The "functional imprint" of the brain can serve as its identifier throughout life
- Transfer
A unique neurological "functional imprint" allows scientists to study the effect on the structure of brain connections of genetics, the environment and aging.
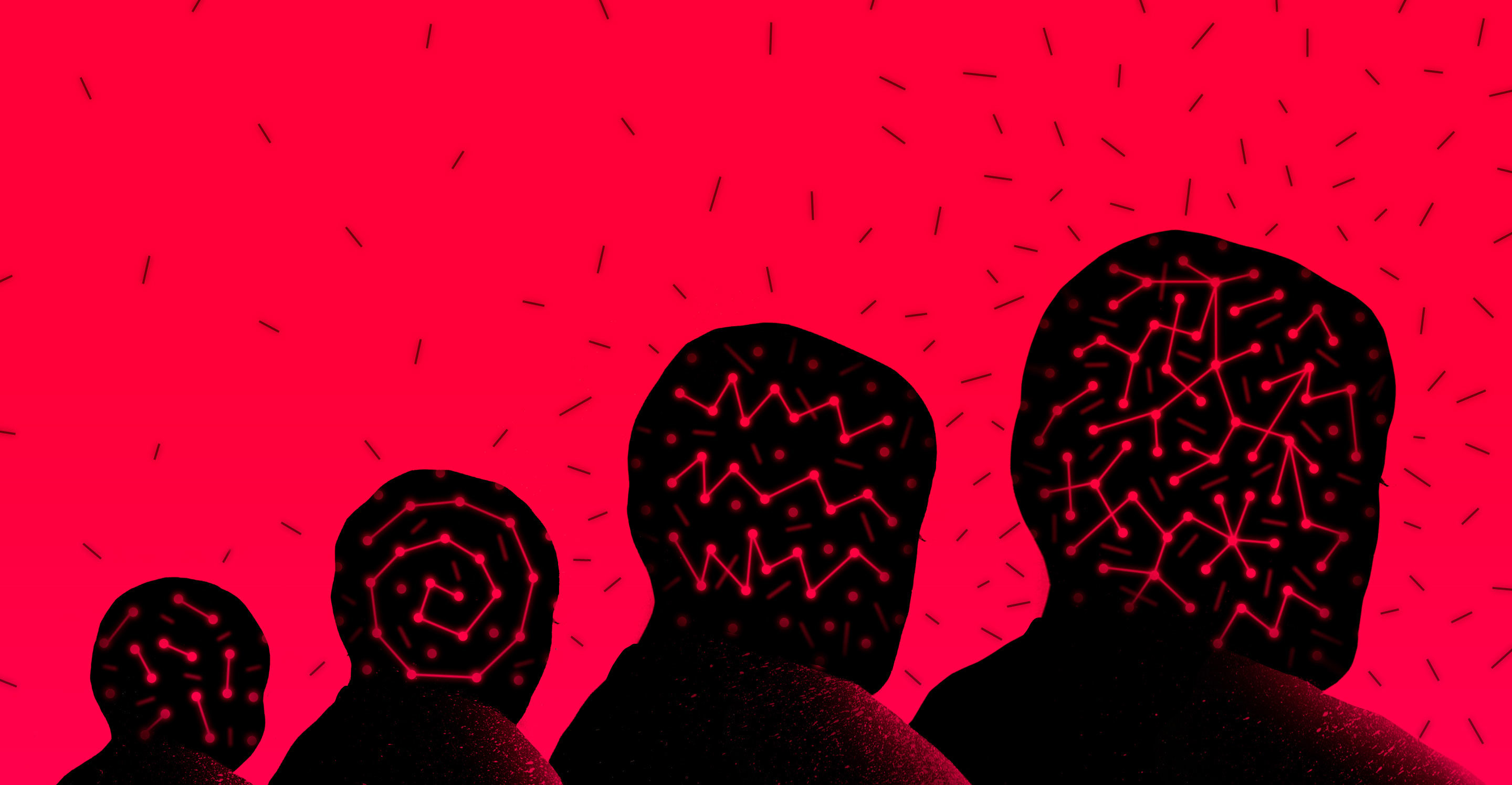
Michaela Cordova, a researcher and laboratory manager at the Oregon Institute of Health and Science, begins with demetallization: removes rings, watches, removes gadgets and other sources of metal, checks pockets for forgotten objects, which she says can “fly inside”. Then she enters the room with the scanner, raises and lowers the bed, waves her headband with sensors approximately in the direction of the viewing window and iPad camera, which allows to conduct this virtual tour (I observe what is happening from a distance of thousands of kilometers while in Massachusetts). Her voice is slightly distorted by the microphone built into the MRI scanner, which, from my slightly blurred point of view, does not look like an industrial-type tube, but more like a beast with a luminous blue jaw. I can't help thinking
Cordova works with children, calming their fears, helping them get into the scanner and get out of it, coaxing them with a kind word, cartoons from Pixar studios and promises of snacks in order to minimize their wriggling. These kids are involved in a study aimed at marking the neural connections of the brain.
