
Does AlphaGo have a chance in a match against Lee Sedol: opinions and ratings of professional players in th
Google’s go-pro 9th match and Google’s AI will take place in March
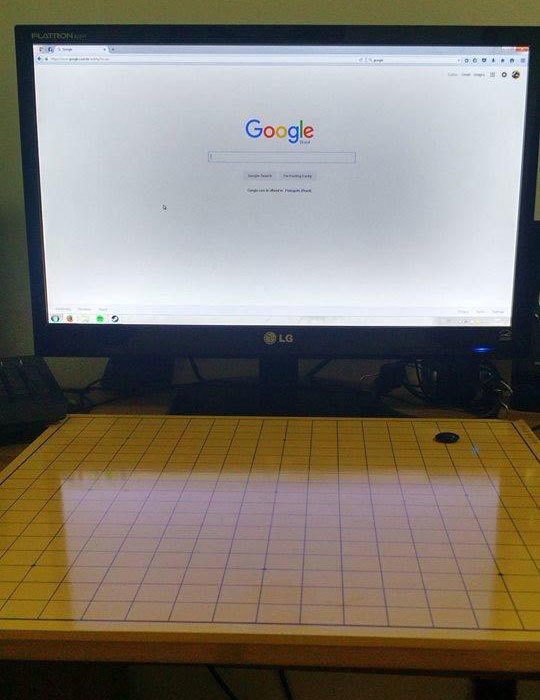
Nevertheless, professional players and acquaintances from go had questions about the quality of the game. Hui is a three-time champion, but he is a European champion, where the level of the game is not too high. Not only the player's choice to demonstrate the power of AlphaGo, but also some moves in the parties raises questions.
Algorithm
Guo has long been considered a game to train in which artificial intelligence is difficult due to the huge search space and the complexity of the choice of moves. Guo belongs to the class of games with perfect information, that is, players are aware of all the moves that other players have previously made. The solution to the problem of finding the outcome of the game involves computing the optimal value function in the search tree containing approximately b d possible moves. Here b is the number of correct moves in each position, and d is the length of the game. For chess, these values are b ≈ 35 and d ≈ 80, and a complete search is not possible. Therefore, the positions of the figures are evaluated, and then the assessment is taken into account in the search. In 1996, the computer won chess for the first time against the champion, and since 2005, no champion has been able to beat the computer.
For go b ≈ 250, d ≈ 150. The possible positions of stones on a standard board are more than googol (10 100 ) times more than in chess. The number of possible positions is greater than the atoms in the universe. Complicating the situation is that it is difficult to predict the value of states because of the complexity of the game. Two players place stones of two colors on a board of a certain size, the standard field is 19 × 19 lines. The rules vary in details, but the main goal of the game is simple: you need to fence off a territory larger than the opponent’s size on the board with stones of your color.
Existing programs can play go at the amateur level. They use the Monte Carlo tree search to evaluate the value of each state in the search tree. The programs also include policies that predict the moves of strong players.
Recently, deep convolutional neural networks have been able to achieve good results in face recognition and image classification. At Google, AI even learned to play 49 old Atari games on its own . In AlphaGo, similar neural networks interpret the position of stones on the board, which helps to evaluate and select moves. At Google, researchers took the following approach: they used value networks and policy networks. Then these deep neural networks are trained both on a set of parties of people, and on a game against their copies. New is also the search, combining the Monte Carlo method with networks of politics and value. Neural network training scheme and architecture.

Neural networks were trained in several stages of machine learning. At first, controlled training of the policy network was carried out directly using the moves of human players. Another policy network has been reinforced learning. The second played with the first and optimized it so that the policy shifted to win, and not just predictions of moves. Finally, training was conducted, reinforced by a network of values that predicts the winner of games played by policy networks. The end result is AlphaGo, a combination of the Monte Carlo method and networks of politics and value. The result of correct prediction of the next move was achieved in 57% of cases. Prior to AlphaGo, the best result was 44% .
160 thousand games with 29.4 million positions from the KGS server were used as input for training. The parties of players from the sixth to the ninth dan were taken. A million positions were allocated for tests, and the actual training was conducted on 28.4 million positions. The strength and accuracy of networking policies and values. For the algorithms to work, they need several orders of magnitude greater computing power than with the traditional search. AlphaGo is an asynchronous multi-threaded program that performs simulation on the cores of a central processor and runs networks of policies and values on video chips. The final version looked like a 40-threaded application running on 48 processors (probably it meant separate cores or even hyper-threading) and 8 graphics accelerators. A distributed version of AlphaGo was also created, which uses several machines, 40 search threads, 1202 cores and 176 video accelerators.
