly in the ointment at this celebration of life. If you add or remove rows in the database, and, consequently, in the associated B + tree index, then:
- You must maintain the order in which nodes are placed inside the B-tree, otherwise you cannot find anything.
- It is necessary that the B + tree consist of as few levels as possible, otherwise the time complexity will be not O (log (N)), but O (N).
In other words, the B + tree must be self-governing and self-balancing. Due to the low-level optimization, B + trees must be fully balanced. Fortunately, all of this can be done using smart delete and paste operations. But you have to pay for it: insert and delete operations in the B + tree have complexity O (log (N)). So surely many of you are familiar with this advice: do not get involved in creating a large number of indexes . Otherwise, performance drops when adding / updating / deleting rows, since the database has to update all kinds of indexes, and each of them requires O (log (N)) operations. In addition, indexes increase the load on the transaction manager, we will talk about this below.
Read more about B + trees on Wikipedia., as well as in articles ( one , two ) from leading MySQL developers. They talk about how InnoDB (the MySQL engine) works with indexes.
1.3.3. Hash Table
This data structure is very useful for cases where search speed is especially important. Understanding the operation of the hash table is necessary for the subsequent understanding of such an important operation as a hash join. A hash table is often used to store some internal data (lock table, buffer pool). This structure is used to quickly search for elements by their keys. To build a hash table you need:
- The key for each item.
- A hash function that computes hashes by key. Hashes are also called blocks.
- Key comparison function. Having found the correct block, we can use this function to find the desired element inside the block.
Here is an example of a simple hash table:
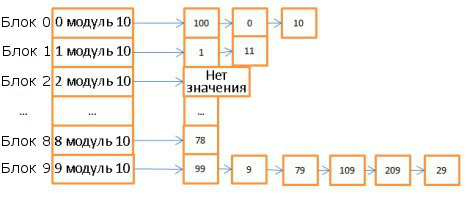
Here are 10 blocks, the module 10 of the key value was taken as a hash function. That is, to find the desired block, it is enough to know the last digit of the key value: if it is 0, then the element is stored in basket 0, if 1, then in basket 1, etc. As a comparison function, the operation of comparing two integers is used.
Suppose we need to find element 78:
- The hash table computes the hash value, it is 8.
- We turn to block 8 and immediately find the desired element.
That is, we spent only two operations: to calculate the hash and to search inside the block.
- Now look for element 59:
- We calculate the hash, it is equal to 9.
- We turn to block 9, the first element is 99. Since 99 is not equal to 59, we compare with the second element (9), then with the third (79), and so on until the last (29).
- The item is missing.
Total we spent 7 operations.
As you can see, the search speed depends on the desired value. Change the hash function, it will calculate hashes modulo 1 000 000 (that is, we take the last 6 digits). In this case, searching for element 59 will take only one operation, since there are no elements in block 000059. Therefore, it is extremely important to choose a successful hash function that will generate blocks with a very small number of elements.
In the above example, it is easy to choose the appropriate option. But this is too simple a case. It is much harder to choose a hash function if the keys are:
- String (e.g. last names).
- Double string (first and last names).
- Double string with date (date of birth added)
etc.
But if you managed to find a good hash function, then the complexity of searching the table will be O (1).
Array vs. Hash Table
Why not use single arrays? The fact is that:
- The hash table can be loaded into memory by half, and the rest can be left on disk.
- In the case of an array, you have to use contiguous memory space. And if you load a large table, then it is very difficult to provide a sufficient amount of this adjacent space.
- You can select any keys for the hash table. For example, the name of the state and the name of the person.
For details, see the Java HashMap article , this is an example of an effective implementation of a hash table. For this you do not need to understand Java, if that.
2. General overview
We examined the basic components of the database. Now let's step back and take a look around the whole picture.
A database is a collection of information that can be easily accessed and modified. But the same can be said of a group of files. In fact, the simplest databases, like SQLite, are just a bunch of files. But here it is important how exactly these files are organized internally and interconnected, since SQLite allows you to:
- Use transactions to ensure the integrity and coherence of data.
- Quickly process data regardless of its size.
In general, the database structure can be represented as follows:
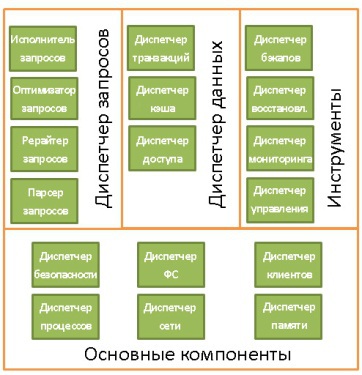
In fact, there are a bunch of different ideas about how the database structure might look. So do not get hung up on this illustration. It is only necessary to understand that the database consists of various components interacting with each other.
The main components of the database (Core Components):
- Process manager . Many databases have a pool of processes / threads that need to be managed. Moreover, in the pursuit of performance, some databases use their own threads, and not provided by the OS.
- Network manager . Network bandwidth is of great importance, especially for distributed databases.
- File system manager . The first "bottleneck" of any database is the performance of the disk subsystem. Therefore, it is very important to have a manager that works perfectly with the OS file system or even replaces it.
- Memory manager . In order not to run into the low performance of the disk subsystem, you need to have a lot of RAM. So, you need to effectively manage it, which this dispatcher does. Especially when you have many concurrent queries that use memory.
- Security Manager . Manages user authentication and authorization.
- Customer dispatcher . Manages client connections.
Tools:
- Backup manager. The purpose is obvious.
- Recovery manager. It is used to restore the coherent state of a database after a failure.
- Monitoring manager. It is engaged in the logging of all activities inside the database and is used to monitor its status.
- General Manager It stores metadata (like names and table structure) and is used to manage databases, schemas, table spaces, etc.
Query Manager
- Parser of requests . Checks their validity.
- Request Writer. Carries out preliminary optimization.
- Query optimizer .
- Query Executor. Compiles and executes.
Data Manager:
- Transaction manager . Engaged in their processing.
- The cache manager . Used to send data before use and before writing to disk.
- Data Access Manager . Controls access to data in the disk subsystem.
Next, we will look at how the database manages SQL queries with:
- Customer dispatcher.
- Query manager.
- Data manager.
3. Customer Manager
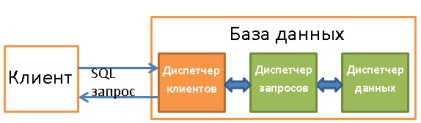
Used to manage connections with clients - web servers or end users / applications. The client manager provides different ways to access the database using both well-known APIs (JDBC, ODBC, OLE-DB, etc.) and using proprietary ones.
When you connect to the database:
- The dispatcher first authenticates you (check your username and password), and then authorizes you to use the database. These permissions are determined by your DBA.
- Next, the dispatcher checks to see if there is an available process (or thread) that can process your request.
- He also checks whether the base is in a state of high load.
- The dispatcher can wait until the necessary resources are released. If the waiting period has ended, but they have not been released, the dispatcher closes the connection with an error message.
- If resources are available, the dispatcher redirects your request to the query dispatcher.
- Since the client manager sends and receives data from the request manager, it saves them in the buffer in parts and sends it to you.
- When a problem occurs, the dispatcher terminates the connection with some explanation and frees up resources.
4. Request Manager
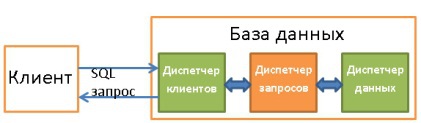
It is he who is the heart of the database. Here, all poorly written requests turn into fast-executing code, then execution takes place, and the result is sent to the client manager. The described process consists of several stages:
- The request is first checked for validity (parsed).
- Then it is overwritten in order to eliminate useless operations and preliminary optimization.
- Next, final optimization is done to increase productivity. After that, the request is transformed into a plan of execution and access to data.
- The plan is compiling.
- And fulfilled.
If you want to know more, you can study the following materials:
- Access Path Selection in a Relational Database Management System . A 1979 article is about optimization.