The totality of the physical connections between parts of the brain is known as a “connection”, and is what cognitively distinguishes people from other species. But apart from that, these connections distinguish us from each other. Scientists are now combining neuroimaging and machine learning to understand how the structure and work of the brain of individuals differ and how to predict changes in a particular brain with time under the influence of genetics and the environment.
The laboratory where Cordoba works is led by associate professor Damien Fair at the functional connection — a map of brain areas involved in coordinating certain tasks and influencing behavior. Faire came up with the name of the distinctive neural connections of the personality: a functional imprint. As well as fingerprints, functional fingerprints are different for everyone, and can serve as a unique identifier.
“I can take an imprint from my five-year-old daughter, and find out that the imprint belongs to her, even when she is already 25 years old,” said Fair. And although her finger can grow, somehow change with age and the experience gained, "its main signs will not go anywhere." Similarly, the work of the Faire lab suggests that the essence of the human functional connection can be used as an identifier, and that normal changes in the brain during life can in principle be predicted.
Identifying, tracking, and modeling a functional connectom can reveal exactly how a brain device affects a person’s behavior, and, in some cases, leads to certain neuropsychiatric diseases. To do this, Faire and the team systematically scan in the search for patterns in connections data from scans, studies, and case histories.
Characterizing the connect
Traditional markup techniques of the functional connectom concentrate on just two areas of the brain at a time, using MRI to find correlations between activity changes in these areas. Parts of the brain with signals that change at the same time receive 1 point. If at one site the signal increases, and at another - decreases, it is -1 point. If there is no relationship between the sites, it is 0 points.
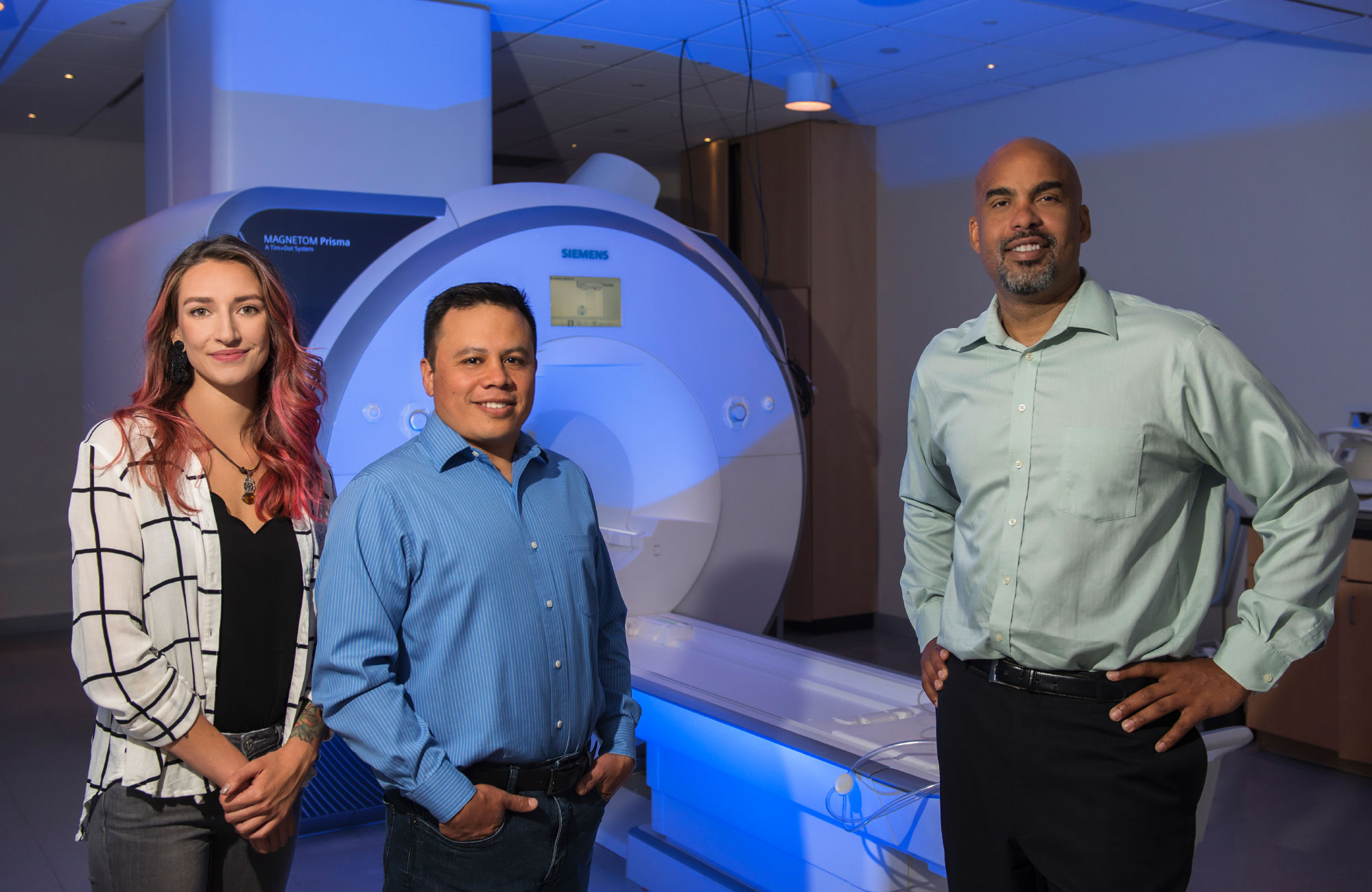
From left to right: Michaela Córdoba, Oscar Miranda-Dominguez and Damien Fair
