Amazon Cloud Services and Investment Portfolio Analysis
Recently, high volatility has been observed in stock markets, when, for example, a stable paper of a well-known company can lose several percent at once on news of sanctions against its leadership or, on the contrary, fly to the skies on a positive report and investors' expectations about super-profit dividends.
How to determine whether the ownership of this security brought in income or only loss and disappointment?
(Source)
In this article I will tell you how to determine and visualize the adjusted financial result for securities.
Using the example of client reporting Opening Broker, we consider parsing and consolidating brokerage reports for the stock market, building a cloud reporting system architecture followed by a simple and convenient analysis in AWS Quicksight.
Many trainings and training lessons tell us about the need to maintain a trading journal, which records all the parameters of the transaction for further analysis and summarizing the work of the trading strategy. I agree that such an approach to work on the Exchange allows you to discipline a trader, to increase his awareness, but it can also be great to tire out the tedious process.
I admit, at first I tried carefully to follow the advice of journaling, meticulously wrote down every transaction with its parameters in an Excel spreadsheet, built some reports, summary diagrams, planned future deals, but ... I was quickly tired of all this.
Often, brokerage companies are high-tech organizations that provide their clients with fairly high-quality analytics on virtually all issues of interest. It is fair to say that with each update this reporting gets better and better, but even the most advanced of them may not have the customization and consolidation that demanding and inquisitive customers want to see.
For example, Opening Broker allows you to receive brokerage reports in XML format in your account, but if you have an IIS and a normal brokerage account on the Moscow Stock Exchange (MOEX), these will be two different reports, and if you still have an account on St. Petersburg Petersburg Stock Exchange (SPB), then one more will be added to the first two.
So, to get a consolidated investor log, you will need to process three files in XML format.
The above reports on MOEX and SPB are slightly different in their formats, which will need to be taken into account in the implementation of the data mapping.
The diagram below shows the architecture model of the system being developed:
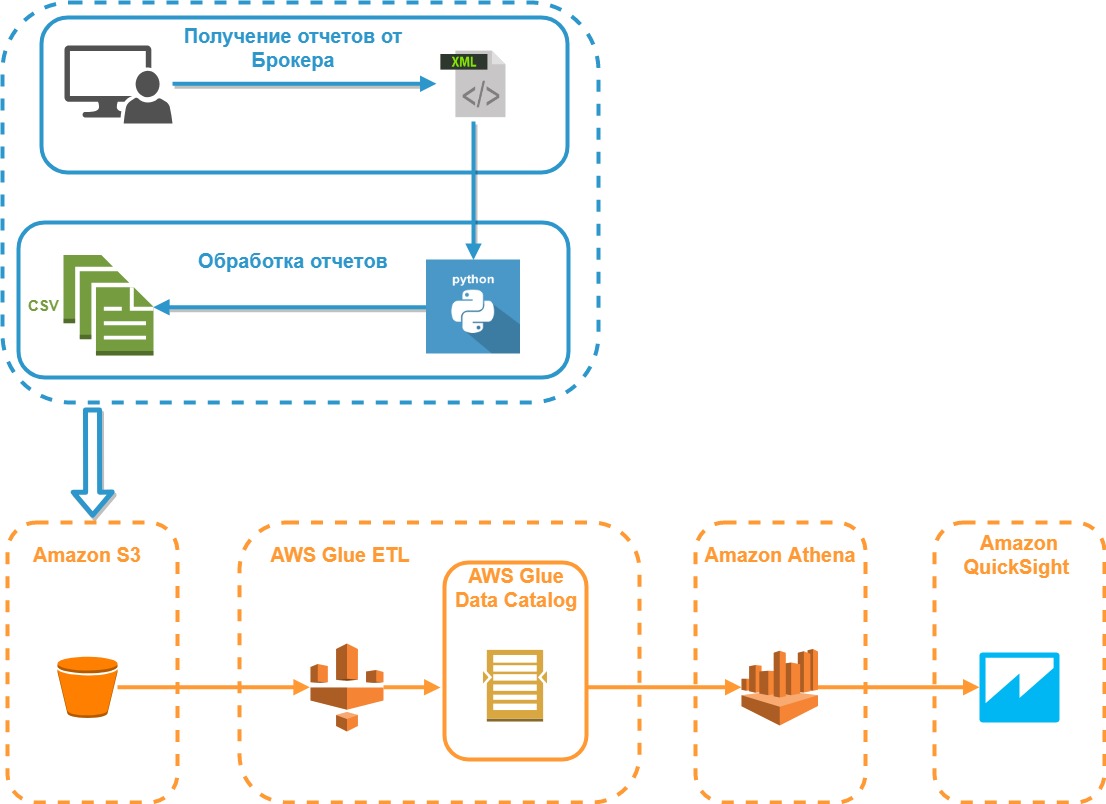
We will receive reports on all three accounts in the My Account for the maximum possible period (can be divided into several reports for each year), save them in XML format and put them in one folder. As a test data for the study we will use the fictional client portfolio, but with the most approximate parameters to market realities.

Suppose that the investor Mr. X we are considering has a small portfolio of five securities:
According to our five papers, there may be buy / sell transactions, dividends and coupon payments, prices may change, etc. We want to see the current situation, namely: the financial result, taking into account all payments, transactions and current market value.
And here comes Python, we read information from all reports into one array:
A few words about what these dictionaries are.
We process an array of XML documents and fill these dictionaries with the appropriate data:
All processing is looped through all the XML data from the reports. Information about the trading platform, client code is the same in all reports, so you can safely extract it from the same tags without using mapping.
But then you have to apply a special construction that will provide the necessary alias for the tag based on the report (SPB or MOEX), since the same data in these reports are called differently.
The get_allias function returns the name of the required tag for processing, taking as input the name of the trading platform:
The function get_briefcase is responsible for processing information about the state of the client portfolio:
Further, using the function get_deals, information about transactions is extracted:
In addition to processing an array with information about the parameters of the transaction, it also calculates the average price of an open position and the PNL realized by the FIFO method. The PnlSnapshot class is responsible for this calculation, for creating which, with minor modifications, the code presented here was taken as the basis: P & L calculation
And, finally, the most difficult to implement is the function of obtaining information about non-trading operations - get_nontrade_operation . Its complexity lies in the fact that in the report block used for non-trading operations, there is no clear information about the type of operation and the security to which this operation is linked.
Accordingly, it will be difficult to manage without regular expressions, therefore we will use them to the full. The other side of the question is that the name of the company does not always coincide with the name in the portfolio or in the transactions. Therefore, the received name of the issuer from the purpose of the payment must be additionally correlated with the dictionary. As a dictionary, we will use an array of transactions, since There is the most comprehensive list of companies.
The function get_company_from_str extracts the name of the issuer from the comment:
The function get_company_from_briefcase brings the name of the company to the dictionary, if it finds a match among the companies that participated in the transactions:
And finally, the final data collection function for non-trading operations is get_nontrade_operation :
The result of the collection of data from the reports will be three DataFrame, which are approximately the following:
So, all we have to do is perform the external merging of the table of transactions with the table of information about the portfolio:
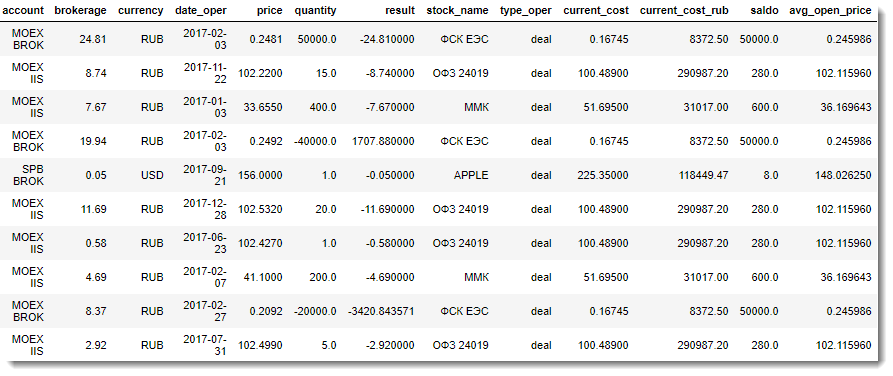
And, finally, the final part of the data array processing is the merging of the data array obtained at the previous step with the DataFrame for non-trade transactions.
The result of the work done is one large flat table with all the necessary information for the analysis:
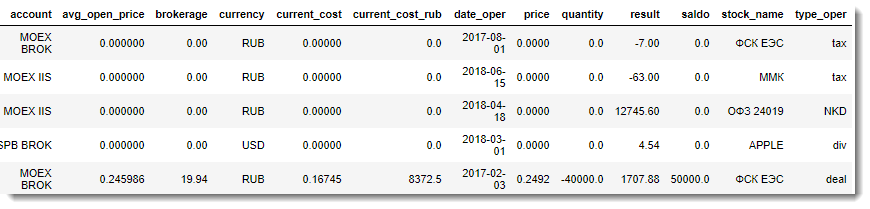
The resulting data set (Final Report) from the DataFrame is easily downloaded to CSV and can then be used for detailed analysis in any BI system.
Progress does not stand still, and now cloud services and serverless computing models are gaining great popularity in processing and storing data. This is largely due to the simplicity and low cost of such an approach, when building an architecture for complex calculations or processing large data does not require buying expensive equipment, and you only rent power for the cloud you need and deploy the necessary resources quickly for a relatively small fee. .