- A very good and in-depth presentation on how DB2 9.x optimizes queries.
- No less good presentation on how PostgreSQL does the same. Here the information is presented in the most understandable form.
- The official SQL documentation on optimization. It is pretty easy to read because SQLite uses simple rules. In addition, this is the only official document on this topic.
- A good presentation on how SQL Server 2005 optimizes queries.
- White paper on optimization in Oracle 12c.
- Two theoretical courses on query optimization from the author of the book “Database System Concepts”: the first and second . Good material focusing on saving input / output operations. But assimilation requires a good command of CS.
- Another theoretical course , more accessible to understanding. But it is dedicated to union operators and I / O operations.
4.1. Request parser
Each SQL statement is sent to the parser and checked for the correct syntax. If you make a mistake in the request, the parser will reject it. For example, write SLECT instead of SELECT.
But parser functions are not limited to this. It also checks that keywords are used in the correct order. For example, if WHERE goes before SELECT, the request will be rejected.
The parser also analyzes the tables and their fields inside the query. It uses database metadata to verify:
- The very fact of the existence of the table.
- The presence of fields in it.
- Possibilities of operations with fields of available types. For example, you cannot compare integers with strings, or use the substring () function with respect to integers).
Next, the parser checks if you have the right to read (or write) tables in the query. These permissions are also set by your DBA.
During all these checks, the SQL query is transformed into an internal representation (most often into a tree). If everything is in order, then it is sent to the request rewriter.
4.2. Request Writer
His tasks include:
- Preliminary query optimization.
- Exclusion of unnecessary operations.
- Help the optimizer find the best possible solution.
The rewriter applies a list of known rules to the request. If the request satisfies all of them, then it is overwritten. Here are some of these rules:
- Merge of representations (types) . If you use a view in a query, then it is transformed using SQL code.
- Rollup subqueries. The presence of subqueries makes optimization very difficult, so the rewriter tries to modify the request in such a way as to get rid of the subqueries.
For example, this query:
SELECT PERSON.*
FROM PERSON
WHERE PERSON.person_key IN
(SELECT MAILS.person_key
FROM MAILS
WHERE MAILS.mail LIKE 'christophe%');
will be replaced by:
SELECT PERSON.*
FROM PERSON, MAILS
WHERE PERSON.person_key = MAILS.person_key
and MAILS.mail LIKE 'christophe%';
- Exclusion of unnecessary operations. Suppose you use DISTINCT even though you have a UNIQUE clause that prevents non-unique data from appearing. In this case, the DISTINCT keyword is deleted by the parser.
- Prevention of unnecessary associations. If you specified the same join condition twice, since one of them was hidden in the view, or simply meaningless due to transitivity, then it is deleted.
- Arithmetic calculations. If you write something that requires computation, then it is done once during the rewriting. Let's say WHERE AGE> 10 + 2 transforms into WHERE AGE> 12. And TODATE (some date) is replaced with a date in the corresponding format.
- Partition pruning . If you use partitioned tables, the rewriter may find suitable partitions.
- Rewriting a materialized view. If you have a materialized view that matches a subset of the predicates in the query, then the rewriter checks to see if the representation is relevant and modifies the query so that it uses the materialized representation instead of tables.
- Special rules. If you have specific rules for modifying the request (like Oracle policies), then the rewriter can apply them.
- OLAP transforms. The functions of analysis / cropping, star-shaped associations, coagulation, etc. are transformed. Since the rewriter and the optimizer interact very closely, most likely, the optimizer can perform OLAP transformations, at the discretion of the database.
After applying the policies, the request is sent to the optimizer, and there the fun begins.
4.3. Statistics
Before moving on to query optimization, let's talk about statistics, because without it, the database becomes completely brainless. If you do not tell her to analyze her own data, she will not do this, making very bad assumptions.
What kind of information does a database need?
First, let's recall how the database and OS store data. To do this, they use such minimally possible entities as pages or blocks (4 or 8 Kbytes in size by default). If you need to save 1 Kbyte, then this will take a whole page anyway. That is, you waste a lot of space in memory and on disk.
Let's get back to our statistics. When you ask the database to collect statistics, it:
- Counts the number of rows / pages in a table.
- For each column:
- Counts the number of unique values.
- Defines the length of data values (min, max, average).
- Defines a range of data values (min, max, average).
- Collects information about database indices.
All this information helps the optimizer to predict the number of input / output operations, as well as the consumption of processor and memory resources when executing a request.
The statistics for each column are very important. For example, we need to combine two columns in the table: “last name” and “first name”. Thanks to statistics, the database knows that it contains 1,000 unique values “name” and 1,000,000 - “last name”. Therefore, the database will combine the data in this order - “last name, first name” and not “first name, last name”: this requires much less comparison operations, since the probability of coincidence of last names is much lower, and in most cases it is enough to take the first 2-3 letters surnames.
But all this is basic statistics. You can also ask the database to collect additional statistics and build histograms. They give an idea of the distribution of values within columns. For example, determine the most common values, quantiles, etc. These additional statistics help the database even better plan the query. Especially in the case of equality predicates (WHERE AGE = 18) or range predicates (WHERE AGE> 10 AND AGE <40), since the database will better represent the number of columns related to these predicates (selectivity, selectivity).
Statistics are stored as metadata. For example, you can find it in:
- Oracle: USER / ALL / DBA_TABLES and USER / ALL / DBA_TAB_COLUMNS
- DB2: SYSCAT.TABLES and SYSCAT.COLUMNS
Statistics need to be updated regularly. There is nothing worse than a database that thinks it consists of 500 rows, while there are 1,000,000 in it. Unfortunately, it takes some time to collect statistics, so in most databases this operation is not performed automatically by default. When you have millions of records, collecting statistics on them is quite difficult. In this case, you can only collect basic information, or take a small part of the database (for example, 10%) and collect complete statistics on it. But there is a risk that the rest of the data may differ significantly, which means your statistics will be unrepresentative. And this will lead to improper planning of the request and increase the time of its execution.
If you want to know more about statistics, read the documentation for your databases. Best describedfor PostgreSQL .
4.4. Query optimizer
All modern databases use CBO (Cost Based Optimization), cost optimization. Its essence is that for each operation its “cost” is determined, and then the total cost of the request is reduced by using the most “cheap” chains of operations.
For a better understanding of cost optimization, we’ll look at three common ways to combine two tables and see how even a simple join query can turn into a nightmare for the optimizer. In our consideration, we will focus on time complexity, although the optimizer calculates the “cost” in processor resources, memory, and I / O operations. Just time complexity is an approximate concept, and to determine the necessary processor resources, you need to count all operations, including addition, if statements, multiplication, iterations, etc.
Moreover:
- To perform each high-level operation, the processor performs a different number of low-level operations.
- The cost of processor operations (in terms of cycles) is different for different types of processors, that is, it depends on the specific architecture of the CPU.
Therefore, it will be easier for us to evaluate in the form of complexity. But remember that most of the time database performance is limited by the disk subsystem, not processor resources.
4.4.1. Indexes
We talked about them when we looked at B-trees. As you remember, indexes are already sorted. By the way, there are other types of indices, for example, bitmap index. But they do not give a gain in terms of processor, memory and disk subsystem use compared to B-tree indexes. In addition, many modern databases can dynamically create temporary indexes for current queries, if this helps reduce the cost of implementing the plan.
4.4.2. Methods of access
Before applying the union operators, you must first obtain the necessary data. You can do this in the following ways.
- Full scan. The database simply reads the whole table or index. As you understand, for a disk subsystem an index is cheaper to read than a table.
- Range scan. It is used, for example, when you use predicates like WHERE AGE> 20 AND AGE <40. Of course, to scan the range of an index, you need to have an index for the AGE field.
In the first part of the article, we already found out that the time complexity of a range query is defined as M + log (N), where N is the amount of data in the index, and M is the estimated number of rows within the range. The values of both of these variables are known to us thanks to statistics. When scanning a range, only a part of the index is read, so this operation costs less than a full scan. - Scan by unique values. It is used in cases where you need to get only one value from the index.