This approach has its limitations. For example, he views a selected pair of brain regions independently of the others, although each of them probably depends on data coming from neighboring areas, and this additional data may mask the true functional relationship of any pair. To go beyond these assumptions, it was necessary to study the simultaneous communication of all parts of the brain, and not just some of their sample, and to find in their connections broader and more informative patterns that we might otherwise have missed.
In 2010, Fair was co-author of the work, published in Science, describing the use of machine learning and MRI scans in the simultaneous recording of all couples involved, to determine the age of a given brain. And although it was not the only team that analyzed the patterns of several communication channels at once, his work generated an active discussion in the research community, as she was the first to use these patterns to determine the age of an individual.
Four years later, in the work where the phrase “functional imprint” was coined, Fair's team developed its own method of marking the functional connection and predicting the activity of individual brain regions based on signals from not just one, but from all other sites in combination with each other.
In their simple linear model, the activity of one region of the brain is equal to the total contribution of all the other sites, each of which receives its own weight in accordance with the strength of the relationship between the two brain regions in question. Unique functional imprint make the relative contributions to the interaction of each of the brain regions. The researchers needed only 2.5 minutes per person to build a linear model based on high-quality MRI scans.
According to their calculations, about 30% of the connectom is unique to the individual. Most of the sites studied usually manage high-level tasks that require cognitive processing — learning, memory, and attention — compared to simpler functions like sensory, motor, or visual.
The fact that these areas are so different between people has its own meaning, Faire explained, since the regions that control high-level functions, in fact, make us what we are. In fact, such parts of the brain as the frontal and parietal lobes appeared on the late stages of evolution, and increased with the advent of modern man.