One of the largest and most well-known cloud technology vendors on the market is Amazon. Consider the example of the environment Amazon Web Services (AWS) building an analytical system for processing data on our investment portfolio.
AWS has an extensive selection of tools, but we will use the following:
With Amazon's documentation, everything is in order, in particular, there is a good article Best Practices When Using Athens with AWS Glue , which describes how to create and use tables and data using AWS Glue. Let us and we will use the main ideas of this article and apply them to create our own architecture of the analytical reporting system.
CSV files prepared by our report parser will be added to the S3 bucket. It is planned that the corresponding folder on S3 will be replenished every Saturday - at the end of the trading week, so we can not do without data partitioning by the date of the formation and processing of the report.
In addition to optimizing the work of SQL queries to such data, this approach will allow us to conduct additional analysis, for example, to obtain the dynamics of changes in the financial result for each paper, etc.
Consider the generated table in more detail.
If you click on the name of the created table, we will go to the page with the description of the metadata. At the bottom there is a table diagram and the most recent is a column that was not in the original CSV file - date_report. Glue creates this AWS column automatically based on the definition of the source data sections (in the S3 batch, we specifically named folders - date_report = YYYY-MM-DD, which allowed them to be used as sections, separated by date).
Having at our disposal downloaded processed data, we can easily proceed to their analysis. First, let's look at the features of Amazon Athena as the easiest and fastest way to perform analytical queries. To do this, go to the Amazon Athena service, select the database we need (financial) and write the following SQL code:
This query will give us a net financial result for each paper for all reporting dates. Because we have downloaded the same report three times for different dates, and the result will not change, which, of course, in the real market conditions will be different:
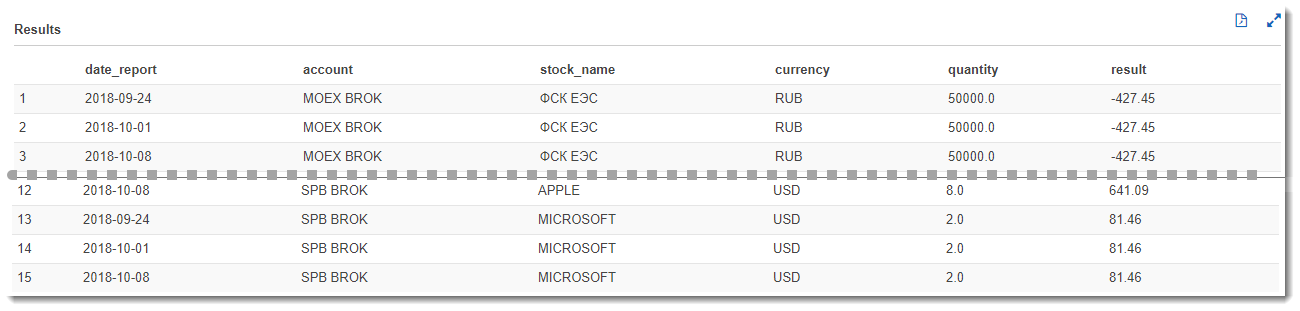
But what if we want to visualize the data in the form of flexible tables or charts? This is where Amazon QuickSight comes to the rescue, with which you can set up flexible analytics almost as quickly as writing a SQL query. Let's go to Amazon QuickSight (if you have not registered there yet, then registration is required).
Click on the button New analyses -> New dataset and in the appeared source selection window for dataset, click on Athena:
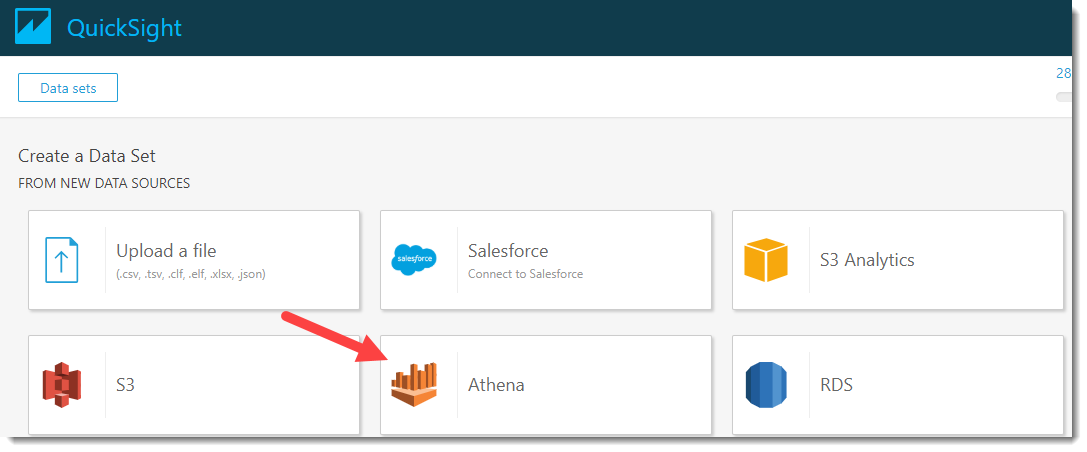
We will invent a name for our data source, for example, “PNL_analysis” and click on the “Create data source” button.
Next, the Choose your table window will open, where you need to select a database and a data source table. Choose a database - financial, and a table in it: my_traider_diary. By default, the entire table is used, but when “Use custom SQL” is selected, you can customize and fine-tune the data sample you need. For example, use the entire table and click on the Edit / Preview Data button.
A new page will open, where you can make additional settings and process the available data.
Now it is necessary to add additional calculated fields to our dataset: quarter and year of the operation. The attentive reader may notice that such manipulations were easier to do on the side of the parser before saving the Final Report to CSV. Undoubtedly, but my goal now is to demonstrate the capabilities and flexibility of setting up a BI system on the fly. Continue the creation of calculated fields by clicking on the "New field" button.
To select the year of the operation and the quarter, simple formulas are used:

When the calculated fields are successfully created and added to the sample, we give the name to our dataset, for example, “my_pnl_analyze” and click on the “Save and visualize” button.
After that, we are transferred to the Amazon QuickSight main board and the first thing we need to do is set up a filter for the report date (taking into account that the same data was collected from three sections). Select the key date 2018-10-01 and click on the Apply button and go to the Visualize tab.
Now we can visualize the result for a portfolio in any plane, for example, for each security within a trading account, and divided in turn by currency (since the result is not comparable in different currencies) and types of transactions. Let's start with the most powerful tool of any BI - pivot tables. To save space and display flexibility, I rendered currencies into a separate control (analog slice in MS Excel)
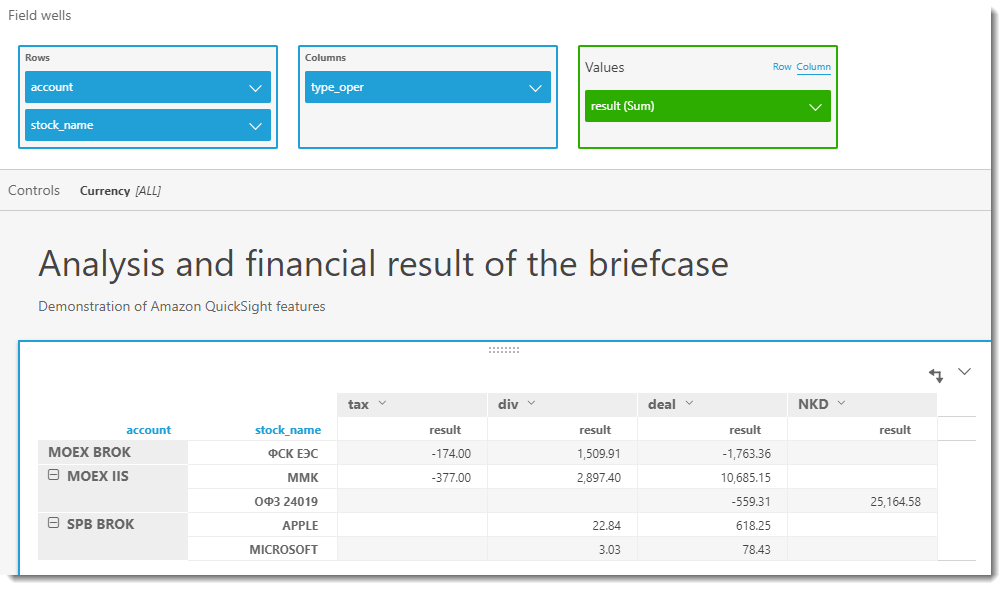
The following graph is a bar graph:
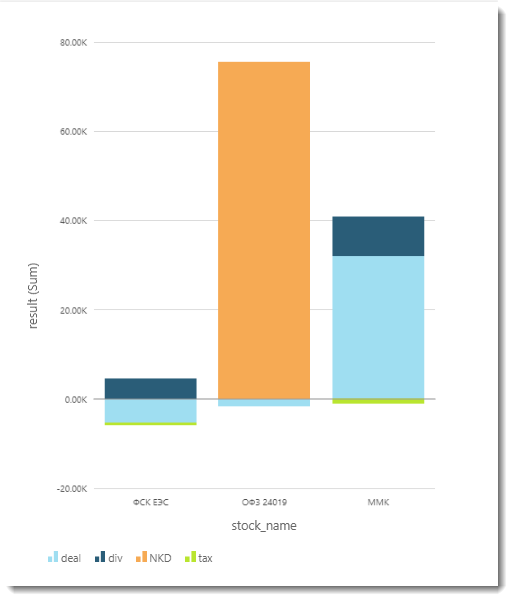
And now we will create a table that will show us how much we have invested in each paper, how many days it is in our portfolio and what is the yield for the entire period of ownership. To do this, add two new calculated fields: sum_investment and count_days.