- Access by row ID. If the database uses the index, then most of the time it will search for rows associated with it. For example, we make this request:
SELECT LASTNAME, FIRSTNAME from PERSON WHERE AGE = 28
If we have an index for the age column, then the optimizer will use the index to search for all 28-year-olds, and then request the ID of the corresponding rows of the table, since the index contains information only about age.
Let's say we have another request:SELECT TYPE_PERSON.CATEGORY from PERSON, TYPE_PERSON WHERE PERSON.AGE = TYPE_PERSON.AGE
To combine with TYPE_PERSON, the index on the PERSON column will be used. But since we did not request information from the PERSON table, no one will access it by row ID.
This approach is only good for a small number of hits, as it is expensive in terms of I / O. If you often need to contact by ID, it is better to use a full scan. - Other ways . You can read about them in the Oracle documentation . Different names may be used in different databases, but the principles are the same everywhere.
4.4.3. Combining operations
So, we know how to get the data, it's time to combine them. But first, let's define new terms: internal dependencies and external dependencies . Dependence may be:
- table,
- index,
- an intermediate result of a previous operation (for example, a previous join).
When we combine two dependencies, the union algorithms control them differently. Suppose A JOIN B is the union of A and B, where A is an external relationship and B is an internal relationship.
Most often, the value of A JOIN B is not equal to the value of B JOIN A.
Suppose that the external dependence contains N elements, and the internal - M. As you remember, the optimizer knows these values thanks to statistics. N and M are cardinal numbers of dependencies .
- Combining using nested loops. This is the easiest way to combine.
It works like this: for each line of the external dependency, matches are found for all the lines of the internal dependency.
Pseudo-code example:nested_loop_join(array outer, array inner) for each row a in outer for each row b in inner if (match_join_condition(a,b)) write_result_in_output(a,b) end if end for end for
Since there is a double iteration, the time complexity is defined as O (N * M).
For each of the N lines of external dependency, you need to consider M lines of external dependence. That is, this algorithm requires N + N * M reads from disk. If the internal dependence is small enough, then you can put it in its entirety in memory, and then the disk subsystem will have only M + N readings. So it is recommended to make internal dependence as compact as possible in order to drive it into memory.
From the point of view of time complexity, there is no difference.
You can also replace the internal dependency with an index, this will save input / output operations.
If the internal dependency does not fit into the memory entirely, you can use another algorithm that uses the disk more economically.- Instead of reading both dependencies line by line, they are read by groups of lines (bunch), while one group of each dependency is stored in memory.
- Lines from these groups are compared with each other, and found matches are stored separately.
- Then new groups are loaded into the memory and also compared with each other.
And so on, until the groups end.
Algorithm Example:// improved version to reduce the disk I/O. nested_loop_join_v2(file outer, file inner) for each bunch ba in outer // ba is now in memory for each bunch bb in inner // bb is now in memory for each row a in ba for each row b in bb if (match_join_condition(a,b)) write_result_in_output(a,b) end if end for end for end for end for
In this case, the time complexity remains the same, but the number of disk accesses decreases: (the number of external groups + the number of external groups * the number of internal groups). As the size of the groups increases, the number of disk accesses decreases even more.
Note: in this algorithm, a larger amount of data is read with each call, but this does not matter, since the calls are sequential. - Hash join. This is a more complicated operation, but in many cases its cost is lower.
The algorithm is as follows:- All elements are read from the internal dependency.
- A hash table is created in memory.
- One by one, all the elements from the external dependency are read.
- A hash is calculated for each element (using the corresponding function from the hash table) so that the corresponding block can be found in the internal dependency.
- Elements from a block are compared with elements from an external dependency.
To evaluate this algorithm in terms of time complexity, several assumptions need to be made:- The internal dependency contains X blocks.
- The hash function distributes hashes almost equally for both dependencies. That is, all blocks have the same size.
- The cost of finding a correspondence between elements of an external dependency and all elements inside a block is equal to the number of elements inside the block.
Then the time complexity will be equal to:
(M / X) * (N / X) + the cost of
creating the hash table (M) + the cost of the hash function * N And if the hash function creates enough small blocks, then the time complexity will be O (M + N )
There is another hash join method that saves more memory and does not require more I / O:- Hash tables are calculated for both dependencies.
- Put on the disk.
- And then they are compared behaviorally with each other (one block is loaded into memory, and the second is read line by line).
Merge Merge. This is the only way to combine, which results in the data being sorted. In the framework of this article, we consider a simplified case when the dependencies are not divided into external and internal, since they behave the same way. However, in life they can differ, say, when working with duplicates.
The merge operation can be divided into two stages:- (Optional) the merge is first performed by sorting, when both sets of input data are sorted by the combination keys.
- Then merge is carried out.
Sorting
The merge sorting algorithm has already been discussed above, in this case it justifies itself if it is important for you to save memory.
But it happens that data sets come in already sorted, for example:- If the table is organized natively.
- If the dependency is an index in the presence of a join condition.
- If combining occurs with an intermediate sorted result.
Merge Merge
This operation is very similar to the merge operation in the merge sort procedure. But instead of selecting all the elements of both dependencies, we select only equal elements.- The two current elements of both dependencies are compared.
- If they are equal, then they are entered in the resulting table, and then the next two elements are compared, one from each dependency.
- If they are not equal, then the comparison is repeated, but instead of the smallest of the two elements, the next element is taken from the same dependence, since the probability of coincidence in this case is higher.
- Steps 1-3 are repeated until the elements of one of the dependencies end.
If both dependencies are already sorted, then the time complexity is O (N + M).
If both dependencies still need to be sorted, then the time complexity is O (N * Log (N) + M * Log (M)).
This algorithm works well because both dependencies are already sorted, and we don’t have to go back and forth through them. However, some simplification is allowed here: the algorithm does not handle situations when the same data occurs multiple times, that is, when multiple matches occur. In reality, a more complex version of the algorithm is used. For instance:mergeJoin(relation a, relation b) relation output integer a_key:=0; integer b_key:=0; while (a[a_key]!=null and b[b_key]!=null) if (a[a_key] < b[b_key]) a_key++; else if (a[a_key] > b[b_key]) b_key++; else //Join predicate satisfied write_result_in_output(a[a_key],b[b_key]) //We need to be careful when we increase the pointers integer a_key_temp:=a_key; integer b_key_temp:=b_key; if (a[a_key+1] != b[b_key]) b_key_temp:= b_key + 1; end if if (b[b_key+1] != a[a_key]) a_key_temp:= a_key + 1; end if if (b[b_key+1] == a[a_key] && b[b_key] == a[a_key+1]) a_key_temp:= a_key + 1; b_key_temp:= b_key + 1; end if a_key:= a_key_temp; b_key:= b_key_temp; end if end while
Which algorithm to choose?
If there were a better way to combine, then all of these varieties would not exist. So the answer to this question depends on a bunch of factors:
- The amount of available memory . If it's not enough, forget about the powerful hash join. At least about its implementation entirely in memory.
- The size of two sets of input data. If you have one table large and the second very small, then joining with nested loops will work the fastest because hash join involves an expensive procedure for creating hashes. If you have two very large tables, then joining using nested loops will consume all processor resources.
- Availability of indices. If you have two B-tree indexes, it is best to use merge union.
- Do I need to sort the result. You might want to use the expensive merge union (with sorting) if you are working with unsorted datasets. Then at the output you will get sorted data that is more convenient to combine with the results of another union. Or because the query indirectly or explicitly involves retrieving data sorted by ORDER BY / GROUP BY / DISTINCT statements.
- Are output dependencies sorted . In this case, it is better to use merge merging.
- Dependencies of what types you use . Equivalence union (table A. column 1 = table B. column 2)? Internal dependencies, external, Cartesian product or self-join? In different situations, some combination methods do not work.
- Data distribution . If the data is rejected by the join condition (for example, you combine people by last name, but namesake names are often found), then in no case should you use a hash join. Otherwise, the hash function will create baskets with very poor internal distribution.