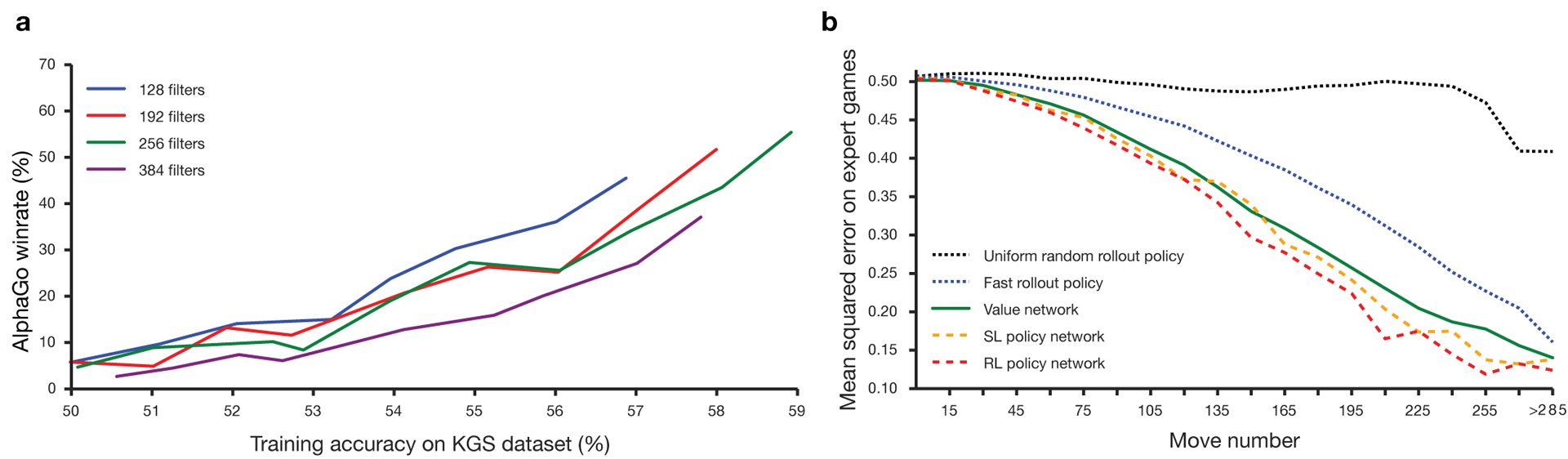
The full DeepMind report is available in the document . Search for Monte Carlo in AlphaGo. To assess AlphaGo's abilities, internal matches were held against other versions of the program, as well as other similar products. Including a comparison was conducted with such popular commercial programs as Crazy Stone and Zen, and the strongest open source projects Pachi and Fuego. All of them are based on high-performance Monte Carlo algorithms. But also AlphaGo compared with non-Monte Carlo GnuGo. Programs were given 5 seconds per move. A comparison was made of both the AlphaGo running on a single machine and the distributed version of the algorithm.

According to the developers, the results showed that AlphaGo is much stronger than any previous go-programs. AlphaGo won 494 of 495 games, i.e. 99.8% of matches against other similar products. Go rules allow a handicap , handicap: up to 9 black stones can be set on the field before white moves. But even with 4 handicap stones, the AlphaGo single-machine won 77%, 86% and 99% of the time against Crazy Stone, Zen and Pachi, respectively. The distributed version of AlphaGo was significantly stronger: in 77% of games, it defeated the single-machine version and in 100% of games - all other programs. AlphaGo vs other programs.