The final table is shown in the screenshot below:
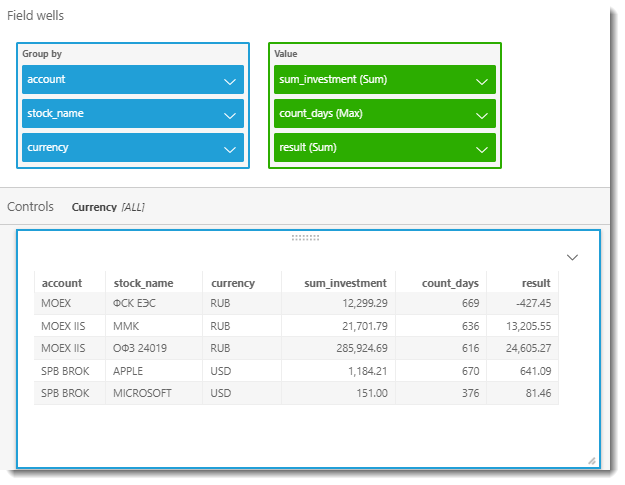
We have reviewed with you the implementation of the report parser and how to analyze the data prepared by it on the fly using Amazon services. Also touched upon some business and fundamental aspects of the analysis of the investment portfolio, because this topic is almost immense and it is quite difficult to fit it in one article, I think it makes sense to put it in a separate publication or even a cycle of publications.
As for the use of the broker's report processing tool and the approaches and algorithms involved in it, they can be used (with a corresponding modification) for processing reports of other Brokers. In any case, if you are thinking of adapting the code to your needs, I am ready to give some advice, so do not hesitate to ask questions - I will definitely try to answer them.
I am sure that this system will find its application and will have further development. For example, it is planned to add to the calculation of the full PNL for the portfolio, accounting for depository and other commissions (for example, withdrawal of funds), as well as redemption of bonds, etc. ... The calculated fields on the Quicksight side were used for the demonstration purpose, in the next version of the parser, all these additional columns will be transferred to Python and will be calculated on the side of the parser.
As an architect and main business customer of this solution, I see further modernization as follows: well, I don’t want to manually manually request these XML reports every time! Of course, there is no other possibility so far, but the Broker API with the transfer of a token and a sampling range would ideally be suitable for receiving weekly raw reports. The subsequent full automatic processing on the Amazon side: from triggering an ETL-job on AWS Glue to getting finished results in the form of graphs and tables in Amazon QuickSight will allow you to fully automate the process.
The full source code can be found in my GitHub repository.
How to determine whether the ownership of this security brought in income or only loss and disappointment?
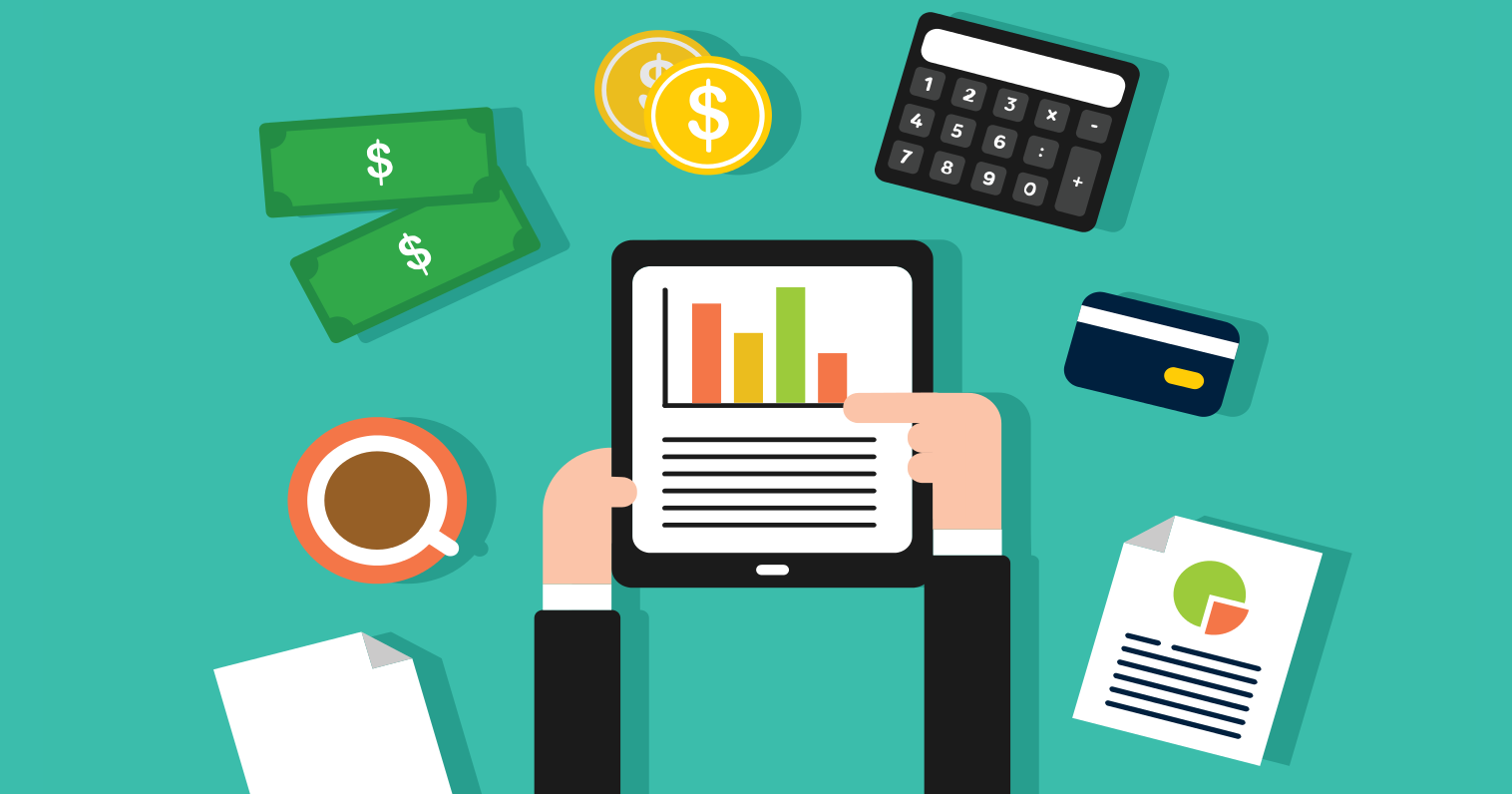
In this article I will tell you how to determine and visualize the adjusted financial result for securities.
Using the example of client reporting Opening Broker, we consider parsing and consolidating brokerage reports for the stock market, building a cloud reporting system architecture followed by a simple and convenient analysis in AWS Quicksight.
Task Description
Many trainings and training lessons tell us about the need to maintain a trading journal, which records all the parameters of the transaction for further analysis and summarizing the work of the trading strategy. I agree that such an approach to work on the Exchange allows you to discipline a trader, to increase his awareness, but it can also be great to tire out the tedious process.
I admit, at first I tried carefully to follow the advice of journaling, meticulously wrote down every transaction with its parameters in an Excel spreadsheet, built some reports, summary diagrams, planned future deals, but ... I was quickly tired of all this.
Why keeping a trader's manual journal is inconvenient?
- manual filling of the magazine (even with the use of partial automation, in the form of unloading transactions from the trading terminal for the day) quickly tires;
- there is a high risk of errors or typographical errors during manual input;
- может случиться так, что активный трейдер становится пассивным инвестором и он все реже и реже возвращается к этому журналу, а потом и вовсе забывает о нем (мой случай); ну, и наконец,
- мы умеем программировать, почему бы этим не воспользоваться и не автоматизировать весь процесс? Итак, погнали!
Often, brokerage companies are high-tech organizations that provide their clients with fairly high-quality analytics on virtually all issues of interest. It is fair to say that with each update this reporting gets better and better, but even the most advanced of them may not have the customization and consolidation that demanding and inquisitive customers want to see.
For example, Opening Broker allows you to receive brokerage reports in XML format in your account, but if you have an IIS and a normal brokerage account on the Moscow Stock Exchange (MOEX), these will be two different reports, and if you still have an account on St. Petersburg Petersburg Stock Exchange (SPB), then one more will be added to the first two.
So, to get a consolidated investor log, you will need to process three files in XML format.
The above reports on MOEX and SPB are slightly different in their formats, which will need to be taken into account in the implementation of the data mapping.
