
Labor Market Analysts and Data Scientists
Data Science is not a new field. They have been engaged in data processing for more than 50 years, which does not prevent the sphere from remaining at the peak of popularity: data analytics and Data Scientist are very popular among employers today. The editors of Netologiya decided to ask market experts - New.HR agency, which specializes in Data Science, and leading IT companies - about the real situation in the field of working with data.
How much do specialists of different levels get? How to increase your value in the eyes of the employer? Where are companies looking for employees? What do HR look at first when choosing a candidate?
The DS labor market is developing rapidly. In the last two years alone, we have trained more than 800 students in Data Science specialization, most of them work in specialized positions in Russian and foreign companies. In total, we have more than 10 training programs on the topic of Data Science, but the most popular areas are Data Analyst, BI Analyst, Data Scientist, and Machine Learning.
All students have different goals: someone comes to radically change their profession, someone - to grow in their position and start earning more. Salary, of course, is one of the main reasons why there are more and more people who want to study Data Science.
In June 2019, New.HR agency will release a large study of the market for analysts and Data Scientist, but for now, Oksana Prutyanova, head of analytics and Data Scientists at New.HR , agreed to share salary data in the field and give advice to specialists.
Data obtained through a direct survey of specialists in Data Science. Indicators received from respondents working in Moscow are taken into account. The salary figures are shown after taxes, “on hand”:
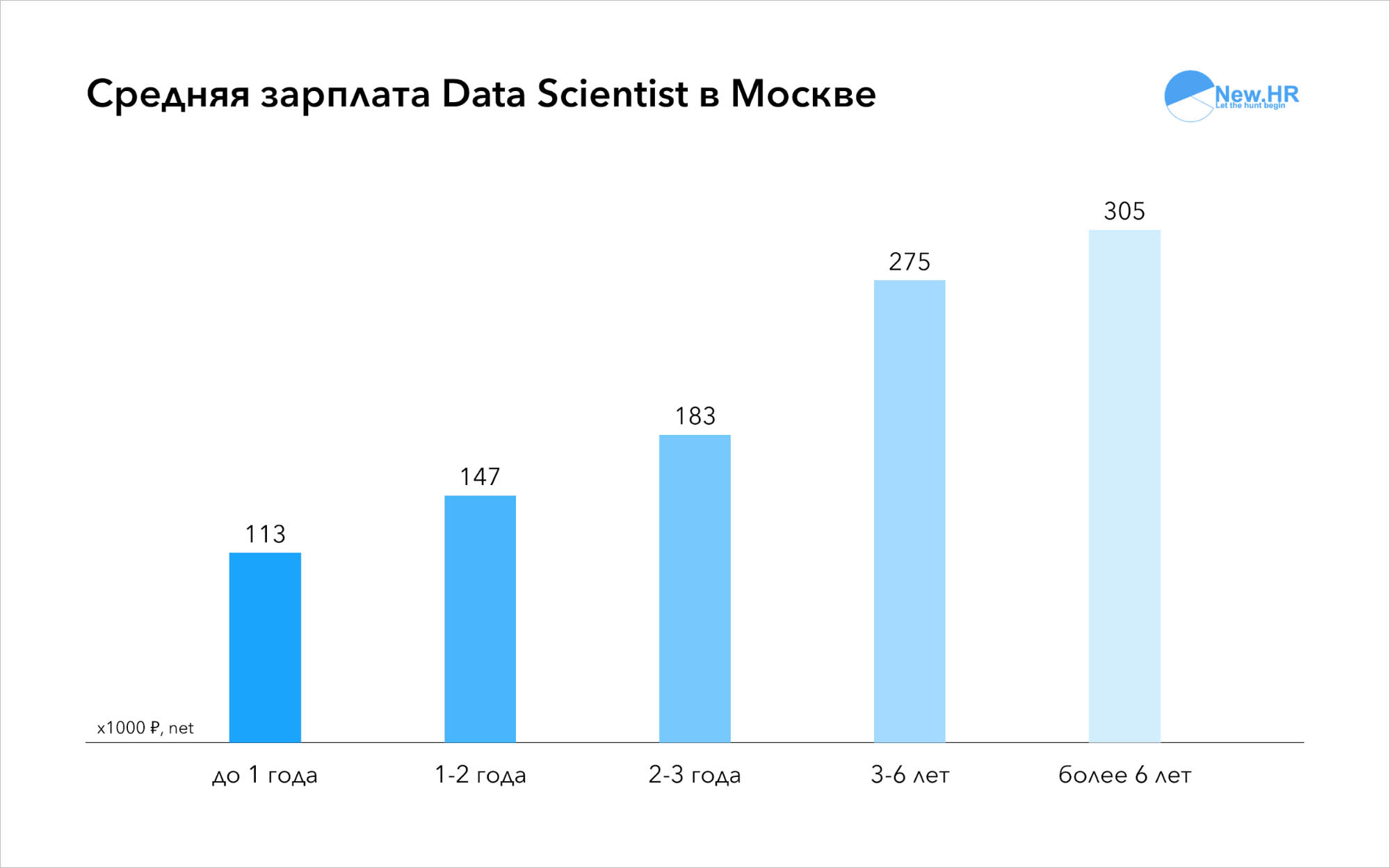
What does the Data Scientist income level consist of:
There are points that add value to Data Scientist in the eyes of the employer:
Total, you can select a list of tips for those data professionals who want to earn more. Most of them are quite universal and banal - but nevertheless, they work:
We talked with HR managers and managers of analytics in IT companies and found out where they usually look for employees to join their team, and also what they look at first of all when selecting candidates.
Alexandra Golovina, head of it selection at Avito :
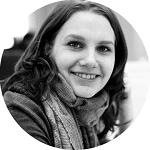
In Avito, Data Scientist is a separate position, different from a data analyst. Data analysts evaluate the quality of existing functionality or a product and help make decisions about changes in them: develop and implement metrics, test hypotheses, conduct A / B tests - identify the main bottlenecks, user pains and think over how to solve them.
Data Scientist is responsible for building ML models that help solve problems already found by data analysts: prepare data, write prototypes, etc.
Usually we get quite a lot of feedback on both positions, but, unfortunately, most resumes are irrelevant. For the most part, people did not work with algorithms, or did not own the stack we needed. Therefore, most often we are looking independently: by alternative sources (for example, competitions) and by internal recommendations.