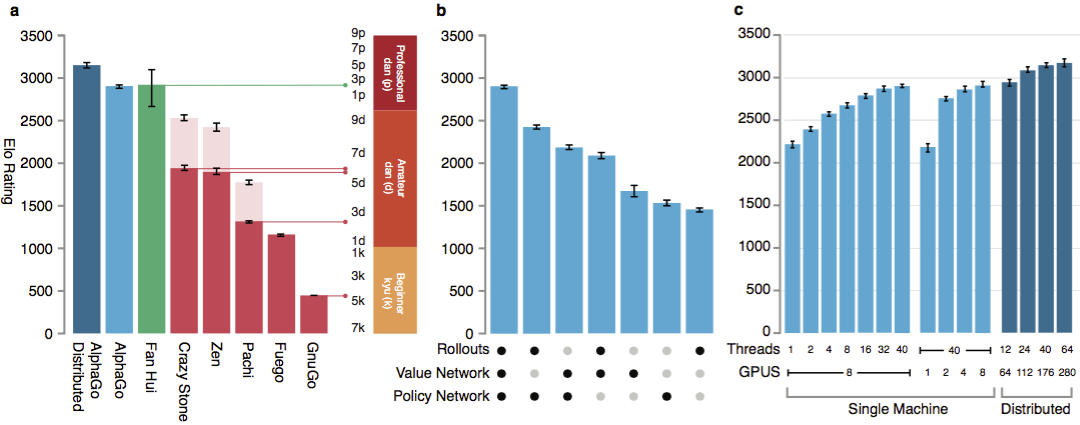
Finally, the created product was compared with a person. The professional player 2 dan, the winner of the European Go Championship in 2013, 2014 and 2015, Fan Hui, fought against the distributed version of AlphaGo. The games were held with the participation of a judge from the British Federation of go and the editor of the journal Nature. 5 games were held in the period from October 5 to 9, 2015. All of them won the Google DeepMind development algorithm. It was these games that led to the statement that the computer was the first to be able to beat a professional player in go. In addition to 5 official parties, 5 unofficial parties were held, which did not count. Fan won two of them.
Available recording moves five games , viewing in a web widget , and videos on YouTube .
Criticism from professional players
The choice of a professional player and the weak game of the champion are being questioned. The chosen rules are also unclear: an hour per game instead of several hours of serious games. However, the format was chosen by Hui himself. In March, AlphaGo will play against Lee Sedola. Can the algorithm beat the Korean professional of the ninth dan, considered one of the best players in the world? At stake is a million dollars. If a person wins, Li Sedol will receive it; if the algorithm wins, he will go to charity.
Researchers say that during the October battle with humans, the AlphaGo system considered thousands of times less positions than Deep Blue during a historic match with Kasparov. Instead, the program used a network of policies for smarter choices and a network of values to more accurately evaluate positions. Perhaps this approach is closer to how people play, the researchers say. In addition, the Deep Blue grading system was programmed manually, while the AlphaGo neural networks were trained directly from the games using universal algorithms of supervised learning and reinforcement learning. Lee Sedoll will try his hand against AlphaGo in March.

Professional players have different points of view. It seems to some that Google specifically chose not a very strong player, someone is sure that Sedol will lose this March.
One of the strongest English-speaking professional players in go, Kim Mengwang (ninth dan) believes that Fan Hui did not play at full strength. At the 51st minute of the video, he gives a concrete example from the second installment. Perhaps Fan played both with a weaker one to test the power of the computer, Kim says. Mengwan admitted that AlphaGo is a shockingly powerful program, but it is unlikely to defeat Lee Sedol.
Match referee Toby Manning told the British Go Journal about the match. He analyzed all five games and highlighted some points. AlphaGo made mistakes in the second, third and fourth games, but Fan did not use them. Three-time European champion replied with his own. An article in the journal ends with a general positive assessment by AlphaGo: the program is strong, but it is unclear how much.
Also, when preparing the material, I received comments from Russian professionals and go lovers. Alexander Dinerstein (Kazan), third dan (professional), seven-time European champion:
In matches with Kasparov, a whole group of strong grandmasters worked on the debut of the Deep Blue program. It is possible that such a team will be assembled for the match with Li Sedol, but the match with Fan shows that work in this direction was not carried out in principle. The strongest go player in the Google team plays by virtue of the fifth amateur dan. This is the level of four handicap stones with Lee Sedoll.
In all games with Fan, the program played at point 4-4 (Hoshi in Japanese, starpoint in English). Besides her, there are many other possible beginnings. Of the common ones: 3-3, 3-4, 5-3, plus there are various non-standard openings, where the first move is played, for example, in the center of the board. From the point of view of the debut novelties, the program did not show anything new. In this regard, she played very primitively.