The architecture of the developed system
The diagram below shows the architecture model of the system being developed:
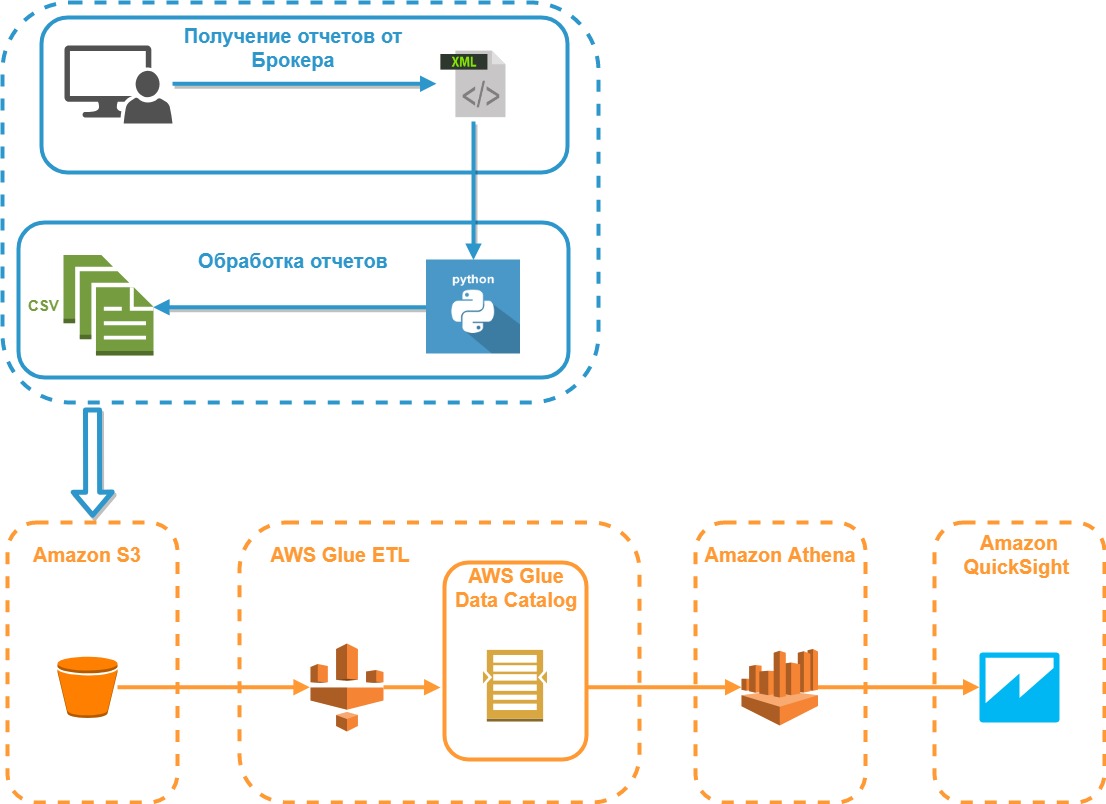
Parser implementation
We will receive reports on all three accounts in the My Account for the maximum possible period (can be divided into several reports for each year), save them in XML format and put them in one folder. As a test data for the study we will use the fictional client portfolio, but with the most approximate parameters to market realities.

Suppose that the investor Mr. X we are considering has a small portfolio of five securities:
- The report on the stock exchange SPB will be two papers: Apple and Microsoft;
- In the report on the stock exchange MOEX (brokerage) one paper: FGC UES;
- The report on the stock exchange MOEX (IIS) two papers: CMI and OFZ 24019;
According to our five papers, there may be buy / sell transactions, dividends and coupon payments, prices may change, etc. We want to see the current situation, namely: the financial result, taking into account all payments, transactions and current market value.
And here comes Python, we read information from all reports into one array:
my_files_list = [join('Data/', f) for f in listdir('Data/') if isfile(join('Data/', f))]
my_xml_data = []
# Считывание отчетов из каталогаfor f in my_files_list:
tree = ET.parse(f)
root = tree.getroot()
my_xml_data.append(root)
For analytics, from reports we need several entities, namely:In order to prepare the sample, we will use four dictionaries to describe the above sets.
- Positions of securities in the portfolio;
- Concluded transactions;
- Non-trading operations and other movements on the account;
- Average prices of open positions
dict_stocks = {'stock_name': [], 'account': [], 'currency': [], 'current_cost': [], 'current_cost_rub': [], 'saldo' : []}
dict_deals = {'stock_name': [], 'account': [], 'date_oper': [], 'type_oper': [], 'quantity': [], 'price': [], 'currency': [], 'brokerage': [], 'result': []}
dict_flows = {'stock_name': [], 'account': [], 'date_oper': [], 'type_oper': [], 'result': [], 'currency': []}
dict_avg_price = {'stock_name': [], 'account': [], 'avg_open_price' : []}
A few words about what these dictionaries are.
Dictionary dict_stocks
Словарь dict_stocks необходим для хранения общей информации по портфелю:
- Наименование бумаги (stock_name);
- Наименование счета (SPB, MOEX BROK, MOEX IIS) (account);
- Валюта, используемая для расчетов по данной бумаге (currency);
- Текущая стоимость (на момент формирования отчета в Личном кабинете Открытие Брокер) (current_cost). Здесь хочу заметить, что для сверхтребовательных клиентов, можно в будущем внести дополнительную доработку и использовать динамическое получение котировки ценной бумаги из торгового терминала или с сайта соответствующей биржи;
- Текущая стоимость позиции ценной бумаги на момент формирования отчета (current_cost_rub)
Аналогично вышеупомянутому пункту, здесь можно так же получать курс ЦБ на текущий момент или биржевой курс, кому как нравится. - Текущий остаток ценных бумаг (saldo)
Dict_deals dictionary
Словарь dict_deals необходим для хранения следующей информации по совершенным сделкам:
- Наименование бумаги (stock_name);
- Наименование счета (SPB, MOEX BROK, MOEX IIS) (account);
- Дата совершения сделки, т.е. Т0 (date_oper);
- Тип операции (type_oper);
- Объем бумаг, участвующих в сделке (quantity);
- Цена, по которой была исполнена сделка (price);
- Валюта, в которой была совершена операция (currency);
- Брокерская комиссия за сделку (brokerage);
- Финансовый результат по сделке (result)
Dict_flows dictionary
Словарь dict_flows отражает движение средств по клиентскому счету и используется для хранения следующей информации:
- Наименование бумаги (stock_name);
- Наименование счета (SPB, MOEX BROK, MOEX IIS) (account);
- Дата совершения сделки, т.е. Т0 (date_oper);
- Тип операции (type_oper). Может принимать несколько значений: div, NKD, tax;
- Валюта, в которой была совершена операция (currency);
- Финансовый результат операции (result)
Dictionary dict_avg_price
Словарь dict_avg_price необходим для учета информации по средней цене покупки по каждой бумаге:
- Наименование бумаги (stock_name);
- Наименование счета (SPB, MOEX BROK, MOEX IIS) (account);
- Средняя цена открытой позиции (avg_open_price)
We process an array of XML documents and fill these dictionaries with the appropriate data:
# Сбор данных из соответствующих частей отчетовfor XMLdata in my_xml_data:
# Информация о Бирже и счете
exchange_name = 'SPB'if XMLdata.get('board_list') == 'ФБ СПБ'else'MOEX'
client_code = XMLdata.get('client_code')
account_name = get_account_name(exchange_name, client_code)
# Маппинг тегов
current_position, deals, flows, stock_name, \
saldo, ticketdate, price, brokerage, \
operationdate, currency, \
current_cost, current_cost_rub, \
stock_name_deal, payment_currency, currency_flows = get_allias(exchange_name)
# Информация о состоянии клиентского портфеля
get_briefcase(XMLdata)
df_stocks = pd.DataFrame(dict_stocks)
df_stocks.set_index("stock_name", drop = False, inplace = True)
# Информация о сделках
get_deals(XMLdata)
df_deals = pd.DataFrame(dict_deals)
df_avg = pd.DataFrame(dict_avg_price)
# Информация о неторговых операциях по счету
get_nontrade_operation(XMLdata)
df_flows = pd.DataFrame(dict_flows)
All processing is looped through all the XML data from the reports. Information about the trading platform, client code is the same in all reports, so you can safely extract it from the same tags without using mapping.
But then you have to apply a special construction that will provide the necessary alias for the tag based on the report (SPB or MOEX), since the same data in these reports are called differently.
Discrepancies in tags
- Комиссия брокера по сделке в отчете SBP лежит в теге brokerage, а в отчете MOEX — broker_commission;
- Дата неторговой операции по счету в отчете SPB – это operationdate, а в MOEX — operation_date и т.д.
Sample Tag Mapping
tags_mapping = {
'SPB': {
'current_position': 'briefcase_position',
'deals': 'closed_deal',
'flows': 'nontrade_money_operation',
...
'stock_name_deal': 'issuername',
'paymentcurrency': 'paymentcurrency',
'currency_flows': 'currencycode'
},
'MOEX': {
'current_position': 'spot_assets',
'deals': 'spot_main_deals_conclusion',
'flows': 'spot_non_trade_money_operations',
...