When choosing an employee, we look at a set of factors: what tasks the candidate solved, whether he participated in any projects, how he wants to develop, etc. It is also important for us that the candidate has a fundamental physical and mathematical education. Our employees came from various fields and companies: from FMCG to specialized competing companies, but all have one thing in common: the presence of fundamental education. Work experience itself is also important, but the specifics of the company are less important.
Having a portfolio is not necessary for us, but it can greatly help a candidate during interviews.
For each unit, we have a prepared test task, which is as close as possible to the future tasks of the employee. This is a win-win situation for everyone: we understand the level of the candidate, and the candidate, in turn, will find out what exactly he will do.
But if a person has a portfolio and he is ready to send the source code of any of his projects in Python, which in his opinion shows a good level of language proficiency, and is ready to explain any part of the code, or is ready to provide a solution to a competition, the technical task is unnecessary.
Nikita Pestrov, Data Science Lead, Habidatum :
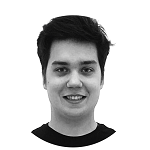
At Habidatum, we create urban data analysis products and customer projects. Projects require the collection of data, such as public transport routes or real estate listings, and an understanding of how to handle them. This, as well as the creation of reporting materials, the search for patterns and conclusions is usually done by a data analyst. Data Scientist develops models for these projects and implements part of them together with Data Engineer in our products and services. These two people work in conjunction.
Every year we conduct team internships to select new employees for analytics. For several weeks, young specialists from different fields have been conducting research, trying out the real problems that we face in the company. During this time, we manage to understand the strengths of a person and decide on the continuation of work with him.
We also conduct workshops and lectures on various topics, after which we receive resumes from interested specialists and are happy to review them. And of course the #jobs channel in the Open Data Science community - when we are actively looking for a person for a specific task.
For young innovative companies, the knowledge and desire of a new employee to develop in the field of the company (for us this is a city) is more important than his knowledge in a specific position. Therefore, when selecting candidates, we look for an interest in urban issues, teamwork experience and the ability to turn difficult conditions into simple models in a person’s resume. In a summary, this can be seen by participating in relevant conferences, internships or projects on topics of interest to us.