"If you think about what people are likely to have in common - it will obviously have some simpler functions," said Faire, "such as how I move my arms, or how visual information is processed." These areas do not vary so much in the human population.
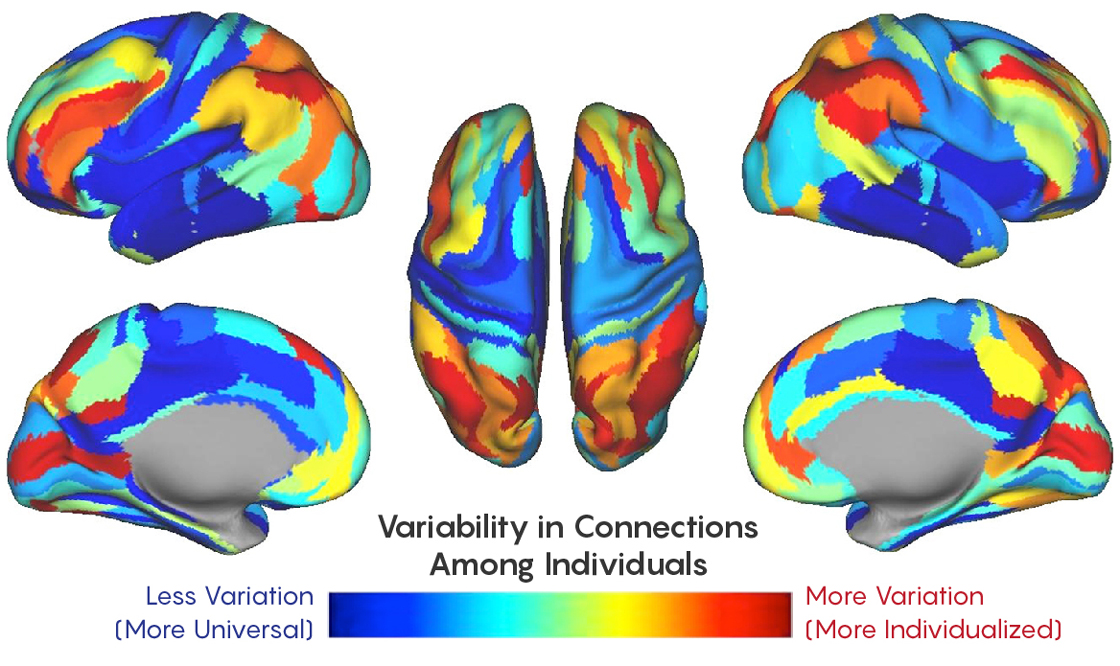
Closer to the blue part of the spectrum - less variations between different people, closer to red - more variations
Considering the unique patterns of activity in different parts of the brain, the model can identify an individual based on new scans made two weeks after the first. But what is a couple of weeks in relation to human life? Faire and the team began to think about whether a functional imprint of a person can exist for years or even generations.
If researchers could compare the functional imprint of a person with the imprints of his close relatives, they could distinguish between genetic influences and environmental influences that form our neural connections.
Tracking Neural Inheritance
The first step in establishing the connection between genes and the organization of the brain is to determine which aspects of the connectome are common among members of one family. There are nuances here - it is known that the brain structures of relatives are similar in volume, form and integrity of white matter , but this does not mean that the structure of the connections between these structures is the same. Since certain mental illnesses are characteristic of one family, Faire’s desire to determine the inherited connections ultimately can help to identify those parts of the brain and genes that increase the risk of certain diseases.
As they wrote in the work, published in June, the laboratory set out to create a machine learning platform capable of answering the question of whether the connections between different parts of the brain are more similar among relatives than random people.
Researchers rechecked their linear model on a new set of brain snapshots, this time, including children's snapshots, to ensure the relative stability of the connectom during adolescence. And it turned out that the model is really sensitive enough to identify individuals, despite the changes in neural connections that occur in the process of brain development over several years.