'stock_name_deal': 'security_name',
'paymentcurrency': 'price_currency_code',
'currency_flows': 'currency_code'
}
}
The get_allias function returns the name of the required tag for processing, taking as input the name of the trading platform:
Get_allias function
defget_allias(exchange_name):return(
tags_mapping[exchange_name]['current_position'],
tags_mapping[exchange_name]['deals'],
tags_mapping[exchange_name]['flows'],
...
tags_mapping[exchange_name]['stock_name_deal'],
tags_mapping[exchange_name]['paymentcurrency'],
tags_mapping[exchange_name]['currency_flows']
)
The function get_briefcase is responsible for processing information about the state of the client portfolio:
Get_briefcase function
defget_briefcase(XMLdata):# В отчете ФБ СПБ портфель находится под тегом briefcase_position
briefcase_position = XMLdata.find(current_position)
ifnot briefcase_position:
returntry:
for child in briefcase_position:
stock_name_reduce = child.get(stock_name).upper()
stock_name_reduce = re.sub('[,\.]|(\s?INC)|(\s+$)|([-\s]?АО)', '', stock_name_reduce)
dict_stocks['stock_name'].append(stock_name_reduce)
dict_stocks['account'].append(account_name)
dict_stocks['currency'].append(child.get(currency))
dict_stocks['current_cost'].append(float(child.get(current_cost)))
dict_stocks['current_cost_rub'].append(float(child.get(current_cost_rub)))
dict_stocks['saldo'].append(float(child.get(saldo)))
except Exception as e:
print('get_briefcase --> Oops! It seems we have a BUG!', e)
Further, using the function get_deals, information about transactions is extracted:
Get_deals function
defget_deals(XMLdata):
stock_name_proc = ''
closed_deal = XMLdata.find(deals)
ifnot closed_deal:
return# Отчет по SPB имеет иную сортировку - только по дате сделки,# тогда как отчеты MOEX: по бумаге, а потом по дате сделки# Отсортируем сделки по бумаге:if exchange_name == 'SPB':
sortchildrenby(closed_deal, stock_name_deal)
for child in closed_deal:
sortchildrenby(child, stock_name_deal)
try:
for child in closed_deal:
stock_name_reduce = child.get(stock_name_deal).upper()
stock_name_reduce = re.sub('[,\.]|(\s?INC)|(\s+$)|([-\s]?АО)', '', stock_name_reduce)
dict_deals['stock_name'].append(stock_name_reduce)
dict_deals['account'].append(account_name)
dict_deals['date_oper'].append(to_dt(child.get(ticketdate)).strftime('%Y-%m-%d'))
current_cost = get_current_cost(stock_name_reduce)
# В отчете по SPB один тег на количество - quantity,# а на MOEX целых два: buy_qnty и sell_qntyif exchange_name == 'MOEX':
if child.get('buy_qnty'):
quantity = float(child.get('buy_qnty'))
else:
quantity = - float(child.get('sell_qnty'))
else:
quantity = float(child.get('quantity'))
dict_deals['quantity'].append(quantity)
dict_deals['price'].append(float(child.get('price')))
dict_deals['type_oper'].append('deal')
dict_deals['currency'].append(child.get(payment_currency))
brok_comm = child.get(brokerage)
if brok_comm isNone:
brok_comm = 0else:
brok_comm = float(brok_comm)
dict_deals['brokerage'].append(float(brok_comm))
# Доходность по каждой сделке и средняя цена позицииif stock_name_proc != stock_name_reduce:
if stock_name_proc != '':
put_avr_price_in_df(account_name, stock_name_proc, \
pnl.m_net_position, pnl.m_avg_open_price)
current_cost = get_current_cost(stock_name_proc)
pnl.update_by_marketdata(current_cost)
if len(dict_deals['result']) > 0:
if exchange_name != 'SPB':
dict_deals['result'][-1] = pnl.m_unrealized_pnl * 0.87 -dict_deals['brokerage'][-2]
else:
dict_deals['result'][-1] = pnl.m_unrealized_pnl - dict_deals['brokerage'][-2]
stock_name_proc = stock_name_reduce
pnl = PnlSnapshot(stock_name_proc, float(child.get('price')), quantity)
dict_deals['result'].append(-1 * brok_comm)
else:
pnl.update_by_tradefeed(float(child.get('price')), quantity)
# Продажа бумаг, фиксация результатаif quantity < 0:
if pnl.m_realized_pnl > 0and exchange_name != 'SPB':
pnl_sum = pnl.m_realized_pnl * 0.87 - brok_comm
else:
pnl_sum = pnl.m_realized_pnl - brok_comm
dict_deals['result'].append(float(pnl_sum))
else:
pnl.update_by_marketdata(current_cost)
dict_deals['result'].append(-1 * brok_comm)
put_avr_price_in_df(account_name, stock_name_proc, \
pnl.m_net_position, pnl.m_avg_open_price)
current_cost = get_current_cost(stock_name_proc)
pnl.update_by_marketdata(current_cost)
if len(dict_deals['result']) > 0:
if exchange_name != 'SPB':
dict_deals['result'][-1] = pnl.m_unrealized_pnl * 0.87 -dict_deals['brokerage'][-2]
else:
dict_deals['result'][-1] = pnl.m_unrealized_pnl - dict_deals['brokerage'][-2]
except Exception as e:
print('get_deals --> Oops! It seems we have a BUG!', e)
In addition to processing an array with information about the parameters of the transaction, it also calculates the average price of an open position and the PNL realized by the FIFO method. The PnlSnapshot class is responsible for this calculation, for creating which, with minor modifications, the code presented here was taken as the basis: P & L calculation
And, finally, the most difficult to implement is the function of obtaining information about non-trading operations - get_nontrade_operation . Its complexity lies in the fact that in the report block used for non-trading operations, there is no clear information about the type of operation and the security to which this operation is linked.
Example of payment purposes for non-trading operations
Выплата дивидендов или накопленного купонного дохода может быть указана так:
- Выплата дохода клиент <777777> дивиденды <APPLE INC-ао> --> выплата дивидендов из отчета SPB;
- Выплата дохода клиент <777777> дивиденды <MICROSOFT COM-ао>
- Выплата дохода клиент 777777i (НКД 2 ОФЗ 24019) налог к удержанию 0.00 рублей --> выплата купона из отчета MOEX;
- Выплата дохода клиент 777777 дивиденды ФСК ЕЭС-ао налог к удержанию XX.XX рублей --> выплата дивидендов из отчета MOEX. и т.д.
Accordingly, it will be difficult to manage without regular expressions, therefore we will use them to the full. The other side of the question is that the name of the company does not always coincide with the name in the portfolio or in the transactions. Therefore, the received name of the issuer from the purpose of the payment must be additionally correlated with the dictionary. As a dictionary, we will use an array of transactions, since There is the most comprehensive list of companies.
The function get_company_from_str extracts the name of the issuer from the comment:
Get_company_from_str function
defget_company_from_str(comment):
company_name = ''# Шаблоны для случаев дивиденды/купон
flows_pattern = [
'^.+дивиденды\s<(\w+)?.+-ао>$',
'^.+дивиденды\s(.+)-а.+$',
'^.+\(НКД\s\d?\s(.+)\).+$',
'^.+дивидендам\s(.+)-.+$'
]
for pattern in flows_pattern:
match = re.search(pattern, comment)
if match:
return match.group(1).upper()
return company_name
The function get_company_from_briefcase brings the name of the company to the dictionary, if it finds a match among the companies that participated in the transactions:
Get_company_from_briefcase function
defget_company_from_briefcase(company_name):
company_name_full = None
value_from_dic = df_deals[df_deals['stock_name'].str.contains(company_name)]
company_arr = value_from_dic['stock_name'].unique()
if len(company_arr) == 1:
company_name_full = company_arr[0]
return company_name_full
And finally, the final data collection function for non-trading operations is get_nontrade_operation :
Get_nontrade_operation function
defget_nontrade_operation(XMLdata):
nontrade_money_operation = XMLdata.find(flows)
ifnot nontrade_money_operation:
returntry:
for child in nontrade_money_operation:
comment = child.get('comment')
type_oper_match = re.search('дивиденды|НКД|^.+налог.+дивидендам.+$', comment)
if type_oper_match:
company_name = get_company_from_str(comment)
type_oper = get_type_oper(comment)
dict_flows['stock_name'].append(company_name)
dict_flows['account'].append(account_name)
dict_flows['date_oper'].append(to_dt(child.get(operationdate)).strftime('%Y-%m-%d'))
dict_flows['type_oper'].append(type_oper)
dict_flows['result'].append(float(child.get('amount')))
dict_flows['currency'].append(child.get(currency_flows))
except Exception as e:
print('get_nontrade_operation --> Oops! It seems we have a BUG!', e)
The result of the collection of data from the reports will be three DataFrame, which are approximately the following:
- DataFrame with information on average prices of open positions:
- Transaction Information DataFrame:
- DataFrame with information about non-trading operations:
So, all we have to do is perform the external merging of the table of transactions with the table of information about the portfolio:
df_result = pd.merge(df_deals, df_stocks_avg, how='outer', on=['stock_name', 'account', 'currency']).fillna(0)
df_result.sample(10)
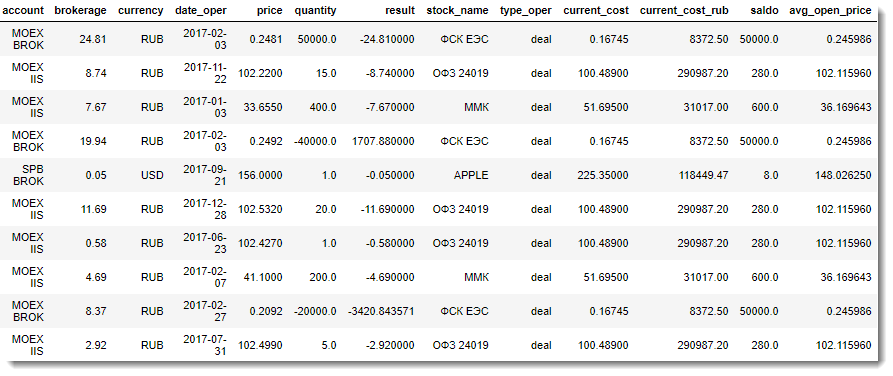
And, finally, the final part of the data array processing is the merging of the data array obtained at the previous step with the DataFrame for non-trade transactions.