Vera Mashkova, Vice President, Human Resources, ABBYY Group of Companies :
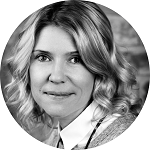
In different companies, the position Data Scientist may mean people with different tasks. We mainly employ universal employees who are involved in data analysis and 50/50 development. This is the need of our business.
There are Data Scientist positions in the company, including within the framework of a large internship program, but we do not translate them as a “data analyst”, we are talking more about “researchers” in two departments of promising research and development. We most often call such positions Junior Data Scientist (NLP).
The popularity of Data Science is growing. The market has become more developed, many universities have become more active in teaching data science. There are many additional courses. A growing number of candidates are reassigned.
The largest external recruitment site is HeadHunter. In addition, we are happy to employ children from our two departments at MIPT. There are good and structured resumes, but not all candidates are able to describe their experience. To help novice professionals with this, we hold career development meetings for students at the Higher School of Economics and the Moscow Institute of Physics and Technology.
We also have developed a referral program within the company: colleagues advise their friends as candidates for open positions - so we often get very good recommendations.
When choosing an employee, we first of all pay attention to basic education: which university and in what direction a person graduated or is graduating.
If the candidate has a non-core (for example, humanitarian) education, he is highly likely to be unable to cope with his tasks. The most important thing for us is our willingness to learn, our readiness for independent research, if we are talking specifically about promising research and development teams.
If a person is engaged in scientific activity, this, of course, is a plus. A portfolio is not necessary for us if a person comes to the position of a junior specialist.
Alexey Kuzmin, development director, head of DS-direction at DomKlik :
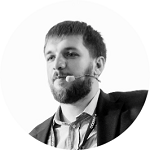
At DomKlik, we do not share the position of Data Scientist and data analytics. We clearly have another role highlighted - analyst / business analyst. Its main task is to build business reporting. Data Scientist is engaged in the construction of models and obtaining insights from data based on machine learning. The area of responsibility of the data analyst runs approximately in the middle, and therefore the tasks are divided approximately in half. Part is given to the stream of "business analytics", part - to the direction of Data Science.
In my opinion, the labor market in the field of Data Science is now very overheated - high demand with a small number of truly competent specialists. Therefore, when searching, we use either thematic communities and groups, or try to actively use internal recommendations.
When choosing a candidate, we first look at previous jobs and tasks. A portfolio is not necessary, but encouraged, as it helps to understand what tasks the applicant has solved before and with what experience he can strengthen our team. We are faced with a very wide range of tasks (from computer vision to word processing and sound signals) and we also need a variety of competencies.
We try to study all the CVs sent, the only taboo is when a person who responds to the position of Data Scientist does not have a single line in the CV with skills in Data Science. In this case, it is not very clear what a person is counting on, since even a specialist without experience should have undergone any training in the field.
Dmitry Malkov, project manager at Data Monsters , head of educational and scientific projects of the Laboratory of business solutions based on Artificial Intelligence MIPT :

In our company, the position of a Data Scientist requires knowledge of mathematics and Python, while a data analyst can have a liberal arts education. For example, we have a number of projects with professional historians on economic statistics. The analyst is required to work manually with data and a deeper immersion in the subject area.
We pay great attention to training personnel to replace ourselves from a young age: we conduct courses for high school students at physics and mathematics schools, and work with university students. It takes a long time to wait for the results, but they justify themselves - this is how truly “our” people are formed. As for the experienced dear employees, here we come carefully. Just placing a vacancy and selecting those who responded is not enough. Fortunately, there are many mature specialists among MIPT graduates.
We note that over the past few years, more and more people, not only physical technicians, are interested in a career at the intersection of science and business, and we have wide scope for such work and interesting international projects.
When selecting candidates, we try to take people according to personal recommendations.
Well, it’s good if, in addition to mathematics, a person has subject knowledge.
We are alarmed by candidates who jump from one company to another and work in obscure positions. For example, now the direction of machine learning is at the peak of popularity, but we are filtering out those who come into the profession because of hype. They did blockchain last year, AI is doing it. For science, nevertheless, many years of work and a corresponding outlook are needed.