- Do I need to combine into several processes / threads.
The hungry for knowledge can go deeper into the documentation for DB2 , ORACLE, and SQL Server .
4.4.4. Simplified examples
Let's say we need to combine five tables to get a complete picture of some people. Everyone can have:
- Several mobile phone numbers.
- Multiple email addresses.
- Several physical addresses.
- Several bank account numbers.
That is, you need to quickly give an answer to this request:
SELECT * from PERSON, MOBILES, MAILS,ADRESSES, BANK_ACCOUNTS
WHERE
PERSON.PERSON_ID = MOBILES.PERSON_ID
AND PERSON.PERSON_ID = MAILS.PERSON_ID
AND PERSON.PERSON_ID = ADRESSES.PERSON_ID
AND PERSON.PERSON_ID = BANK_ACCOUNTS.PERSON_ID
The optimizer needs to find the best way to process the data. But there are two problems:
- Which way to combine to use? There are three options (hash join, merge join, join using nested loops), with the possibility of using 0, 1 or 2 indexes. Not to mention that indexes can also be different.
- In what order do you need to merge?
For example, the following are possible plans for three joins of four tables:
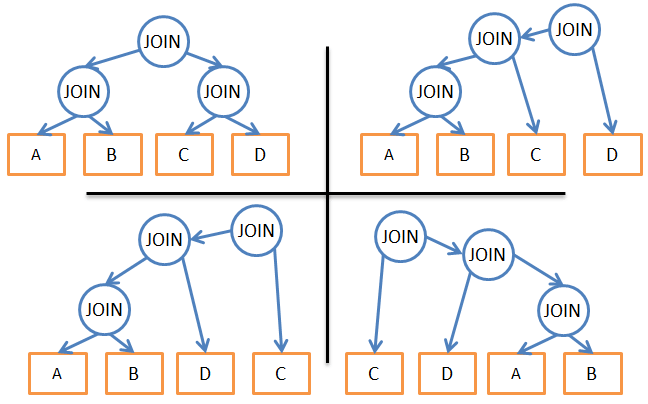
Based on the described, what are the options?
- Use the brute force approach. Using statistics, calculate the cost of each of the possible plans for fulfilling the request and choose the cheapest one. But there are quite a few options. For each merger order, you can use three different merge methods, for a total of 34 = 81 possible execution plans. In the case of a binary tree, the task of choosing the union order turns into a permutation problem, and the number of options is (2 * 4)! / (4 + 1)! .. As a result, in this very simplified example, the total number of possible plans for executing the request is 34 * (2 * 4)! / (4 + 1)! = 27,216. If you add to this, when merging uses 0, 1, or 2 B-tree indexes, the number of possible plans rises to 210,000. Have we already mentioned that this is a VERY SIMPLE request?
- Cry and quit. Very tempting, but unproductive, and money is needed.
- Try a few plans and choose the cheapest. Once it’s impossible to calculate the cost of all possible options, you can take an arbitrary test data set and run all kinds of plans on it to evaluate their cost and choose the best one.
- Apply smart rules to reduce the number of possible plans.
There are two types of rules:- "Logical", with which you can exclude useless options. But they are far from always applicable. For example, “when combined using nested loops, the internal dependency should be the smallest set of data.”
- You can not look for the most profitable solution and apply more stringent rules to reduce the number of possible plans. Say, “if the dependency is small, we use join using nested loops, but never merge join or hash join”.
Even such a simple example presents us with a huge choice. But other relationship operators may be present in real queries: OUTER JOIN, CROSS JOIN, GROUP BY, ORDER BY, PROJECTION, UNION, INTERSECT, DISTINCT, etc. That is, the number of possible execution plans will be even greater.
So how does a database make a choice?
4.4.5. Dynamic programming, “greedy” algorithm and heuristic.
Relational database uses different approaches, which were mentioned above. And the optimizer’s task is to find the right solution for a limited time. In most cases, the optimizer is not looking for the best, but just a good solution.
Bruteforce might work with small queries. And thanks to ways to eliminate unnecessary calculations, even for medium-sized queries, you can use brute force. This is called dynamic programming.
Its essence is that many execution plans are very similar.
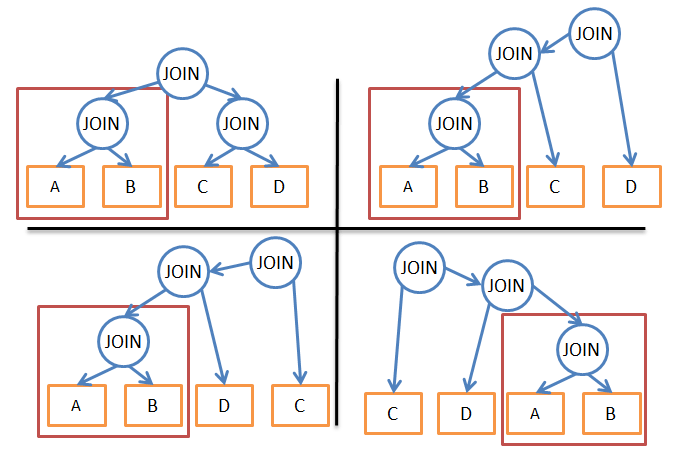
In this illustration, all four plans use the A JOIN B subtree. Instead of calculating its cost for each plan, we can only calculate it once and then use this data as much as needed. In other words, with the help of memoization, we solve the problem of overlap, that is, we avoid unnecessary calculations.
Thanks to this approach, instead of time complexity (2 * N)! / (N + 1)! we get “only” 3 N. In relation to the previous example with four associations, this means a decrease in the number of options from 336 to 81. If you take a query with 8 associations (a small query), then the complexity will decrease from 57 657 600 to 6 561.
If you are already familiar with dynamic programming or algorithmization, you can play around with this algorithm:
procedure findbestplan(S)
if (bestplan[S].cost infinite)
return bestplan[S]
// else bestplan[S] has not been computed earlier, compute it now
if (S contains only 1 relation)
set bestplan[S].plan and bestplan[S].cost based on the best way
of accessing S /* Using selections on S and indices on S */
else for each non-empty subset S1 of S such that S1 != S
P1= findbestplan(S1)
P2= findbestplan(S - S1)
A = best algorithm for joining results of P1 and P2
cost = P1.cost + P2.cost + cost of A
if cost < bestplan[S].cost
bestplan[S].cost = cost
bestplan[S].plan = “execute P1.plan; execute P2.plan;
join results of P1 and P2 using A”
return bestplan[S]
Dynamic programming can also be used for larger queries, but additional rules (heuristics) will have to be introduced to reduce the number of possible plans:
- If we analyze only any one type of plans (for example, following on the left branches of a tree), instead of 3 N we obtain the complexity at the level of N * 2 N .
- Adding logical rules (“if the table is an index for a given predicate, use the merge join only for the index, not the table”) helps reduce the number of options with little risk of excluding the best solutions.
- Adding rules “on the fly” (for example, “perform union operations BEFORE all other relationship operations”) also greatly helps to reduce the breadth of choice.
Greedy algorithms
But if the request is very large, or if we need to get a response extremely quickly, another class of algorithms is used - greedy.
In this case, the query execution plan is constructed step by step using a certain rule (heuristic). Thanks to him, the greedy algorithm is looking for the best solution for each stage individually. The plan begins with a JOIN statement, and then at each stage a new JOIN is added in accordance with the rule.
Consider a simple example. Take a query with 4 joins of 5 tables (A, B, C, D, and E). Simplify the problem somewhat and imagine that the only option is to combine using nested algorithms. We will use the rule "apply the least cost combination".
- We start with one of the tables, for example, A.
- We calculate the cost of each association with A (it will be both in the role of external and internal dependence).
- We find that the cheapest is A JOIN B.
- Then we calculate the cost of each association with the result A JOIN B (we also consider it in two roles).
- We find that (A JOIN B) JOIN C will be most profitable.
- Again, we evaluate the options.
- ...