The study of the influence of genetics and the environment on brain circuits began with a classifier — a sorting algorithm that divides the subjects into two groups, “relatives” and “not relatives”, based on their functional imprints. The model was trained on children from Oregon, and then checked on another set of children's data, as well as on another sample, which included adults from the human connection project .
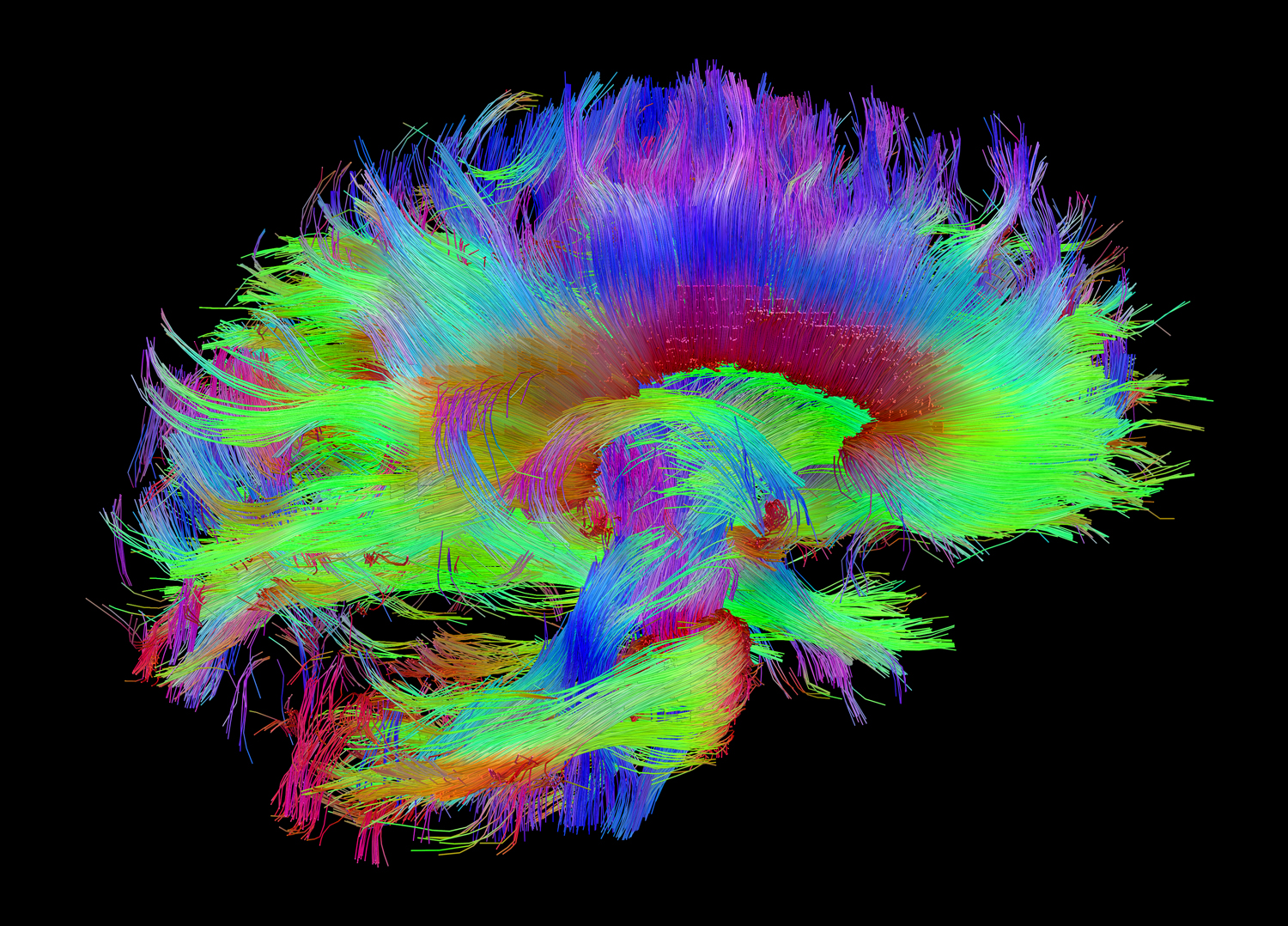
One of the images of anatomical connections between parts of the brain, obtained as part of a human connectome project
Just as a person can establish connections between people on the basis of physical signs such as eye color, hair color and height, the categorizer conducted a similar procedure based on neural connections. It turned out that functional prints are most similar in identical twins , then the differences increase in monopodal twins, then in ordinary brothers and sisters, and, finally, in people not related by kinship.