Maxim Chikurov, an expert in Data Science in Netology and the head of the analytics team at a large Russian bank , believes that despite the developed labor market in the field of Data Science, it cannot be called very dynamic:
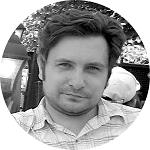
In the banking sector, in my opinion, Data Science is less relevant, than data analysis.
A feature of the sphere now is a large number of people who want to "enter the profession." Such job seekers require a creative approach, because a resume with the heading Data Scientist, but without relevant experience, looks uninteresting. I advise, for example, to create a video presentation of your research, this will greatly raise you among other applicants. On the other hand, employers, too, often still have high expectations from Data Science in general, and as a result from candidates. Therefore, a full supply-demand relationship does not come out.
Almost all experts noted the lack of competent specialists in the market and the fact that companies constantly have new requirements for candidates in the field of Data Science. Therefore, those who want to keep up with the pace of the market should invest as much as possible in developing themselves as a specialist: independently read training materials, study cases and articles of practitioners, participate in workshops, internships, and take courses. All this will allow to remain in demand and receive decent wages.
How much do specialists of different levels get? How to increase your value in the eyes of the employer? Where are companies looking for employees? What do HR look at first when choosing a candidate?
The DS labor market is developing rapidly. In the last two years alone, we have trained more than 800 students in Data Science specialization, most of them work in specialized positions in Russian and foreign companies. In total, we have more than 10 training programs on the topic of Data Science, but the most popular areas are Data Analyst, BI Analyst, Data Scientist, and Machine Learning.
All students have different goals: someone comes to radically change their profession, someone - to grow in their position and start earning more. Salary, of course, is one of the main reasons why there are more and more people who want to study Data Science.
How much do they pay for Data Scientist positions
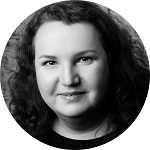
Data obtained through a direct survey of specialists in Data Science. Indicators received from respondents working in Moscow are taken into account. The salary figures are shown after taxes, “on hand”:
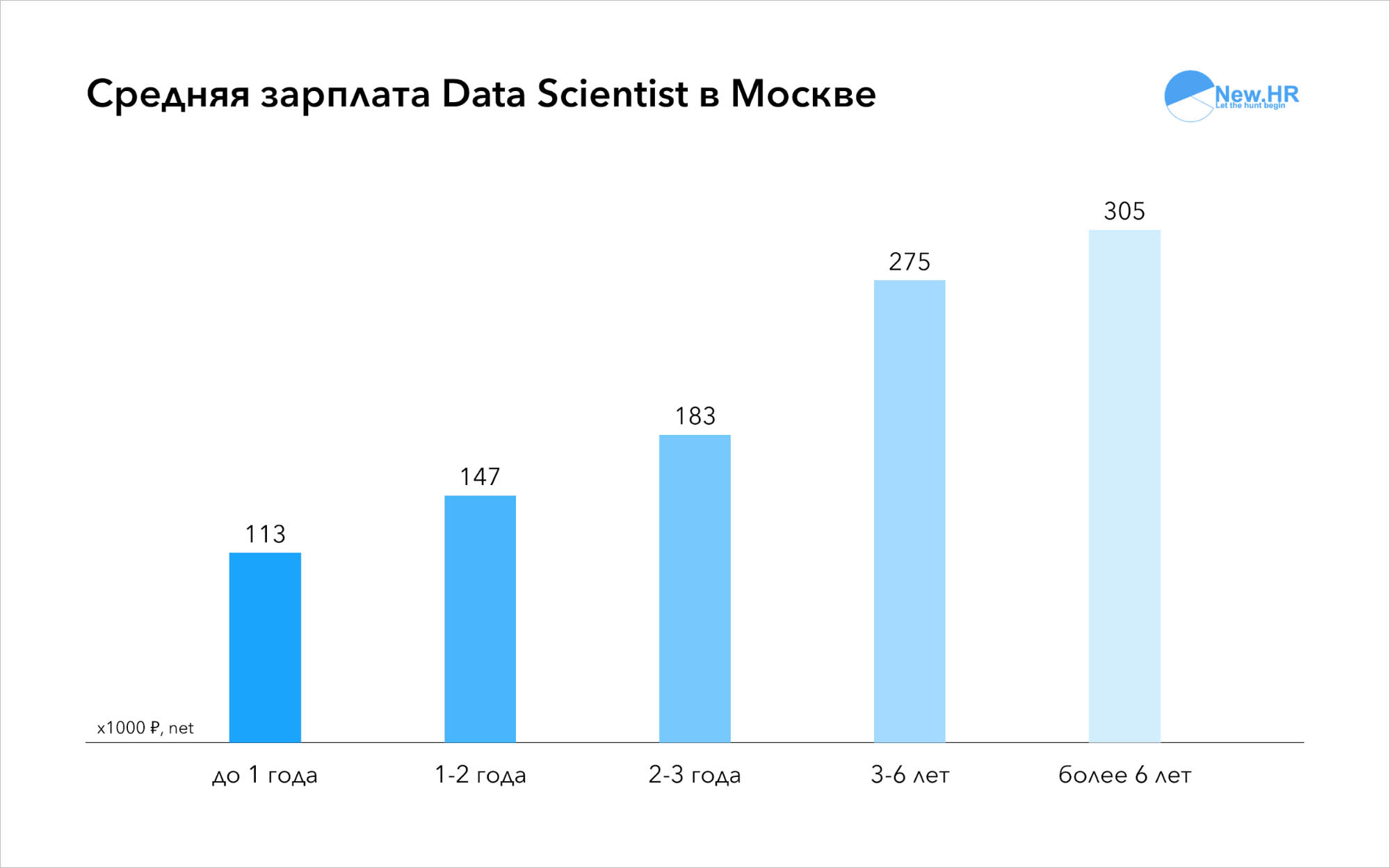
What does the Data Scientist income level consist of:
- general work experience in the specialty;
- depth of professional expertise;
- education in a status and quoted university. For example, strong analysts are trained at MIPT, MSU, ITMO;
- location - in Moscow there is more money. But even in the regions you can earn comparable money. For example, in cities with a strong academic base, such as Novosibirsk. Also, the capital level of salary can be counted on while working on a project remotely;