- At the end we get the following plan for executing the request: (((A JOIN B) JOIN C) JOIN D) JOIN E) /
The same algorithm can be used to evaluate options starting with table B, then with C, etc. As a result, we get five plans, from which we choose the cheapest.
This algorithm is called the “ nearest neighbor algorithm ”.
We will not go into details, but with a good simulation of the complexity of sorting N * log (N), this problem can be easily solved . The time complexity of the algorithm is O (N * log (N)) instead of O (3 N ) for the full version with dynamic programming. If you have a large query with 20 associations, then this will be 26 against 3,486,784,401. A big difference, right?
But there is a nuance. We assume that if we find the best way to combine the two tables, we will get the lowest cost when combining the result of previous joins with the following tables. However, even if A JOIN B is the cheapest option, then (A JOIN C) JOIN B may have a lower cost than (A JOIN B) JOIN C.
So if you desperately need to find the cheapest plan of all time, you can advise to repeatedly run greedy algorithms using different rules.
Other Algorithms
If you are already fed up with all of these algorithms, you can skip this chapter. It is not required for the assimilation of all other material.
Many researchers deal with the problem of finding the best query execution plan. Often they try to find solutions for specific tasks and patterns. For example, for star-shaped joins, execution of parallel queries, etc.
We are looking for options for replacing dynamic programming to execute large queries. The same greedy algorithms are a subset of heuristic algorithms. They act in accordance with the rule, remember the result of one stage and use it to find the best option for the next stage. And algorithms that do not always use the solution found for the previous stage are called heuristic.
An example is genetic algorithms:
- Each decision is a plan for the full execution of the request.
- Instead of one solution (plan), the algorithm at each stage generates X decisions.
- First, X plans are created, this is done randomly.
- Of these, only those plans are preserved whose value satisfies a certain criterion.
- Then these plans are mixed to create X new plans.
- Some of the new plans are modified randomly.
- The previous three steps are repeated Y times.
- From the plans of the last cycle, we get the best.
The more cycles, the cheaper the plan can be calculated. Natural selection, fixing signs, that’s all.
By the way, genetic algorithms are integrated into PostgreSQL .
The database also uses heuristic algorithms such as Simulated Annealing, Iterative Improvement, and Two-Phase Optimization. But it’s not a fact that they are used in corporate systems, perhaps their destiny is research products.
4.4.6. Real optimizers
Also an optional chapter, you can skip.
Let's move away from theorizing and consider real examples. For example, how SQLite optimizer works. This is a “lightweight” database that uses simple optimization based on a greedy algorithm with additional rules:
- SQLite never changes the order of tables in a CROSS JOIN operation.
- Used union using nested loops.
- External associations are always evaluated in the order in which they were carried out.
- Up to version 3.8.0, the greedy Nearest Neighor algorithm is used to find the best plan for executing a request. And from version 3.8.0, “ N nearest neighbors ” (N3, N Nearest Neighbors) are used.
Here is another example. If you read the IBM DB2 documentation , we will find out that its optimizer uses 7 different levels of optimization:
- Greedy Algorithms:
- 0 - minimum optimization. It uses index scanning, combining using nested loops, and eliminating the overwrite of some queries.
- 1 - low optimization
- 2 - full optimization
- Dynamic programming:
- 3 - average optimization and rough approximation
- 5 - full optimization, all heuristic techniques are used
- 7 - the same, but without heuristic
- 9 - maximum optimization at any cost, without regard to the efforts expended. All possible methods of unification are evaluated, including Cartesian products.
The default level is 5. This includes:
- Collection of all possible statistics, including frequency distributions and quantiles.
- Application of all rules for rewriting queries, including creating a tabular route for materialized queries). The exception is rules requiring intensive computations, applied for a very limited number of cases.
- When enumerating union options using dynamic programming:
- Limited use of compound internal dependency.
- For star-shaped schemes with conversion tables, Cartesian products are used to a limited extent.
- We consider a wide range of access methods, including prefetching the list (more on that below), a special operation with AND indices, and compiling a tabular route for materialized queries.
Of course, developers do not share details about the heuristics used in their product, because the optimizer is perhaps the most important part of the database. However, it is known that, by default, dynamic programming is used to determine the join order, limited by heuristics.
Other conditions (GROUP BY, DISTINCT, etc.) are processed by simple rules.
4.4.7. Query plan cache
Because planning takes time, most databases store the plan in the query plan cache. This helps to avoid unnecessary calculations of the same steps. The database should know exactly when it needs to update outdated plans. To do this, a certain threshold is set, and if changes in statistics exceed it, then the plan related to this table is deleted from the cache.
Query Executor
At this stage, our plan is already optimized. It is recompiled into executable code and, if there are enough resources, is executed. The operators contained in the plan (JOIN, SORT BY, etc.) can be processed both sequentially and in parallel, the decision is made by the executor. It interacts with the data manager to receive and record data.
5. Data Manager
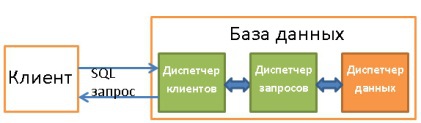
The query manager executes the query and needs data from tables and indexes. He requests them from the data manager, but there are two difficulties:
- Relational databases use a transactional model. It is impossible to get any desired data at a particular time, because at that time they can be used / modified by someone.
- Data extraction is the slowest operation in the database. Therefore, the data manager needs to be able to predict its work in order to fill the memory buffer in a timely manner.
5.1. Cache manager
As has been said more than once, the most bottleneck in the database is the disk subsystem. Therefore, a cache manager is used to increase performance.
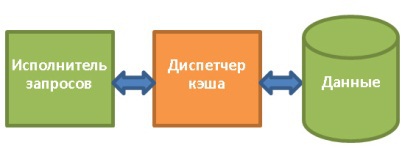
Instead of receiving data directly from the file system, the requestor requests it from the cache manager. He uses the buffer pool contained in the memory, which allows to drastically increase the database performance. In numbers, this is difficult to assess, since much depends on what you need:
- Serial access (full scan) or random access (access by line ID).
- Read or write.
Also of great importance is the type of drives used in the disk system: “hard drives” with different spindle speeds, SSDs, the presence of RAID in different configurations. But we can say that the use of memory is 100-100,000 times faster than disk.
However, here we are faced with another problem. The cache manager needs to put data in memory BEFORE the request executor needs it. Otherwise, he will have to wait for them to be received from the slow disk.
5.1.1. Anticipation
The query executor knows what data he will need, since he knows the whole plan, what data is contained on the disk and statistics.
When the executor processes the first batch of data, it asks the cache manager to preload the next batch. And when he proceeds to its processing, he asks the DC to load the third one and confirms that the first portion can be deleted from the cache.
The cache manager stores this data in the buffer pool. He also adds to them the service information (trigger, latch) to know whether they are still needed in the buffer.
Sometimes the contractor does not know what data he will need, or some databases do not have such functionality. Then, speculative prefetching is used (for example, if the contractor requests data 1, 3, 5, then it will probably request 7, 9, 11 in the future) or sequential prefetching (in this case, the DC simply loads the next a chunk of data.
To control the quality of lead in modern databases, the metric “buffer / cache utilization factor” (buffer / cache hit ratio) is used. It shows how often the requested data ends up in the cache, without the need to access the disk. However, a low coefficient value does not always indicate poor cache usage. Read more about this in the Oracle documentation .
Do not forget that the buffer is limited by the amount of available memory. That is, to load some data, we have to periodically delete others. Filling and flushing the cache consumes some of the resources of the disk subsystem and network. If you have a frequently executed query, it would be counterproductive to load and clear the data it uses every time. To solve this problem, modern databases use a buffer replacement strategy.
5.1.2. Buffer replacement strategies
Most databases (at least SQL Server, MySQL, Oracle, and DB2) use the LRU (Least Recently Used) algorithm for this. It is designed to maintain in the cache those data that were recently used, which means that it is likely that they may be needed again.