The result of the work done is one large flat table with all the necessary information for the analysis:
df_result_full = df_result.append(df_flows, ignore_index=True).fillna(0)
df_result_full.sample(10).head()
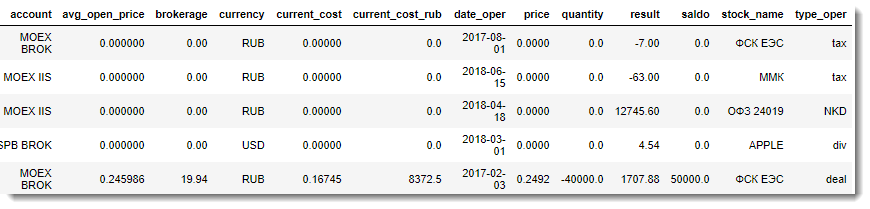
The resulting data set (Final Report) from the DataFrame is easily downloaded to CSV and can then be used for detailed analysis in any BI system.
ifnot exists('OUTPUT'): makedirs('OUTPUT')
report_name = 'OUTPUT\my_trader_diary.csv'
df_result_full.to_csv(report_name, index = False, encoding='utf-8-sig')
Loading and processing data in AWS
Progress does not stand still, and now cloud services and serverless computing models are gaining great popularity in processing and storing data. This is largely due to the simplicity and low cost of such an approach, when building an architecture for complex calculations or processing large data does not require buying expensive equipment, and you only rent power for the cloud you need and deploy the necessary resources quickly for a relatively small fee. .
One of the largest and most well-known cloud technology vendors on the market is Amazon. Consider the example of the environment Amazon Web Services (AWS) building an analytical system for processing data on our investment portfolio.
AWS has an extensive selection of tools, but we will use the following:
- Amazon S3 - object storage, which allows you to store virtually unlimited amounts of information;
- AWS Glue is the most powerful ETL cloud service that can itself determine the structure and generate the ETL code using the given source data;
- Amazon Athena is a serverless online SQL query service that allows you to quickly analyze data from S3 without much preparation. He also has access to the metadata that AWS Glue prepares, which makes it possible to access the data immediately after passing the ETL;
- Amazon QuickSight is a serverless BI service, you can build any visualization, analytical reports on the fly, etc.
With Amazon's documentation, everything is in order, in particular, there is a good article Best Practices When Using Athens with AWS Glue , which describes how to create and use tables and data using AWS Glue. Let us and we will use the main ideas of this article and apply them to create our own architecture of the analytical reporting system.
CSV files prepared by our report parser will be added to the S3 bucket. It is planned that the corresponding folder on S3 will be replenished every Saturday - at the end of the trading week, so we can not do without data partitioning by the date of the formation and processing of the report.
In addition to optimizing the work of SQL queries to such data, this approach will allow us to conduct additional analysis, for example, to obtain the dynamics of changes in the financial result for each paper, etc.
Work with Amazon S3
- Создадим бакет на S3, назовем его «report-parser»;
- В этом бакете «report-parser» создадим папку под названием «my_trader_diary»;
- В каталоге «my_trader_diary» создадим каталог с датой текущего отчета, например, «date_report=2018-10-01» и поместим в него CSV-файл;
- Только ради эксперимента и лучшего понимания секционирования создадим еще два каталога: «date_report=2018-09-27» и «date_report=2018-10-08». В них положим тот же CSV-файл;
- Итоговый S3 бакет «report-parser» должен иметь вид как показано на картинки ниже:
Work with AWS Glue
По большому счету, можно обойтись лишь Amazon Athena чтобы создать внешнюю таблицу из данных, лежащих на S3, но AWS Glue – более гибкий и удобный для этого инструмент.
- Заходим в AWS Glue и создаем новый Crawler, который будет из разрозненных по отчетным датам CSV-файлов собирать одну таблицу:
- Задаем имя нового Crawler;
- Указываем хранилище, откуда брать данные (s3://report-parser/my_trader_diary/)
- Выбираем или создаем новую IAM роль, которая будет иметь доступ к запуску Crawler и доступ к указанному ресурсу на S3;
- Далее, необходимо задать частоту запуска. Пока ставим по требованию, но в дальнейшем, думаю, это изменится и запуск станет еженедельным;
- Сохраняем и ждем, когда Crawler создастся.