Adjunct Professor Oscar Miranda-Dominguez- an employee of the Faire laboratory and the first author of the study - was surprised that they were able to identify adult relatives using models trained on children. Trained on adult models could not do this - perhaps because adults have higher-level systems that are fully developed, and their features are worse summarized than those of the young, developing brain. “Further research with increasing data sets and a range of ages can clarify issues of growing up,” Miranda said.
The model’s ability to recognize small differences between family members, he added, turned out to be quite remarkable, since researchers coded the classifier for a more sketchy search for only “relatives” or “not relatives”, and not for discrimination of relationship degrees (their 2014 model was able to recognize these implicit differences , and more traditional correlation approaches failed).
Although their sample of twins was not large enough to understand the differences in the influence of genetics and the environment, Faire says that "there is no doubt" that the latter plays a large role in the formation of a functional imprint. In addition to the article, a model was described that can distinguish the general environment from general genetics, but without sufficiently large data sets, the team is still careful not to draw final conclusions. “Most of what we see relates more to genetics, and less to the environment,” Faire said, “but it’s not true that the environment doesn’t have much influence on the connection.”
To share the contributions of the common environment and common genetics, says Miranda, "you can, for example, find brain properties that distinguish identical twins from monopods, since both species have a common environment, but the genetic contribution is the same for identical twins."
Although all the nerve contours studied by them demonstrate a certain level of similarity between relatives, the systems of higher order differed in the greatest heritability. These turned out to be the same plots that demonstrated the greatest difference between relatives in a study that was carried out four years before. As Miranda pointed out, these plots regulate behavior that is responsible for social interaction, possibly predetermining “family characteristics”. To the list of family features, immediately after high blood pressure, arthritis and myopia, you can add "distributed brain activity."
Looking for a sign of age predicted by the brain
While in Oregon, Fair and Miranda describe the genetic basis of a functional connection, a researcher at King’s College London, James Cole, is working on deciphering the hereditary age of the brain using neuroimaging and machine learning. Faire’s team defines the age of the brain in terms of the functional connections between its parts, and Cole considers it as an atrophy index — the contraction of the brain over time. Over the years, the cells dry out or die, the neural volume decreases, and the skull does not change, and the emptying space is filled with cerebrospinal fluid. In a sense, after a certain stage of development, the brain grows old through shrinking.
In 2010, when Faire published an influential work in Science that caused excitement to delight in using data from fMRITo determine the age of the brain, one of Cole's colleagues led a similar project, the results of which were published in NeuroImage. He used anatomical data, since the difference between perceived and chronological brain ages (“gap of brain ages”) can be biologically informative.
According to Cole, age has a slightly different effect on every person, every brain, and even every type of cell. It is still unknown where this mosaic of aging comes from, but Cole says that in a sense we still do not know what aging is in principle. Over time, gene expression changes, as does metabolism, the work of cells and the change of cells. However, organs and cells can change independently; There is no single gene or hormone that controls the entire aging process.

James Cole
Although it is generally accepted that different people age at different speeds, the idea that different facets of one person may age differently looks more controversial. As Cole explained, there are many methods for measuring age, but so far few have been compared or combined. Perhaps exploring several different human tissues, scientists will be able to develop a more generalized age estimate. Cole's work is at the beginning of this journey, exploring images of brain tissue.
Cole’s theoretical platform is relatively straightforward: feeding the algorithm data from healthy people so that it learns to predict brain age from anatomical data, and then test the model on a new sample, subtracting the chronological age of the subjects from the brain age. If their brain age is higher than chronological, this suggests an accumulation of age-related changes, possibly associated with such illnesses as Alzheimer's disease .