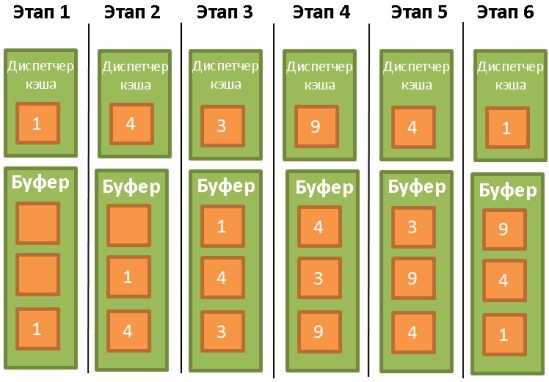
To facilitate understanding of the algorithm, we assume that the data in the buffer is not blocked by triggers (latch), and therefore can be deleted. In our example, the buffer can store three pieces of data:
- The cache manager uses data 1 and puts it in an empty buffer.
- Then it uses data 4 and also sends them to the buffer.
- The same thing is done with data 3.
- Next, data is taken 9. But the buffer is already full. Therefore, data 1 is deleted from it, since it has not been used for the longest. After that, data 9 is placed in the buffer.
- The cache manager uses the data 4 again. It is already in the buffer, so it is marked as last used.
- Data 1 is again in demand. In order to put it into the buffer, data 3 is deleted from it, as if it were not used the longest.
This is a good algorithm, but it has some limitations. What if we have a full scan of a large table? What happens if the size of the table / index exceeds the size of the buffer? In this case, the algorithm will delete all its contents from the cache, so the full scan data will most likely be used only once.
Algorithm Improvements
To prevent this from happening, some databases use special rules. According to Oracle documentation :
«Для очень больших таблиц обычно используется прямой доступ, то есть блоки данных считываются напрямую, чтобы избежать переполнения буфера кэша. Для таблиц среднего размера может использоваться как прямой доступ, так и чтение из кэша. Если система решит использовать кэш, то БД помещает блоки данных в конец списка LRU, чтобы предотвратить очистку буфера».
An improved version of LRU - LRU-K is also used. SQL Server uses LRU-K at K = 2. The essence of this algorithm is that when evaluating a situation, it takes into account more information about past operations, and not only remembers the last data used. The letter K in the name means that the algorithm takes into account which data was used the last K times. They are assigned a certain weight. When new data is loaded into the cache, the old, but often used data is not deleted, because its weight is higher. Of course, if the data is no longer used, it will still be deleted. And the longer the data remains unclaimed, the more its weight decreases over time.
Calculation of the weight is rather expensive, therefore, SQL Server uses LRU-K with K equal to only 2. With a slight increase in K, the efficiency of the algorithm improves. You can get to know him better thanks to the work of 1993 .
Other Algorithms
Of course, LRU-K is not the only solution. There are also 2Q and CLOCK (both similar to LRU-K), MRU (Most Recently Used, which uses LRU logic, but another rule is applied, LRFU (Least Recently and Frequently Used), etc. In some databases, you can choose which algorithm will be used
5.1.3.
We talked only about the read buffer, but DBs also use write buffers, which accumulate data and flush portions to the disk instead of sequential write. This saves I / O.
Remember that buffers store pages (indivisible data units), not rows from tables. A page in the buffer pool is called “dirty” if it is modified but not written to disk. There are many different algorithms with which you select the time to record dirty pages. But this is largely due to the concept of transactions.
5.2. Transaction manager
His responsibilities include tracking so that each request is executed using his own transaction. But before we talk about the dispatcher, let's clarify the concept of ACID transactions.
5.2.1. “Under acid” (pun, if someone doesn’t understand) An
ACID transaction (Atomicity, Isolation, Durability, Consistency) is an elementary operation, a unit of work that satisfies 4 conditions:
- Atomicity There is nothing less than a transaction, no smaller operation. Even if the transaction lasts 10 hours. In case of unsuccessful transaction, the system returns to the “before” state, that is, the transaction is rolled back.
- Изолированность (Isolation). Если в одно время выполняются две транзакции А и В, то их результат не должен зависеть от того, завершилась ли одна из них до, во время или после исполнения другой.
- Надёжность (Durability). Когда транзакция зафиксирована (commited), то есть успешно завершена, использовавшиеся ею данные остаются в БД вне зависимости от возможных происшествий (ошибки, падения).
- Консистентность (согласованность) (Consistency). В БД записываются только валидные данные (с точки зрения реляционных и функциональных связей). Консистентность зависит от атомарности и изолированности.

During the execution of a transaction, you can execute various SQL queries for reading, creating, updating and deleting data. Problems begin when two transactions use the same data. A classic example is the transfer of money from account A to account B. Let's say we have two transactions:
- T1 takes $ 100 from account A and sends them to account B.
- T2 takes $ 50 from account A and also sends them to account B.
Now consider this situation in terms of ACID properties:
- Atomicity allows you to be sure that no matter what happens during T1 (server crash, network failure), it cannot happen that $ 100 will be debited from A, but will not come to B (otherwise they say “inconsistent state”) .
- Isolation suggests that even if T1 and T2 are carried out simultaneously, as a result, $ 100 will be debited from A and the same amount will go to B. In all other cases, they again speak of an inconsistent state.
- Reliability allows you not to worry about the fact that T1 will disappear if the base falls immediately after the T1 commit.
- Consistency prevents the ability to create money or destroy it in the system.
You can not read below, it is no longer important for understanding the rest of the material.
Many databases do not provide complete isolation by default, as this leads to huge performance overheads. SQL uses 4 isolation levels:
- Сериализуемые транзакции (Serializable). Наивысший уровень изолированности. По умолчанию используется в SQLite. Каждая транзакция исполняется в собственной, полностью изолированной среде.
- Повторяемое чтение (Repeatable read). По умолчанию используется в MySQL. Каждая транзакция имеет свою среду, за исключением одной ситуации: если транзакция добавляет новые данные и успешно завершается, то они будут видимы для других, всё ещё выполняющихся транзакций. Но если транзакция модифицирует данные и успешно завершается, то эти изменения будут не видны для всё ещё выполняющихся транзакций. То есть для новых данных принцип изолированности нарушается.
Например, транзакция А выполняетSELECT count(1) from TABLE_X
Потом транзакция Б добавляет в таблицу Х и коммитит новые данные. И если после этого транзакция А снова выполняет count(1), то результат будет уже другим.
Это называется фантомным чтением. - Чтение зафиксированных данных (Read commited). По умолчанию используется в Oracle, PostgreSQL и SQL Server. Это то же самое, что и повторяемое чтение, но с дополнительным нарушением изолированности. Допустим, транзакция А читает данные; затем они модифицируются или удаляются транзакцией Б, которая коммитит эти действия. Если А снова считает эти данные, то она увидит изменения (или факт удаления), сделанные Б.
Это называется неповторяемым чтением (non-repeatable read). - Чтение незафиксированных данных (Read uncommited). Самый низкий уровень изолированности. К чтению зафиксированных данных добавляется новое нарушение изолированности. Допустим, транзакция А читает данные; затем они модифицируются транзакцией Б (изменения не коммитятся, Б всё ещё выполняется). Если А считает данные снова, то увидит сделанные изменения. Если же Б будет откачена назад, то при повторном чтении А не увидит изменений, словно ничего и не было.
Это называется грязным чтением.
Most databases add their own isolation levels (for example, based on snapshots, as in PostgreSQL, Oracle and SQL Server). Also, in many databases, all four of the above levels are not implemented, especially reading uncommitted data.
The user or developer can immediately override the default isolation level after establishing a connection. To do this, just add a very simple line of code.
5.2.2. Concurrency management
The main thing for which we need isolation, consistency and atomicity is the ability to write operations on the same data (add, update and delete).
If all transactions will only read data, they will be able to work simultaneously, without affecting other transactions.
If at least one transaction changes the data read by other transactions, then the database needs to find a way to hide these changes from them. You also need to make sure that the changes made will not be deleted by other transactions that do not see the changed data.
This is called concurrency management.