- knowledge of English greatly expands opportunities and allows you to be a member of the global professional community. Follow publications, study under the programs of the best world universities, consider vacancies abroad, write articles in English.
How Data Scientist to Increase Its Value in the Labor Market
There are points that add value to Data Scientist in the eyes of the employer:
- Knowledge of unique technology. When a specialist becomes an expert in a narrow professional field, for example, he is cool in NLP - this increases his value in the market.
- Work experience in a developed, hype field: everything related to computer vision, robotics, drones and so on. The cost of a specialist depends on the intersection of market demand, general trends and knowledge of a specific, narrow segment.
- Versatile experience. Some employers consider specialists only from their field, for example, from banks. But those who look wider and invite analysts from other areas benefit. Because the candidate can bring a new vision, apply non-obvious tools and approaches.
- The experience of creating your own startup. For the employer, this means that the specialist can understand the tasks at the business level.
- Participation in international projects. You can get a grant or compete at Kaggle .
- Experience in foreign companies. Foreign companies have adopted other standards and approaches to business, and this is appreciated by Russian companies.
Total, you can select a list of tips for those data professionals who want to earn more. Most of them are quite universal and banal - but nevertheless, they work:
- Constantly learn and precisely develop your expertise. To do this, it is important to monitor market trends in order to pump demand competencies.
- Be able to speak the language of business. To be proactive, understand and communicate the benefits of their work to leaders.
- Form a team around you. You can become the leader or team leader of a small team or training project. Management experience at any level is in demand.
- The Data Scientist profession is very much in demand in the market. Therefore, there is a lazy way - just go from company to company. So you can increase income by 20-30%. But this can only be done to a certain level.
What employers think about Data Scientist
We talked with HR managers and managers of analytics in IT companies and found out where they usually look for employees to join their team, and also what they look at first of all when selecting candidates.
Alexandra Golovina, head of it selection at Avito :
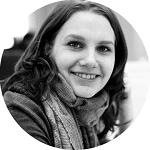
In Avito, Data Scientist is a separate position, different from a data analyst. Data analysts evaluate the quality of existing functionality or a product and help make decisions about changes in them: develop and implement metrics, test hypotheses, conduct A / B tests - identify the main bottlenecks, user pains and think over how to solve them.