- Когда Crawler перейдет в состояние Ready, запускаем его!
- Как только он отработает, в закладке AWS Glue: Database -> Tables появится новая таблица my_trader_diary:
Consider the generated table in more detail.
If you click on the name of the created table, we will go to the page with the description of the metadata. At the bottom there is a table diagram and the most recent is a column that was not in the original CSV file - date_report. Glue creates this AWS column automatically based on the definition of the source data sections (in the S3 batch, we specifically named folders - date_report = YYYY-MM-DD, which allowed them to be used as sections, separated by date).
Table partitioning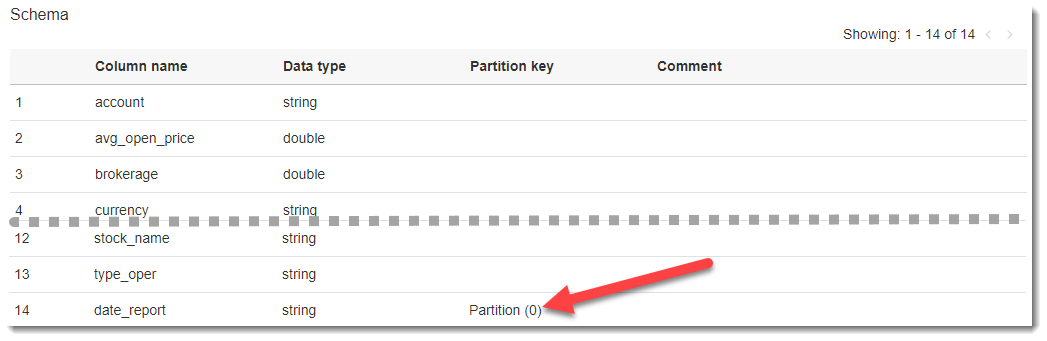
На той же странице в верхнем правом углу есть кнопка View partitions, нажав на которую, мы можем увидеть из каких секций состоит наша сформированная таблица:
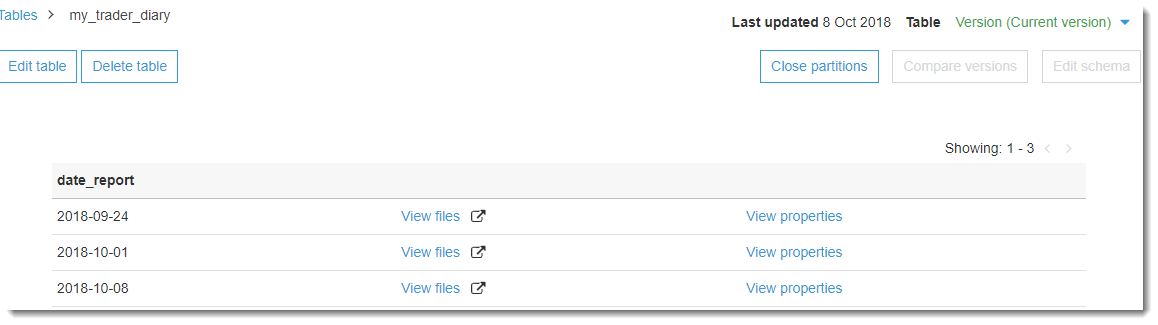
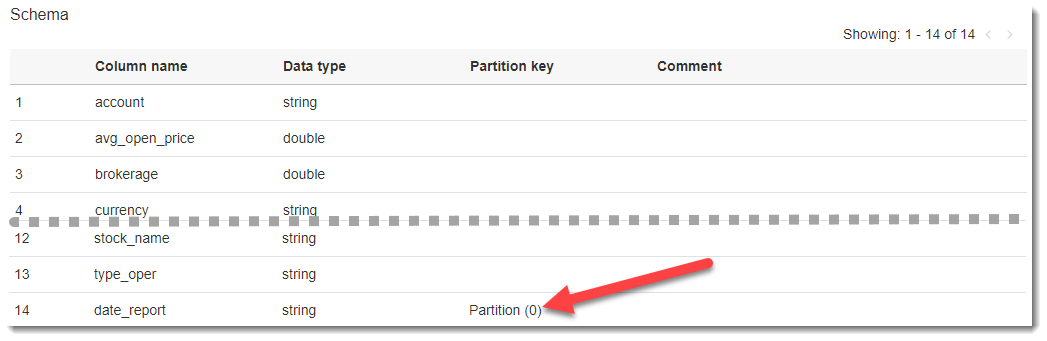
На той же странице в верхнем правом углу есть кнопка View partitions, нажав на которую, мы можем увидеть из каких секций состоит наша сформированная таблица:
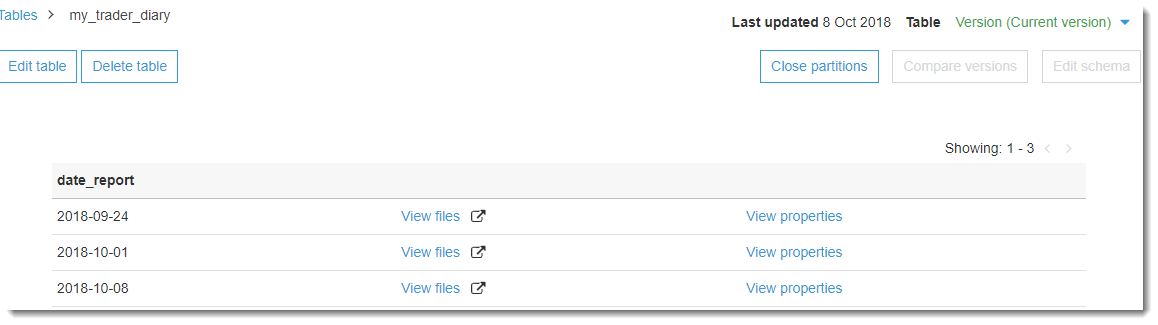
Data analysis
Having at our disposal downloaded processed data, we can easily proceed to their analysis. First, let's look at the features of Amazon Athena as the easiest and fastest way to perform analytical queries. To do this, go to the Amazon Athena service, select the database we need (financial) and write the following SQL code:
select
d.date_report, d.account,
d.stock_name, d.currency,
sum(d.quantity) as quantity,
round(sum(d.result), 2) asresultfrom my_trader_diary d
groupby
d.date_report, d.account,
d.stock_name, d.currency
orderby
d.account, d.stock_name,
d.date_report;
This query will give us a net financial result for each paper for all reporting dates. Because we have downloaded the same report three times for different dates, and the result will not change, which, of course, in the real market conditions will be different:
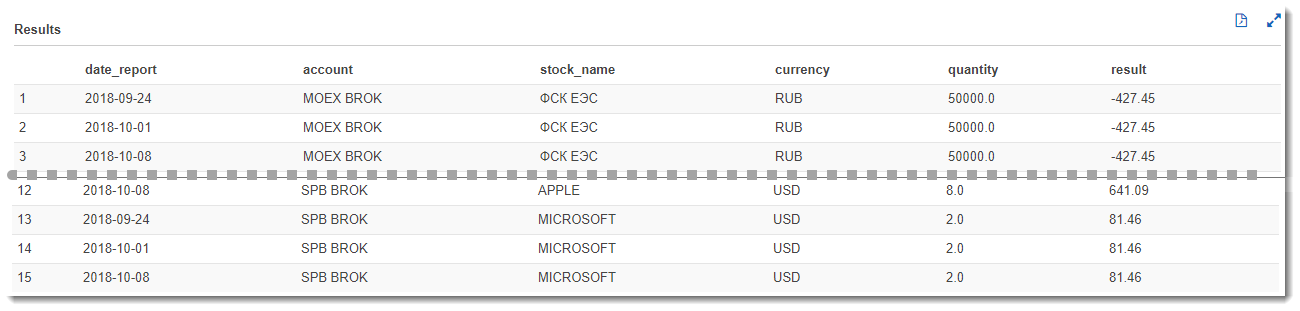
But what if we want to visualize the data in the form of flexible tables or charts? This is where Amazon QuickSight comes to the rescue, with which you can set up flexible analytics almost as quickly as writing a SQL query. Let's go to Amazon QuickSight (if you have not registered there yet, then registration is required).
Click on the button New analyses -> New dataset and in the appeared source selection window for dataset, click on Athena:
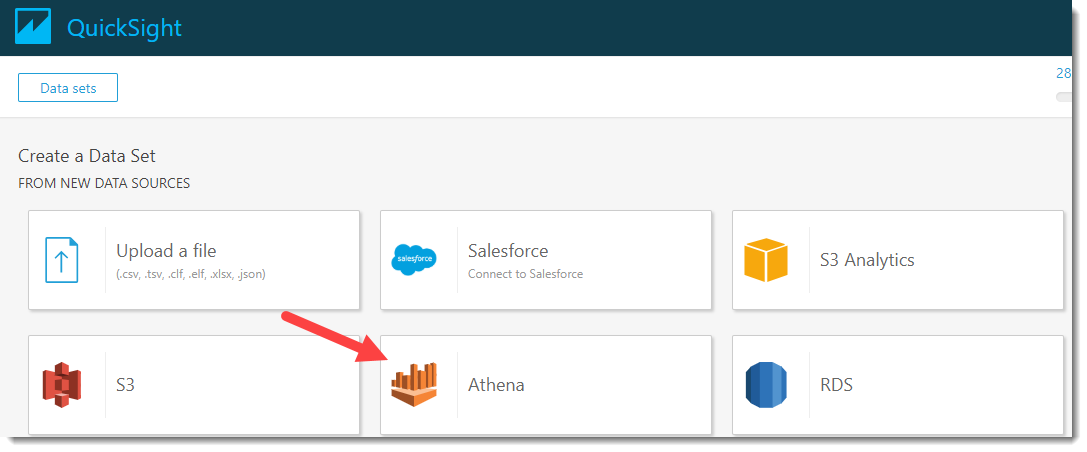
We will invent a name for our data source, for example, “PNL_analysis” and click on the “Create data source” button.
Next, the Choose your table window will open, where you need to select a database and a data source table. Choose a database - financial, and a table in it: my_traider_diary. By default, the entire table is used, but when “Use custom SQL” is selected, you can customize and fine-tune the data sample you need. For example, use the entire table and click on the Edit / Preview Data button.
A new page will open, where you can make additional settings and process the available data.
Now it is necessary to add additional calculated fields to our dataset: quarter and year of the operation. The attentive reader may notice that such manipulations were easier to do on the side of the parser before saving the Final Report to CSV. Undoubtedly, but my goal now is to demonstrate the capabilities and flexibility of setting up a BI system on the fly. Continue the creation of calculated fields by clicking on the "New field" button.
Creating a new field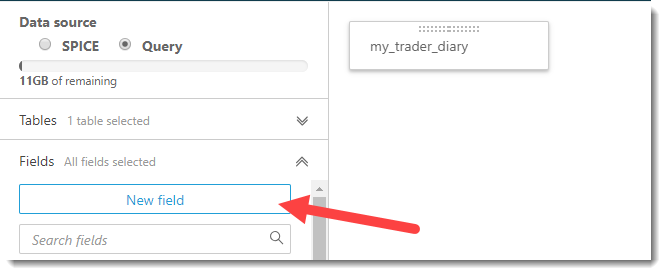
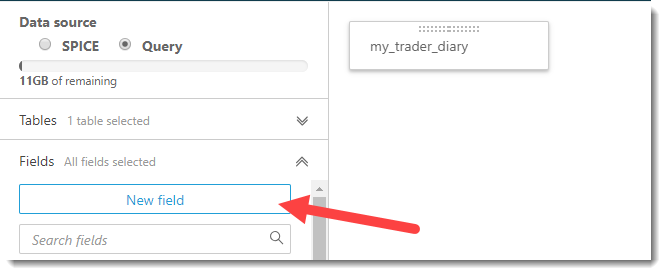
To select the year of the operation and the quarter, simple formulas are used:

Filling formulas for the new field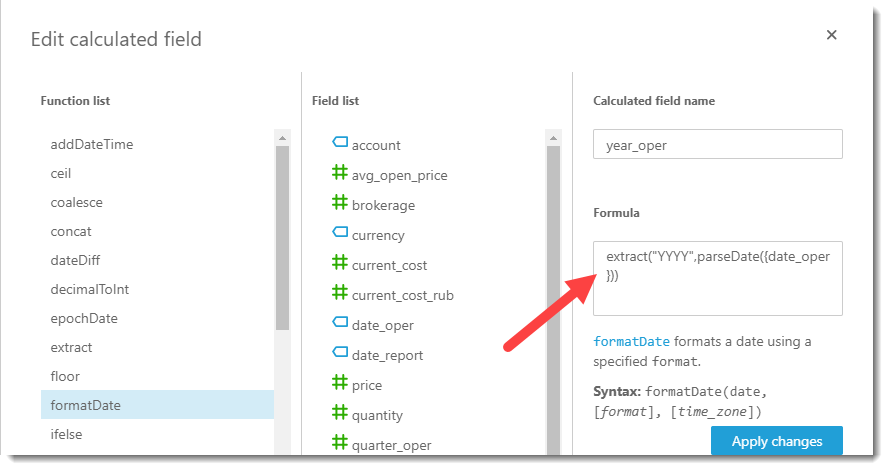
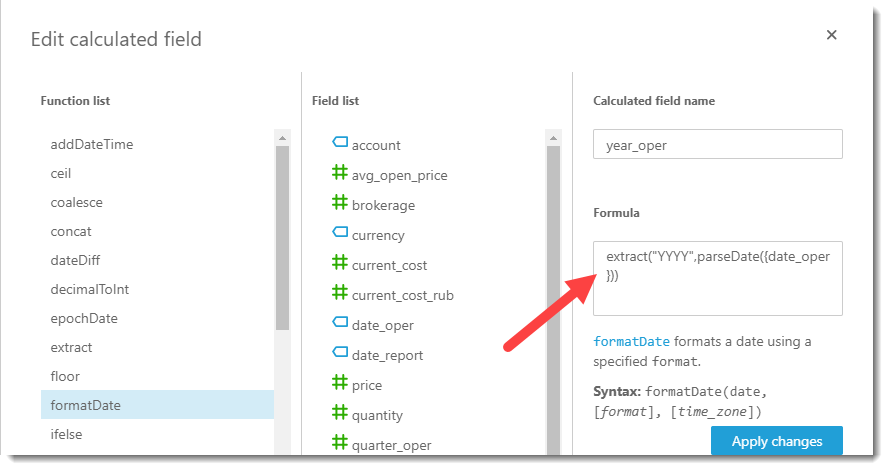
When the calculated fields are successfully created and added to the sample, we give the name to our dataset, for example, “my_pnl_analyze” and click on the “Save and visualize” button.