In 2017, Cole used the Gaussian process regression algorithm .(Gaussian process regressions, GPR) for the formation of the age of the brain of each participant. This allowed him to compare his age estimates with other existing ones, for example, with the study of which parts of the genome are turned on and off by adding methyl groups at different ages. Biomarkers such as DNA methylation have already been used to predict mortality, and Cole suspects that the age of the brain can be used for this.
Indeed, individuals whose brains seemed older than chronological age were at greater risk due to poor physical and cognitive health. Cole was surprised to learn that a high brain age, derived from neuroimaging data, does not always correlate with a high methylation age. However, if both ages were found high in the subject, their risk of mortality was increased.
Later that year, Cole and his colleagues expanded their work.using digital neural networks to study whether the estimates of the age of the brain of identical twins are closer to each other than that of single fetuses. The data were taken from MRI scans, including images of the entire head, nose, ears, tongue, spinal cord, and, in some cases, fat around the neck. After minimal pre-processing, they were fed to the neural networks, which, after training, were able to give an estimate of the age of the brain. Confirming the hypothesis of the influence of genetics, the brain ages of identical twins differed less than those of single-fetuses.
And although these results suggest that the age of the brain is most likely determined by genetics, Cole warns that it is not necessary to reject the influence of the environment. "Even if you are genetically predisposed to the fact that your brain will look older," he said, "there is a chance that if you change the environment, it will help you to completely level the damage caused by genetics."
Help neural networks in assessing the age of the brain has its drawbacks - at least for today. Neural networks sift through MRI data and find differences between individuals, despite the fact that researchers are not yet sure which differences are relevant to the issue under study. But the general lack of deep learning is that no one knows what features of the data set the neural network recognizes. On the raw MRI images, the whole head is visible, so Cole admits that we may be talking about the whole age of the head, and not just the age of the brain. He said that he had already been told that the person’s nose was changing with time, so how to determine that the algorithm does not really track this feature?
But Cole is sure that this is not the case, since his neural network worked equally well with both raw data and images in which all features of the head outside the brain were removed. He expects that the real benefits of understanding what the neural network pays attention to can be obtained by understanding which parts of the brain most influence the age estimate.

Tobias Kaufman
Tobias Kaufman, a researcher at the Norwegian Center for Mental Diseases at the University of Oslo, suggested that machine learning technologies used to predict brain age are almost irrelevant if the model is properly trained and tuned. The results of different algorithms will usually be the same, as Cole discovered, comparing the results of the GPR algorithm with the neural network.
The difference, according to Kaufman, is that Cole’s in-depth training method eliminates the need for time-consuming and time-consuming preprocessing of MRI data. Reducing this step will sometime speed up the delivery of a diagnosis in clinics, but for now it protects scientists from the effect of prejudice on raw data.
Increasing data sets may allow for more complex predictions, for example, to determine patterns associated with mental health. Therefore, Kaufman said, having complete information in a data set, without transforming and reducing, can help science. "I think this is a huge advantage of the method of deep learning."
Kaufman is the lead author of the work, which is currently undergoing an expert assessment, which describes the largest study of the age of the brain from the photographs. Researchers used machine learning on structured MRI data to understand which parts of the brain show the most obvious signs of aging in people with mental illness. Then they took the next step, examining which genes determine the patterns of brain aging in healthy people. They were very interested in the fact that many of the genes that influenced the age of the brain were also involved in widespread violations of his work, which probably indicates the similarity of their biological paths of development.
He said that their next goal would be to go beyond the limits of heritability and the installation of certain nerve pathways and genes involved in determining the anatomy of the brain and its signaling networks.
And although Kaufman’s approach, like Cole’s, also focuses on anatomy, he stressed the importance of measuring the age of the brain in terms of its connections. “I think both of these approaches are very important,” he said. “We need to understand the inheritance and basic genetic architecture of both the structure and the functionality of the brain.”
Cole has no shortage of further research ideas. There is something exciting in that in order to understand our intellect, we have to use artificial intelligence, and this is evident from the way we reveal the links between genes, brain, behavior and heredity. Unless, of course, it turns out that all this time we have been studying the age of the noses.