Data Scientist is responsible for building ML models that help solve problems already found by data analysts: prepare data, write prototypes, etc.
Usually we get quite a lot of feedback on both positions, but, unfortunately, most resumes are irrelevant. For the most part, people did not work with algorithms, or did not own the stack we needed. Therefore, most often we are looking independently: by alternative sources (for example, competitions) and by internal recommendations.
When choosing an employee, we look at a set of factors: what tasks the candidate solved, whether he participated in any projects, how he wants to develop, etc. It is also important for us that the candidate has a fundamental physical and mathematical education. Our employees came from various fields and companies: from FMCG to specialized competing companies, but all have one thing in common: the presence of fundamental education. Work experience itself is also important, but the specifics of the company are less important.
Having a portfolio is not necessary for us, but it can greatly help a candidate during interviews.
For each unit, we have a prepared test task, which is as close as possible to the future tasks of the employee. This is a win-win situation for everyone: we understand the level of the candidate, and the candidate, in turn, will find out what exactly he will do.
But if a person has a portfolio and he is ready to send the source code of any of his projects in Python, which in his opinion shows a good level of language proficiency, and is ready to explain any part of the code, or is ready to provide a solution to a competition, the technical task is unnecessary.
Nikita Pestrov, Data Science Lead, Habidatum :
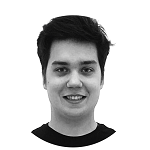
At Habidatum, we create urban data analysis products and customer projects. Projects require the collection of data, such as public transport routes or real estate listings, and an understanding of how to handle them. This, as well as the creation of reporting materials, the search for patterns and conclusions is usually done by a data analyst. Data Scientist develops models for these projects and implements part of them together with Data Engineer in our products and services. These two people work in conjunction.
Every year we conduct team internships to select new employees for analytics. For several weeks, young specialists from different fields have been conducting research, trying out the real problems that we face in the company. During this time, we manage to understand the strengths of a person and decide on the continuation of work with him.
We never took an analyst or Data Scientist without first working with a candidate as part of an internship.
We also conduct workshops and lectures on various topics, after which we receive resumes from interested specialists and are happy to review them. And of course the #jobs channel in the Open Data Science community - when we are actively looking for a person for a specific task.
For young innovative companies, the knowledge and desire of a new employee to develop in the field of the company (for us this is a city) is more important than his knowledge in a specific position. Therefore, when selecting candidates, we look for an interest in urban issues, teamwork experience and the ability to turn difficult conditions into simple models in a person’s resume. In a summary, this can be seen by participating in relevant conferences, internships or projects on topics of interest to us.
Vera Mashkova, Vice President, Human Resources, ABBYY Group of Companies :
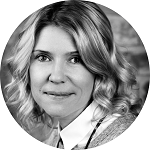
In different companies, the position Data Scientist may mean people with different tasks. We mainly employ universal employees who are involved in data analysis and 50/50 development. This is the need of our business.
There are Data Scientist positions in the company, including within the framework of a large internship program, but we do not translate them as a “data analyst”, we are talking more about “researchers” in two departments of promising research and development. We most often call such positions Junior Data Scientist (NLP).
The popularity of Data Science is growing. The market has become more developed, many universities have become more active in teaching data science. There are many additional courses. A growing number of candidates are reassigned.
The largest external recruitment site is HeadHunter. In addition, we are happy to employ children from our two departments at MIPT. There are good and structured resumes, but not all candidates are able to describe their experience. To help novice professionals with this, we hold career development meetings for students at the Higher School of Economics and the Moscow Institute of Physics and Technology.
We also have developed a referral program within the company: colleagues advise their friends as candidates for open positions - so we often get very good recommendations.
When choosing an employee, we first of all pay attention to basic education: which university and in what direction a person graduated or is graduating.
If the candidate has a non-core (for example, humanitarian) education, he is highly likely to be unable to cope with his tasks. The most important thing for us is our willingness to learn, our readiness for independent research, if we are talking specifically about promising research and development teams.
It is important to be prepared to bring in new ideas.