After that, we are transferred to the Amazon QuickSight main board and the first thing we need to do is set up a filter for the report date (taking into account that the same data was collected from three sections). Select the key date 2018-10-01 and click on the Apply button and go to the Visualize tab.
Filter installation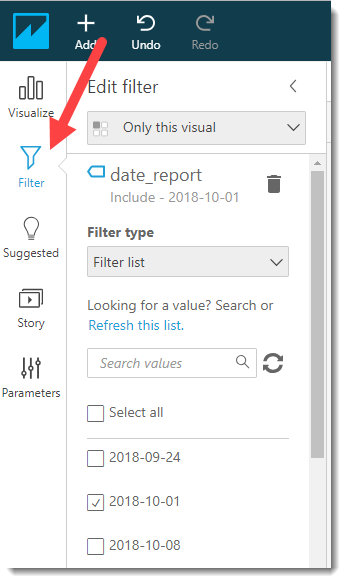
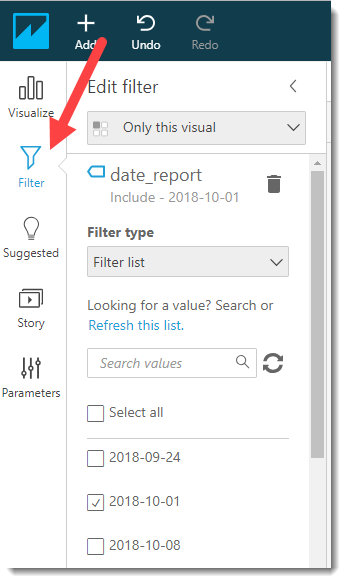
Now we can visualize the result for a portfolio in any plane, for example, for each security within a trading account, and divided in turn by currency (since the result is not comparable in different currencies) and types of transactions. Let's start with the most powerful tool of any BI - pivot tables. To save space and display flexibility, I rendered currencies into a separate control (analog slice in MS Excel)
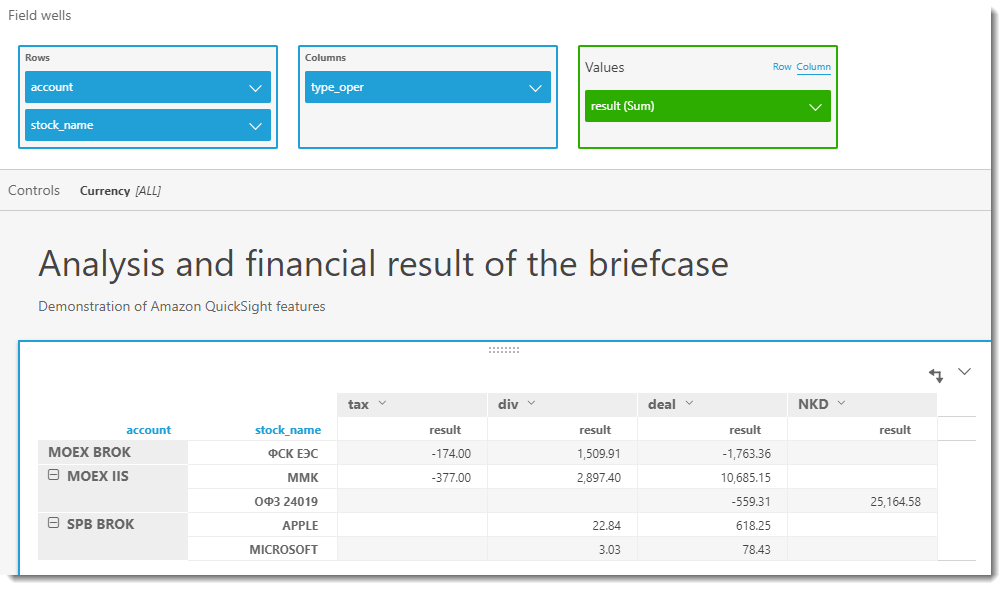
The table above shows that if an investor decides to sell all shares of FGC UES now, he will thereby fix the loss, since dividends paid in the amount of 1 509.91 p. do not cover its costs (1 763.36 p. - negative exchange difference and 174 p. - NDFL for dividends). It makes sense to wait and wait for the best times on the Exchange.
The following graph is a bar graph:
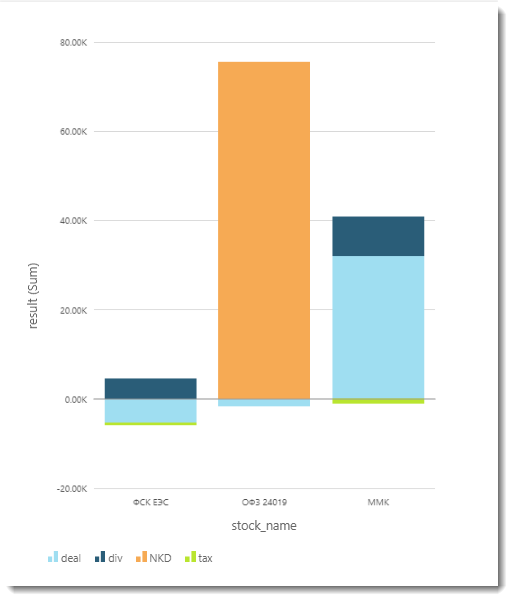
And now we will create a table that will show us how much we have invested in each paper, how many days it is in our portfolio and what is the yield for the entire period of ownership. To do this, add two new calculated fields: sum_investment and count_days.
Field sum_investment
Вычисляемое поле sum_investment (сумма инвестиций) будем определять так:
ifelse({stock_name} = 'ОФЗ 24019',{avg_open_price} * quantity * 10,{avg_open_price} * quantity)
Такой подход к обработке расчета суммы вложений по облигациям обусловлен тем, что по ним всегда указывается цена – как процент от номинала (номинал в данном случае – 1000р).
ifelse({stock_name} = 'ОФЗ 24019',{avg_open_price} * quantity * 10,{avg_open_price} * quantity)
Такой подход к обработке расчета суммы вложений по облигациям обусловлен тем, что по ним всегда указывается цена – как процент от номинала (номинал в данном случае – 1000р).
Count_days field
Вычисляемое поле count_day (количество дней владения бумагой) мы определим как разницу между датой операции и отчетной датой и в сводной таблице возьмем максимум:
dateDiff(parseDate({date_oper}),parseDate({date_report}))
dateDiff(parseDate({date_oper}),parseDate({date_report}))
The final table is shown in the screenshot below:
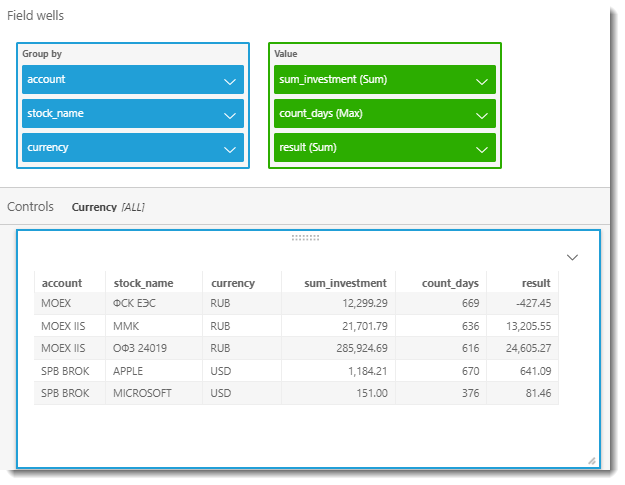
Conclusions and summary
We have reviewed with you the implementation of the report parser and how to analyze the data prepared by it on the fly using Amazon services. Also touched upon some business and fundamental aspects of the analysis of the investment portfolio, because this topic is almost immense and it is quite difficult to fit it in one article, I think it makes sense to put it in a separate publication or even a cycle of publications.
As for the use of the broker's report processing tool and the approaches and algorithms involved in it, they can be used (with a corresponding modification) for processing reports of other Brokers. In any case, if you are thinking of adapting the code to your needs, I am ready to give some advice, so do not hesitate to ask questions - I will definitely try to answer them.
I am sure that this system will find its application and will have further development. For example, it is planned to add to the calculation of the full PNL for the portfolio, accounting for depository and other commissions (for example, withdrawal of funds), as well as redemption of bonds, etc. ... The calculated fields on the Quicksight side were used for the demonstration purpose, in the next version of the parser, all these additional columns will be transferred to Python and will be calculated on the side of the parser.
As an architect and main business customer of this solution, I see further modernization as follows: well, I don’t want to manually manually request these XML reports every time! Of course, there is no other possibility so far, but the Broker API with the transfer of a token and a sampling range would ideally be suitable for receiving weekly raw reports. The subsequent full automatic processing on the Amazon side: from triggering an ETL-job on AWS Glue to getting finished results in the form of graphs and tables in Amazon QuickSight will allow you to fully automate the process.
The full source code can be found in my GitHub repository.