The easiest way is to simply execute transactions one at a time. But this approach is usually inefficient (only one core of one processor is involved), and besides, the ability to scale is lost.
The ideal way to solve the problem is as follows (each time a transaction is created or canceled):
- Monitor all operations of each transaction.
- If two or more transactions conflict due to reading / changing the same data, then change the sequence of operations within the parties to the conflict in order to minimize the number of reasons.
- Выполнять конфликтующие части транзакций в определённом порядке. Неконфликтующие транзакции в это время выполняются параллельно.
- Иметь в виду, что транзакции могут быть отменены.
If you approach the issue more formally, then this is a problem of a conflict of schedules. It is very difficult to solve, and optimization requires large processor resources. Corporate databases cannot afford to spend hours searching for the best schedule for each new transaction. Therefore, less sophisticated approaches are used in which more time is spent on conflicts.
5.2.3. Lock Manager
To solve the above problem, many databases use locks and / or data versioning.
If transactions need some data, then it blocks them. If they also needed another transaction, then it will have to wait until the first transaction releases the lock.
This is called an exclusive lock.
But it’s too wasteful to use exclusive locks in cases where transactions only need to read data. Why bother reading data? In such cases, shared locks are used. If transactions need to read data, they apply a joint lock to them and reads. This does not prevent other transactions from using joint locks and reading data. If any of them needs to change the data, then she will have to wait until all joint locks are released. Only then can she apply an exclusive lock. And then all other transactions will have to wait for its withdrawal in order to read this data.
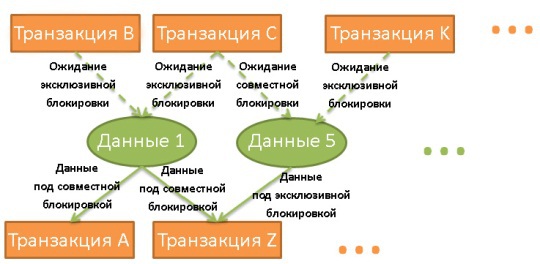
A lock manager is a process that applies and removes locks. They are stored in a hash table (the keys are locked data). The dispatcher knows for all data which transactions have applied locks or are waiting for them to be removed.
Deadlock
Using locks can lead to a situation where two transactions endlessly wait for locks to be released:
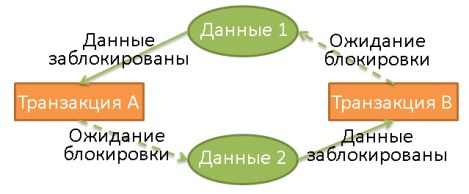
Here, transaction A exclusively blocked data 1 and expects data to be released 2. At the same time, transaction B exclusively blocked data 2 and expects data to be released 1.
When to a deadlock, the dispatcher chooses which transaction to cancel (roll back). And the decision to make is not so simple:
- Would it be better to kill a transaction that changed the last data set (which means rollback would be the least painful)?
- Would it be better to kill the youngest transaction, as users of other transactions have waited longer?
- Would it be better to kill a transaction that takes less time to complete?
- How many other transactions will rollback affect?
But before making a decision, the dispatcher must make sure that a deadlock has actually occurred.
Imagine the hash table in the form of a diagram, as in the illustration above. If there is a cyclic link in the diagram, the deadlock is confirmed. But since checking for the presence of cycles is quite expensive (because the diagram on which all the locks are displayed will be very large), a simpler approach is often used: the use of timeouts. If the lock is not released within a certain time, then the transaction has entered a state of mutual lock.
Before imposing a lock, the dispatcher can also check if this will lead to a deadlock. But in order to unequivocally answer this, one also has to spend money on calculations. Therefore, such pre-checks are often presented as a set of basic rules.
Two-phase locking The
easiest way to ensure complete isolation is when the lock is applied at the beginning and is removed at the end of the transaction. This means that transactions have to wait until all locks are released before starting, and the locks used by it are released only at the end. This approach can be applied, but then a lot of time is wasted on all these expectations of unlocking.
DB2 and SQL Server use a two-phase locking protocol, in which a transaction is divided into two phases:
- A phase of growth (growing phase) , when a transaction can only apply locks, but not remove them.
- A shrinking phase when a transaction can only release locks (from data that has already been processed and will not be processed again), but not apply new ones.
A frequent conflict that occurs in the absence of a two-phase lock:

Before transaction A X = 1 and Y = 1. It processes the data Y = 1, which was changed by transaction B after the start of transaction A. Due to the principle of isolation, transaction A must process Y = 2 .
Aims to be solved with the help of these two simple rules:
- Release locks that are no longer needed to reduce the latency of other transactions.
- To prevent cases when a transaction receives data modified by a previously launched transaction. Such data does not match the requested.
This protocol works great, except in a situation where the transaction modified the data and removed the lock from it, after which it was canceled. At the same time, another transaction can read and change data, which will then be pumped back. To avoid this situation, all exclusive locks must be removed at the end of the transaction.
Of course, real databases use more complex systems, more types of locks and with greater granularity (blocking rows, pages, partitions, tables, table spaces), but the essence is the same.
Data Versioning
Another way to solve the transaction conflict problem is to use data versioning.
- All transactions can modify the same data at the same time.
- Each transaction works with its own copy (version) of data.
- If two transactions change the same data, then only one of the modifications is accepted, the other will be rejected, and the transaction that made it will be rolled back (and maybe restarted).
This allows you to increase productivity, because:
- Reading transactions do not block writing, and vice versa.
- A hulking lock manager has no effect.
In general, anything will be better than locks, except when two transactions are writing the same data. Moreover, this can lead to a huge overspending of disk space.
Both approaches - locking and versioning - have pros and cons, a lot depends on the situation in which they are used (more reads or more records). You can study a very good presentation on the implementation of multi-version concurrency management in PostgreSQL.
Some databases (DB2 prior to version 9.7, SQL Server) use only locks. Others, like PostgreSQL, MySQL, and Oracle, use combined approaches.
Note: versioning has an interesting effect on indexes. Sometimes duplicates appear in a unique index, the index may contain more records than rows in the table, etc.
If part of the data is read at one level of isolation, and then it increases, then the number of locks increases, which means that more time is spent waiting for transactions. Therefore, most databases do not use the default maximum isolation level.
As usual, for more information, refer to the documentation: MySQL , PostgreSQL , Oracle .
5.2.4. Log manager
As we already know, for the sake of increasing productivity, the database stores part of the data in buffer memory. But if the server crashes during a transaction commit, then the data in memory will be lost. And this violates the principle of transaction reliability.
Of course, you can write everything to disk, but if you fall, you will be left with incomplete data, and this is a violation of the principle of atomicity.
Any changes recorded by the transaction must be rolled back or completed.
This is done in two ways:
- Shadow copies / pages. Each transaction creates its own copy of the database (or part of it), and works with this copy. In case of an error, the copy is deleted. If everything went well, then the database instantly switches to the data from the copy with a single trick at the file system level, and then deletes the "old" data.
- Transaction log This is a special repository. Before each write to disk, the database writes information to the transaction log. So in the event of a failure, the database will know how to delete or complete an incomplete transaction.
WAL
In large databases with many transactions, shadow copies / pages take up an incredible amount of space in the disk subsystem. Therefore, in modern databases, a transaction log is used. It should be located in a fault-proof repository.
Most products (in particular, Oracle, SQL Server , DB2 , PostgreSQL , MySQL, and SQLite ) work with the transaction log through the WAL (Write-Ahead Logging) protocol. This protocol contains three rules:
- Каждая модификация в БД должна сопровождаться записью в лог, и она должна вноситься ДО того, как данные будут записаны на диск.
- Записи в логе должны располагаться в соответствии с очерёдностью соответствующих событий.
- Когда транзакция коммитится, запись об этом должна вноситься в лог ДО момента успешного завершения транзакции.
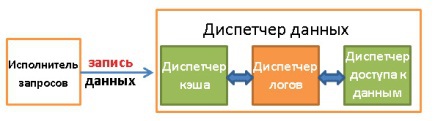
These rules are monitored by the log manager. Logically, it is located between the cache manager and the data access manager. The log manager registers every operation performed by transactions until it is written to disk. It seems right?