If a person is engaged in scientific activity, this, of course, is a plus. A portfolio is not necessary for us if a person comes to the position of a junior specialist.
Alexey Kuzmin, development director, head of DS-direction at DomKlik :
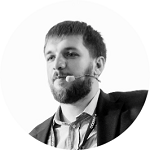
At DomKlik, we do not share the position of Data Scientist and data analytics. We clearly have another role highlighted - analyst / business analyst. Its main task is to build business reporting. Data Scientist is engaged in the construction of models and obtaining insights from data based on machine learning. The area of responsibility of the data analyst runs approximately in the middle, and therefore the tasks are divided approximately in half. Part is given to the stream of "business analytics", part - to the direction of Data Science.
In my opinion, the labor market in the field of Data Science is now very overheated - high demand with a small number of truly competent specialists. Therefore, when searching, we use either thematic communities and groups, or try to actively use internal recommendations.
When choosing a candidate, we first look at previous jobs and tasks. A portfolio is not necessary, but encouraged, as it helps to understand what tasks the applicant has solved before and with what experience he can strengthen our team. We are faced with a very wide range of tasks (from computer vision to word processing and sound signals) and we also need a variety of competencies.
We try to study all the CVs sent, the only taboo is when a person who responds to the position of Data Scientist does not have a single line in the CV with skills in Data Science. In this case, it is not very clear what a person is counting on, since even a specialist without experience should have undergone any training in the field.
Dmitry Malkov, project manager at Data Monsters , head of educational and scientific projects of the Laboratory of business solutions based on Artificial Intelligence MIPT :

In our company, the position of a Data Scientist requires knowledge of mathematics and Python, while a data analyst can have a liberal arts education. For example, we have a number of projects with professional historians on economic statistics. The analyst is required to work manually with data and a deeper immersion in the subject area.
We pay great attention to training personnel to replace ourselves from a young age: we conduct courses for high school students at physics and mathematics schools, and work with university students. It takes a long time to wait for the results, but they justify themselves - this is how truly “our” people are formed. As for the experienced dear employees, here we come carefully. Just placing a vacancy and selecting those who responded is not enough. Fortunately, there are many mature specialists among MIPT graduates.
We note that over the past few years, more and more people, not only physical technicians, are interested in a career at the intersection of science and business, and we have wide scope for such work and interesting international projects.
When selecting candidates, we try to take people according to personal recommendations.
We draw attention to previous work experience and the person’s closeness to us in spirit.
Well, it’s good if, in addition to mathematics, a person has subject knowledge.
We are alarmed by candidates who jump from one company to another and work in obscure positions. For example, now the direction of machine learning is at the peak of popularity, but we are filtering out those who come into the profession because of hype. They did blockchain last year, AI is doing it. For science, nevertheless, many years of work and a corresponding outlook are needed.
Maxim Chikurov, an expert in Data Science in Netology and the head of the analytics team at a large Russian bank , believes that despite the developed labor market in the field of Data Science, it cannot be called very dynamic:
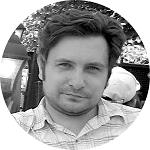
In the banking sector, in my opinion, Data Science is less relevant, than data analysis.
A feature of the sphere now is a large number of people who want to "enter the profession." Such job seekers require a creative approach, because a resume with the heading Data Scientist, but without relevant experience, looks uninteresting. I advise, for example, to create a video presentation of your research, this will greatly raise you among other applicants. On the other hand, employers, too, often still have high expectations from Data Science in general, and as a result from candidates. Therefore, a full supply-demand relationship does not come out.
Almost all experts noted the lack of competent specialists in the market and the fact that companies constantly have new requirements for candidates in the field of Data Science. Therefore, those who want to keep up with the pace of the market should invest as much as possible in developing themselves as a specialist: independently read training materials, study cases and articles of practitioners, participate in workshops, internships, and take courses. All this will allow to remain in demand and receive decent wages.
From the editors
- profession " Data Scientist "
- profession “ Data Analyst ”
- online course « the Python for working with data "
- online course " the Power the BI & Excel the PRO "
is also waiting for all who are interested in the scope of Data Science mitape on " Career in Data Science for Beginners " on August 11 in Moscow (to be broadcast)