The debuts that Fan used were simply packed into her base. He did not use anything non-standard. For me, this remains a big question - how will the program work if we turn off the opening directories from the very first moves. There are so many options on an empty board that no Monte Carlo method can count them. In this go compares favorably with chess. In chess, everything has long been studied to a depth of 20-30 moves, and in go, if desired, the first move can create a position that has not been encountered in the history of professional go. The program will have to play independently, and not pull out options from the knowledge base. Let's see if she can do it. I strongly doubt it and put Lee Sedola.
By the way, in the summer of 2016, the European Go Championship ( EGC), in the framework of which a computer program tournament always takes place. The Russian Federation of Go invited all the strongest programs to participate in the tournament. If they accept the invitation, then perhaps it is at this tournament for the first time that Google and Facebook programs will play among themselves. The latter, unlike its competitor, is following an honest path. The DarkForest bot plays thousands of games on the KGS server . The strongest version is approaching the sixth dan on the server. This is a very good level. Fan Hui and players of his level - this is about the eighth dan on the server (out of nine possible). The difference is about two stone handicaps. With such a difference, a program can sometimes really beat a person. If on equal terms, then approximately in one batch of ten.
Maxim Podolyak, (St. Petersburg), Vice President of the Russian Federation of Go:
It’s hard for me to believe that the largest software developer is starting to solve the most difficult, and generally speaking insoluble, task, does not illuminate his work, does not show intermediate results, successful or not, and immediately achieves tremendous success. Rather, I believe that Google has developed an excellent advertising move: I did not find the most powerful player with professional status and a champion title, which, under certain conditions, would agree not to play very convincingly. This is very similar to the world that I know. A simple and understandable solution: no risks, no prosecution for fraud, no consequences, only a relatively small fee to a living participant. And the advantages are so great that it is hard to imagine: the main competitor is knocked out, the whole world is booming about tremendous success and an unprecedented breakthrough. Google can do anything. Even if the deception later reveals itself, no one will notice this. And who will open it? Who will check the quality of the test?
Alexander Krainov (Moscow), lover of the game go:
Due to my professional activity, I know the situation “from the other side” quite well.
In 2012, there was a quantum leap in machine learning in general. The amount of data for training, the level of algorithms and the power for training have reached such a level that artificial neural networks (developed as a principle for a long time) began to give fantastic results.
The fundamental difference between training on neural networks is that they do not need to be given input factors (in the case of go, explain, for example, which forms are good). In the limit, even the rules can not be explained to them. The main thing is to give a large number of positive (moves of the winning side) and negative (moves of the losing side) examples. And the network will learn itself.
As soon as the algorithm reaches a high level of the game, the program can play with itself a huge number of games, learning from the result. And it’s not necessary to finish the game. You can do this: any position is taken, the network predicts several good moves, the moves are calculated (using traditional algorithms for searching through the decision tree) as much as possible, the result is added to the training set.
I would not recommend betting on Sedol, focusing on playing the program with Fan.
Roughly speaking, observing the game of the program, we are watching the game of a talented four-year-old child. By the time of the game with Sedola in March, he can greatly add. Neural network models now learn very quickly with enough data. And in the case of go, there is a lot of data. Plus, as I said before, they can be generated.
What Lee Sedol himself says
Professional go players compete not for the world title, but for titles. The recognition and status of the master is determined by the number of titles that he was able to get during the year. Lee Sedol is one of the five strongest go players in the world, and in March of this year he will have to fight with the AlphaGo system.
The Korean champion himself predicts that he will win with a score of 4-1 or 5-0. But after 2-3 years, Google will want to take revenge, and then the game with the updated version of AlphaGo will be more interesting, says Lee.
The task of creating such an algorithm poses new questions about what learning and thinking are. As M. Emelyanov reminds , the third level of mastery (pin) from above, according to the ancient Chinese classification, is called "complete clarity." This level of play suggests that decisions are made intuitively, with little or no options. One of the strongest masters of the 20th century, Guo Seigen, said that it seemed to him that he would have won against the “go-god” by taking two or three stones of handicap. Seigan believed that he had almost reached the limit of understanding the game. Can a neural network achieve this? Perhaps human intuition is an algorithm laid down by nature?
The author thanks Alexander Dinerstein and the public go_secrets for comments and help in the publication.