WRONG! After everything that you and I went through in this article, it's time to remember that everything related to the database is subject to the curse of the “database effect”. Seriously, the problem is that you need to find a way to write to the log, while maintaining good performance. After all, if the transaction log is slow, then it slows down all other processes.
Aries
In 1992, IBM researchers created an extended version of WAL called the ARIES. In one form or another, ARIES is used by most modern databases. If you want to study this protocol more deeply, you can study the corresponding work .
Thus, ARIES stands for A lgorithms for R ecovery and I solation E xploiting S emantics. This technology has two tasks:
- Provide good logging performance.
- Provide fast and reliable recovery.
There are several reasons why a database has to roll back a transaction:
- User canceled it.
- Server or network error.
- The transaction violated the integrity of the database. For example, you applied a UNIQUE clause to a column, and the transaction added a duplicate.
- The presence of mutual locks.
But sometimes the database can also restore the transaction, for example, in the case of a network error.
How is this possible? To answer this, you first need to figure out what information is stored in the log.
Logs
Each operation (add / delete / change) during the execution of a transaction leads to the appearance of a log entry. The entry contains:
- LSN (Log Sequence Number) . This is a unique number whose value is determined in chronological order. That is, if operation A occurred before operation B, the LSN for A will be less than the LSN for B. In reality, the method of generating the LSN is more complicated, since it is also associated with the method of storing the log.
- TransID. Идентификатор транзакции, осуществившей операцию.
- PageID. Место на диске, где находятся изменённые данные.
- PrevLSN. Ссылка на предыдущую запись в логе, созданную той же транзакцией.
- UNDO. Способ отката операции.
Например, если была проведена операция обновления, то в UNDO записывается предыдущее значение/состояние изменённого элемента (физический UNDO) или обратная операция, позволяющая вернуться в предыдущее состояние (логический UNDO). В ARIES используется только логический, с физическим работать очень тяжело. - REDO. Способ повтора операции.
In addition, each page on the disk (for storing data, not the log) contains the LSN of the last operation, the data contained here is modified.
As far as we know, UNDO is not used only in PostgreSQL. Instead, a garbage collector that cleans up old versions of data is used. This is due to the implementation of data versioning in this DBMS.
To make it easier for you to imagine the composition of the entry in the log, here is a visual simplified example in which the UPDATE FROM PERSON SET AGE = 18; query is executed. Let it be executed in transaction number 18:

Each log has a unique LSN. Linked logs refer to the same transaction, and they are linked in chronological order (the last log of the list refers to the last operation).
Log buffer
To prevent the log entry from becoming a system bottleneck, the log buffer is used.
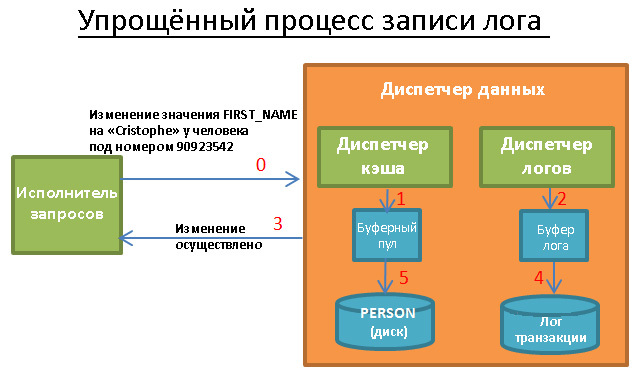
When the requestor requests the modified data:
- The cache manager stores them in a buffer.
- The log manager stores the corresponding log in its own buffer.
- The requestor determines whether the operation is completed and, accordingly, whether the changed data can be requested.
- The log manager saves the necessary information in the transaction log. The moment of making this entry is set by the algorithm.
- The cache manager writes the changes to disk. The moment of recording is also set by the algorithm.
When a transaction is committed, it means that all steps 1 to 5 have been completed. Logging to the transaction log is quick, because it is “adding a log somewhere to the transaction log”. At the same time, writing data to a disk is a more complicated procedure, taking into account that the data must subsequently be read quickly.
STEAL and FORCE Policies
In order to increase performance, step 5 should be done after a commit, because in case of a failure it is still possible to restore a transaction using REDO. This is called a NO-FORCE policy.
But the database can choose the FORCE policy to reduce the load during recovery. Then step number 5 is performed before the commit.
The database also selects whether to write data to the disk step by step (STEAL policy) or, if the buffer manager should wait for a commit, write everything all at once (NO-STEAL). The choice depends on what you need: fast recording with a long recovery or fast recovery?
How the policies mentioned affect the recovery process:
- STEAL / NO-FORCE needs UNDO and REDO. Performance is the highest, but more complex structure of logs and recovery processes (like ARES). This combination of policies is used by most databases.
- For STEAL / FORCE, only UNDO is needed.
- For NO-STEAL / NO-FORCE - only REDO.
- For NO-STEAL / FORCE nothing is needed at all. Performance in this case is the lowest, and a huge amount of memory is required.
Recovery
So, how can we use our wonderful logs? Suppose a new employee has destroyed the database (rule # 1: the newbie is always to blame!). You restart it and the recovery process begins.
ARIES restores in three steps:
- Analysis . The entire transaction log is read so that the chronology of events that occurred during the fall of the database can be restored. This helps determine which transaction needs to be rolled back. All transactions are rolled back without an order to commit. The system also decides which data should be written to disk during the crash.
- Повтор. Для обновления БД до состояния перед падением используется REDO. Его логи обрабатываются в хронологическом порядке. Для каждого лога считывается LSN страницы на диске, содержащей данные, которые нужно изменить.
Если LSN(страницы_на_диске)>=LSN(записи_в_логе), то значит данные уже были записаны на диск перед сбоем. Но значение было перезаписано операцией, которая была выполнена после записи в лог и до сбоя. Так что ничего не сделано, на самом деле.
Если LSN(страницы_на_диске)
Повтор выполняется даже для транзакций, которые будут откачены, потому что это упрощает процесс восстановления. Но современные БД наверняка этого не делают. - Cancel At this stage, all transactions that were not completed at the time of the failure are rolled back. The process begins with the last logs of each transaction and processes UNDO in reverse chronological order using PrevLSN.
During the recovery process, the transaction log should be aware of the actions performed during the recovery. This is necessary to synchronize the data stored on the disk by those recorded in the transaction log. You can delete transaction records that are rolled back, but it is very difficult to do. Instead, ARIES makes compensatory entries in the transaction log, logically invalidating the records of rolled back transactions.
If the transaction is canceled "manually" either by the lock manager or due to a network failure, then the analysis step is not needed. Indeed, the information for REDO and UNDO is contained in two tables located in memory:
- In the transaction table (here the status of all current transactions is stored).
- The table of dirty pages (here contains information about what data you need to write to disk).
As soon as a new transaction appears, these tables are updated by the cache manager and transaction manager. And since the tables are stored in memory, then when the database crashes, they disappear.
The analysis stage is needed just to restore both tables using information from the transaction log. To accelerate this step, ARIES uses breakpoints. The contents of both tables are written to the disk from time to time, as well as the last at the time of writing LSN. So during recovery, only the logs following that LSN are analyzed.
6. Conclusion
As an additional overview on databases, you can recommend the article Architecture of a Database System . This is a good introduction to the topic, written in a fairly understandable language.
If you carefully read all of the above material, then you probably got an idea of how great the capabilities of the databases are. However, this article does not address other important issues:
- How to manage clustered databases and global transactions.
- How to get a snapshot if the database is working.
- How to effectively store and compress data.
- How to manage memory.
So think twice before choosing between a buggy NoSQL and a solid relational database. Do not get it wrong, some NoSQL databases are very good. But they are still far from perfect and can only help in solving specific problems associated with some applications.
So, if someone asks you how the databases work, then instead of spitting and leaving, you can answer:

Or you can just give a link to this article.